Understanding DLP Networks: Strategies for Data Protection
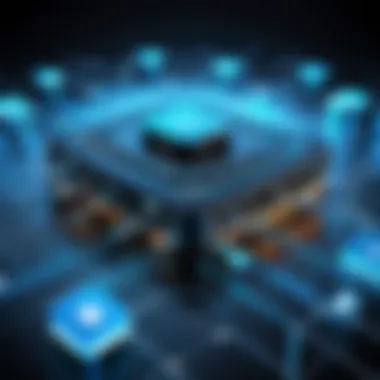
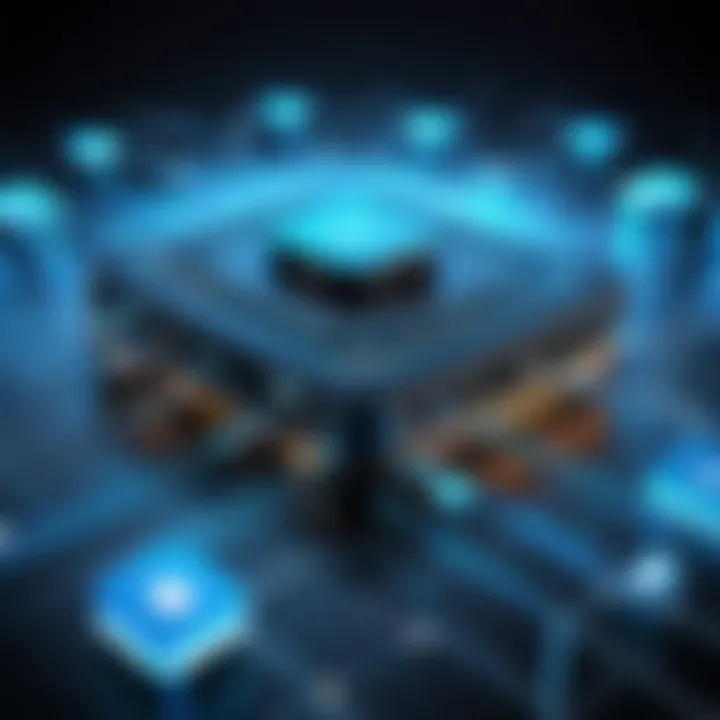
Prolusion to Cybersecurity and Network Security Convergence
In today's hyper-connected world, the significance of cybersecurity has reached unprecedented levels. Organizations are increasingly becoming targets of sophisticated attacks, which exploit vulnerabilities in both human behavior and technological systems. This creates a pressing need for robust cybersecurity measures that encompass not just data, but every aspect of the digital environment. This convergence of networking and security approaches is essential for effective data loss prevention (DLP).
The evolution of network security has been rapid. Early systems relied on simple firewalls and antivirus software, which are no longer sufficient. The integration of cybersecurity practices into network management is now common. This convergence allows for more comprehensive strategies aimed at protecting sensitive data from breaches or unauthorized access.
Securing People, Devices, and Data
Securing every facet of digital interaction is vital. Organizations must implement robust security measures that protect not just data but the people and devices interacting with it. A layered security approach is advisable.
- Training staff to recognize security threats is a foundational strategy. Phishing attacks often exploit human error. Regular training updates can mitigate these risks.
- Endpoint protection is critical. Devices such as laptops, smartphones, and tablets are common entry points for attackers. Solutions like Malwarebytes and Bitdefender are integral to safeguarding these endpoints.
- Data encryption should always be considered for storing and transmitting sensitive information. This prevents unauthorized users from accessing valuable data, even if they manage to breach network defenses.
Latest Trends in Security Technologies
Emerging technologies play a substantial role in enhancing cybersecurity efforts. Integration of artificial intelligence has made it possible to automatically detect and react to threats in real time.
- Artificial Intelligence: AI tools are employed for identifying anomalies that could indicate a cyber threat. AI can analyze vast datasets much quicker than human analysts.
- Internet of Things (IoT): Securing connected devices presents unique challenges. Each device poses a potential vulnerability. Security protocols need to adapt to protect this expanding surface area.
- Cloud security: As more organizations migrate to cloud solutions, a shift in focus towards securing cloud infrastructures is critical. The responsibility is shared between service providers and users, making it essential that both parties adopt security best practices.
Data Breaches and Risk Management
Understanding recent data breaches highlights the critical nature of effective risk management strategies. Case studies of encounters with leading firms like Equifax and Target illustrate the devastating consequences of inadequate security measures.
- These events underscore the importance of regular security audits and assessments to identify vulnerabilities.
- Establishing an incident response plan can significantly lessen the impact of a breach when it occurs. Prepare for potential threats rather than hoping they do not happen.
Future of Cybersecurity and Digital Security Technology
The future of the cybersecurity landscape is geared towards continued innovation and the increasing intersection of technology and security.
- Anticipate more integrations of AI in filtering through data to foresee and neutralize threats. Innovations in predictive analytics will likely help organizations understand their security posture before incidents arise.
- Advancements in quantum cryptography may redefine how data is encrypted and protected, making conventional methods obsolete.
Overall, cybersecurity will increasingly rely on collaborative efforts across disciplines and sectors. Organizations must keep innovating and adapting to stay one step ahead of potential threats.
Foreword to DLP Networks
Data Loss Prevention (DLP) networks play a critical role in the cybersecurity framework of any organization. With the increasing volume of data processed daily and the variety of threats present, a robust understanding and implementation of DLP is paramount to safeguarding sensitive information. This section will explore the foundational concepts of DLP networks, establishing their necessity in today’s digital landscape.
Definition of Data Loss Prevention
Data Loss Prevention refers to a set of strategies and tools designed to prevent the unauthorized access, use, or transmission of sensitive information. It encompasses a range of technologies that monitor and control endpoint activities, data transmissions, and overall network behavior. Essentially, DLP aims to detect and respond to potential data breaches before they escalate into more serious incidents.
DLP is not merely about blocking data transfers; it involves identifying sensitive data, categorizing it, and understanding how such information circulates within an organization. Employing data classification techniques helps enhance DLP's effectiveness. By discerning what data is critical and where it resides, organizations can apply specific policies to manage and protect that information.
Importance of DLP in Cybersecurity
The importance of DLP in cybersecurity cannot be overstated. As organizations increasingly rely on digital transformation, the chances of data breaches significantly increase. Here are some key reasons why implementing DLP is essential:
- Protection of Sensitive Information: Organizations handle a multitude of sensitive data types, including personal identifiable information, intellectual property, and financial records.1 DLP ensures that such information does not fall into unauthorized hands.
- Regulatory Compliance: Many industries are subject to strict regulations regarding data protection. Compliance with regulations like GDPR, HIPAA, and PCI DSS necessitates rigorous protocols for data handling. DLP provides the tools needed to meet these standards, avoiding hefty fines.
- Risk Management: Understanding the potential risks related to data loss or theft allows organizations to take proactive measures. DLP frameworks help assess vulnerabilities, thus minimizing exposure to threats.
- Incident Response: DLP plays a crucial role in immediate threat mitigation. When a potential data loss is detected, DLP solutions facilitate quick responses to prevent or minimize damage.
"In an era where data is a significant asset, the preservation and protection of that data are crucial for maintaining trust and integrity in business operations."
DLP networks, therefore, serve as a frontline defense mechanism. They equip organizations to handle increasing data threats, ensuring that sensitive information remains secure while enabling operational efficiency. Understanding and implementing DLP networks effectively is essential for cybersecurity professionals, IT specialists, and network administrators aiming to build resilient data protection frameworks.
Core Components of DLP Networks
Data Loss Prevention (DLP) networks consist of various critical elements that play a significant role in the protection of sensitive information. Understanding these core components is essential for developing an effective DLP strategy. Each component is interlinked, and together, they contribute to a comprehensive data protection framework. The following are the main components:
Data Discovery and Classification
One of the primary tasks of a DLP network is identifying sensitive data within an organization. Data discovery and classification processes ensure that all critical information is recognized and categorized appropriately.
Organizations need to have a clear understanding of their data landscape. This involves using automated tools to scan data repositories, track sensitive information, and classify it based on predefined criteria. Various types of data, such as personally identifiable information (PII), payment card information (PCI), and confidential business documents, should be classified differently according to their risk levels. The significance of this step cannot be understated; without a thorough discovery and classification process, organizations cannot adequately protect their data.
- Enhanced Visibility: Identifying sensitive data allows companies to focus their protection efforts where they are most needed.
- Regulatory Compliance: Many regulations require organizations to know where sensitive data resides, making classification a priority.
- Informed Policy Development: Classification aids in creating effective data protection policies.
Data discovery and classification not only mitigate risks but also provide a foundation for enforcing policies and responding to incidents.
Policy Enforcement Mechanisms
Once sensitive data is discovered and categorized, the next step is establishing and enforcing policies. Policy enforcement mechanisms dictate how data is handled, who has access to it, and the actions to take when unauthorized access attempts occur.
Policies should be comprehensive and tailored to meet an organization’s specific needs. This can include directives on data handling, sharing practices, and usage restrictions. Enforcement methods may involve integration with existing IT systems to ensure that policies are applied consistently across the network.
Some key aspects of policy enforcement mechanisms include:
- Access Controls: Implementing tiered access based on user roles.
- Monitoring: Continuous examination of data transfer to detect violations.
- Automated Actions: Configuring systems to automatically block, quarantine, or notify relevant personnel of policy breaches.
Effective policy enforcement can drastically reduce the risk of data loss or breaches and enhance an organization’s overall cybersecurity posture.
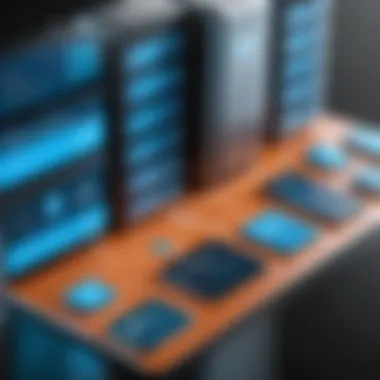
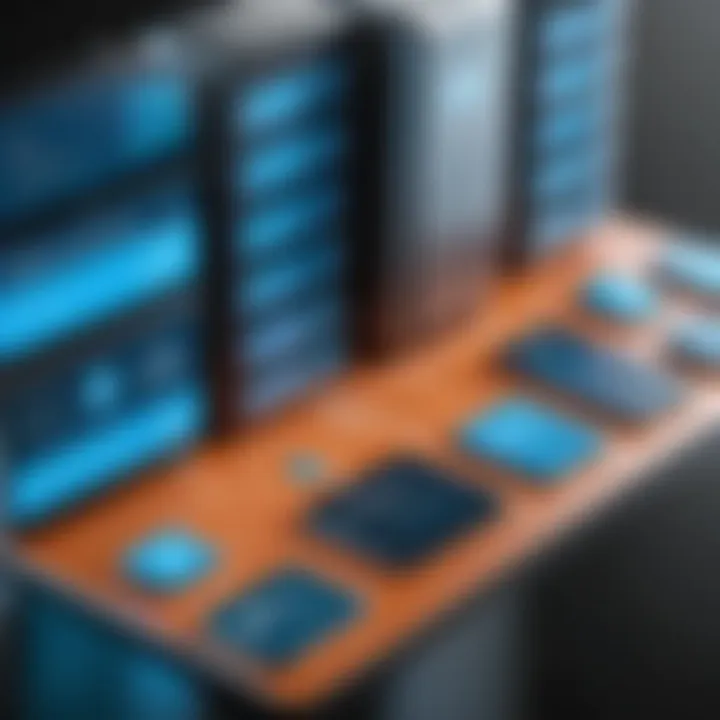
Incident Response Procedures
Despite best efforts, data incidents can still occur. Therefore, having robust incident response procedures in place is critical. These procedures outline the steps to take when a data breach or a policy violation is detected.
An effective incident response program must incorporate:
- Preparation: Develop an actionable plan that all employees are aware of, including roles and responsibilities.
- Detection: Utilize tools for real-time monitoring to swiftly detect incidents.
- Containment: Implement measures to minimize damage during the initial response to an incident.
- Eradication: Remove the cause of the breach to prevent future occurrences.
- Recovery: Restore systems and data after an incident has been handled.
- Review: Conduct a post-incident evaluation to learn from the experience.
The key to managing data loss effectively lies in having a coordinated and rehearsed response plan that enables organizations to react promptly and effectively in the event of a data loss incident.
DLP Deployment Strategies
Data Loss Prevention (DLP) deployment strategies are fundamental for any organization aiming to protect sensitive data. Choosing the right deployment method can significantly affect the efficiency and effectiveness of a DLP system. These strategies can be categorized broadly into three types: Endpoint DLP Solutions, Network DLP Solutions, and Cloud-Based DLP Solutions. Each has distinct characteristics, advantages, and implementation considerations.
Endpoint DLP Solutions
Endpoint DLP Solutions focus on securing data at the devices where it is created or used. Such coverage is crucial because endpoints—laptops, desktops, and mobile devices—are often where data is most vulnerable. Companies face various threats at this level, from malware to insider breaches.
Implementing Endpoint DLP involves installing software that monitors and controls data transfers at the endpoint. This action ensures that sensitive information does not leave the device inappropriately. Results include better control over data access and handling capabilities, providing peace of mind to stakeholders.
Benefits of Endpoint DLP include:
- Direct monitoring of data transfers at the user level.
- Granular control over data sharing (e.g., emailing, printing).
- Real-time alerts on potential data breaches.
However, managing Endpoint DLP effectively demands careful consideration. Organizations must balance usability with security. Too many restrictions may frustrate users, leading to workarounds that could undermine security protocols.
Network DLP Solutions
Network DLP Solutions, on the other hand, monitor and protect data while it is in transit across the network. They serve as a crucial line of defense against external threats and unauthorized access attempts. By analyzing traffic patterns, Network DLP tools help identify potentially risky behaviors and stop data breaches before they occur.
Key techniques in Network DLP include data encryption, protocol filters, and traffic analysis.
Points of interest in Network DLP are:
- Comprehensive visibility across the entire network.
- Policy-based controls that can automatically enforce data protection.
- Scalable systems that grow with your organization’s needs.
Despite its advantages, Network DLP is not a silver bullet. It may require substantial investment in infrastructure and the potential for performance bottlenecks if not managed properly. Therefore, it is essential to regularly review traffic patterns and adjust policies accordingly.
Cloud-Based DLP Solutions
Cloud-Based DLP Solutions represent the archetype of modern data protection. As organizations increasingly use cloud services, protecting sensitive data in these environments through dedicated DLP solutions has become essential.
These solutions help manage data residing in cloud services like Google Drive, Microsoft OneDrive, and Dropbox. They focus on maintaining visibility and control over how sensitive data is stored and shared in cloud platforms.
Some advantages of Cloud-Based DLP include:
- Centralized management of data policies across dispersed locations.
- Automatic updates, reducing the burden on IT staff.
- Flexible scalability to adapt to your business model easily.
However, challenges exist. Organizations may need to ensure compliance with regulations concerning data sovereignty, which can complicate operations. Users' trust in cloud providers is also a critical factor as organizations depend on third-party services for data security.
"Implementing a well-thought-out DLP deployment strategy is essential for minimizing risk and protecting sensitive data across multiple platforms."
Challenges in Implementing DLP Networks
Implementing Data Loss Prevention (DLP) networks involves several complex challenges that organizations must navigate. Understanding these challenges is crucial for creating effective strategies to protect sensitive data. Each challenge not only has implications for the deployment of DLP systems but also influences their overall effectiveness in safeguarding organizational data integrity.
User Privacy Concerns
User privacy is a critical issue in DLP network implementation. Employees often fear surveillance and the potential misuse of their personal data. This concern can lead to resistance against DLP initiatives. Organizations must clearly communicate the purpose of DLP systems, emphasizing their role in protecting the company's sensitive information rather than infringing on users' personal privacy.
Moreover, regulatory compliance such as GDPR brings forth stricter requirements about data handling practices. Organizations need to ensure that their DLP policies align with such regulations, emphasizing transparency and fairness in data collection. This might include measures such as anonymizing user data or granting employees access to information about what data is being monitored.
Balancing Security and Usability
Another significant challenge is finding a balance between security measures and usability. A DLP system that is too restrictive may hinder employee productivity and lead to frustration. It is essential to develop policies that protect data without creating barriers for users. Implementing a risk-based approach to data protection can help tailor security measures according to different data types and usage scenarios.
For example, sensitive data might necessitate stricter controls, while less critical information could have more lenient access policies. Continuous user feedback is also valuable in refining these policies. Only through ongoing evaluation can organizations maintain a functional balance between security and user experience.
Evolving Threat Landscapes
Finally, the landscapes of cybersecurity threats are constantly changing. New vulnerabilities, sophisticated attacks, and emerging technologies create an environment where DLP networks must continuously adapt. This evolution can be challenging for organizations that may struggle to keep pace with the latest threats.
To manage this challenge effectively, organizations should invest in continuous training for their cybersecurity teams. Staying informed about the latest trends in threat intelligence can empower teams to identify vulnerabilities early and make necessary adjustments to DLP policies. Collaboration with technology vendors can also provide insights into emerging risks, ensuring the DLP systems remain robust against potential exploits.
"In the face of evolving threats, proactive strategies and continuous learning are key to maintaining effective DLP networks."
In summary, while the implementation of DLP networks presents notable challenges, understanding and addressing these obstacles can significantly enhance data protection efforts. Focusing on user privacy, balancing security and usability, and adapting to evolving threats will improve the effectiveness of DLP initiatives.
Best Practices for DLP Network Management
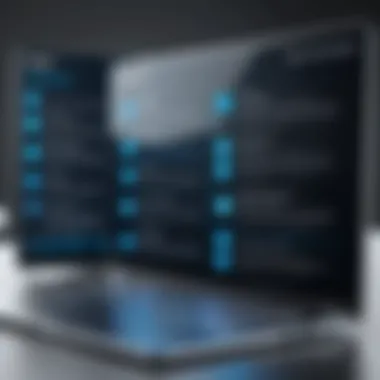
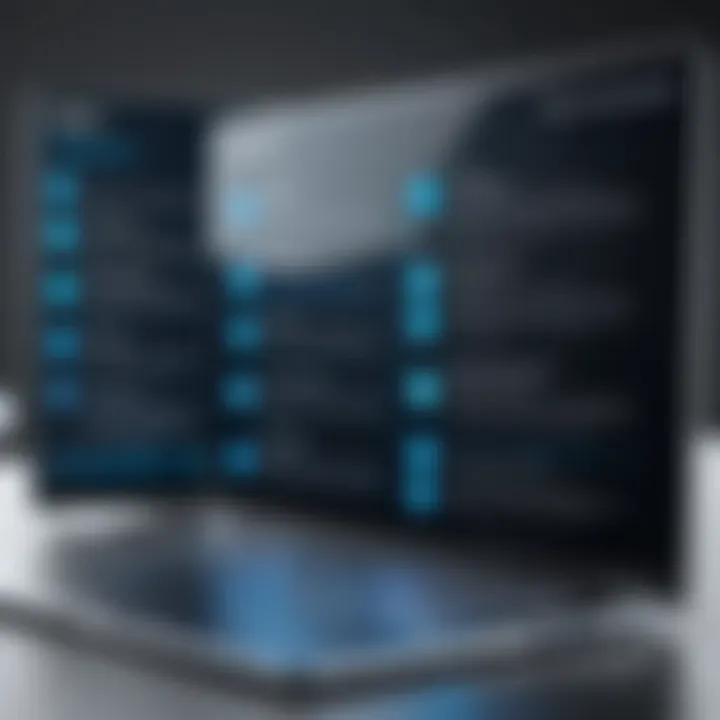
Effective management of Data Loss Prevention (DLP) networks is critical for safeguarding sensitive data. Best practices play a significant role in ensuring that DLP networks operate efficiently and adapt to changing requirements. These practices not only enhance data security but also help organizations to meet compliance standards and maintain trust with stakeholders.
A DLP strategy must be dynamic to address evolving threats and technological advancements. Organizations should prioritize certain elements to maximize the benefits of their DLP initiatives.
Regular Policy Reviews and Updates
Policies are at the core of any DLP strategy. Regular reviews ensure that the existing policies align with current business objectives and compliance requirements. An outdated policy can lead to security gaps where sensitive information may be exposed. Organizations should implement a schedule for policy evaluation, ideally on a quarterly basis. This schedule allows for the identification of any necessary changes, such as adapting to new data types or compliance regulations like GDPR or HIPAA.
Benefits of regular policy updates include:
- Alignment with business goals: As organizations change, so do their data protection needs.
- Adaptation to new threats: Cyber threats evolve rapidly; policies must reflect the latest intelligence.
- Compliance adherence: Keeping policies updated avoids penalties associated with non-compliance.
Regular feedback from stakeholders, such as IT teams and users, can provide insights into practical challenges encountered in policy enforcement. Such feedback mechanisms enhance the relevance and effectiveness of policies.
User Training and Awareness Programs
User behavior is a critical factor in the success of DLP initiatives. A well-informed workforce can significantly reduce the risk of accidental data breaches. Organizations should invest in comprehensive training and awareness programs tailored to different employee levels.
These programs should address:
- Identifying sensitive data: Employees must understand the types of data classified as sensitive within the organization.
- Understanding access control: Educating users on who can access specific data and the importance of safeguarding it.
- Recognizing common threats: Phishing attacks and social engineering practices can lead to data loss. Training can help identify these threats before they result in breaches.
Effective training initiatives should include periodic refresher courses to reinforce knowledge. Real-life examples can demonstrate the impact of poor data handling practices and potentially encourage a culture of security awareness within the organization.
Monitoring and Reporting Mechanisms
Monitoring is essential for maintaining the effectiveness of DLP policies. Continuous monitoring of data access and use allows organizations to detect anomalies or suspicious activities. Implementing automated reporting mechanisms also streamlines this process, providing regular insights into the DLP program’s effectiveness.
Key components of monitoring and reporting include:
- Real-time alerts: Immediate notifications for policy violations or anomalous behavior enable swift responses to potential breaches.
- Dashboards for analysis: Centralized dashboards facilitate data visualization and trend analysis.
- Compliance reporting: Automated reports help fulfill audit requirements and regulatory demands.
Organizations need to analyze the collected data regularly. Insights gained from monitoring can inform policy adjustments and training needs, creating a continuous improvement cycle.
Conclusion: DLP network management requires a continual commitment to best practices. Regular policy reviews, user training, and effective monitoring create a robust framework that not only secures sensitive data but also enhances the organization’s overall data protection strategy.
Technological Solutions in DLP
Data Loss Prevention (DLP) strategies increasingly rely on advanced technological solutions to protect sensitive information. Amid the rise in data breaches and regulatory pressures, integrating technology within DLP frameworks is crucial. Employing effective technological solutions can enhance detection capabilities, streamline policy enforcement, and ultimately bolster an organization’s data protection measures.
Machine Learning in DLP
Machine Learning (ML) has emerged as a transformative element in advancing DLP systems. It allows organizations to analyze large datasets efficiently, making it possible to detect anomalies and patterns that signify potential data breaches.
The implementation of machine learning algorithms assists in the continuous learning process of DLP systems. For example, a DLP system utilizing ML can profile user behaviors, adapting its response when deviations are noted. This adaptability significantly reduces false positives, which can burden IT staff and erode the effectiveness of security measures. Effective model training requires clear definitions of expected behaviors and constant adjustment based on new threats.
Benefits of ML in DLP include:
- Intelligent Threat Detection: Identifying unusual activities that could lead to data loss.
- Automated Policy Application: Facilitating swift response based on recognized threats without human intervention.
- Enhanced Accuracy: Reducing cases of false alerts, thereby improving operational efficiency.
Encryption Technologies
Encryption technology is a foundational pillar for DLP strategies. The essence of encryption lies in its ability to guard sensitive data, rendering it unreadable to unauthorized users. There are various encryption methods, such as symmetric and asymmetric encryption, which serve different security purposes and requirements within DLP architectures.
In DLP, encryption can be applied in several scenarios:
- Data-at-Rest Encryption: Securing stored data on devices or servers, protecting it from unauthorized access during storage.
- Data-in-Transit Encryption: Ensuring data remains secure while being transmitted over networks, which is essential in preventing interception by malicious entities.
- Full Disk Encryption: Protecting entire disk drives ensures that even if hardware is compromised, the data remains secure.
Adopting encryption technologies contributes to compliance with various regulatory frameworks, requiring organizations to safeguard sensitive information. Furthermore, implementing encryption minimizes the impact in the event of a data breach. While encryption adds an essential layer of security, careful planning is necessary to manage encryption keys effectively, as loss of keys can lead to permanent data loss.
"Investing in technological solutions such as machine learning and encryption is critical for a robust DLP strategy. These tools transform the way organizations approach data protection, creating a resilient framework for years to come."
To conclude, the integration of machine learning and encryption technologies into DLP strategies represents not just an enhancement but rather a necessity. The benefits derived from these technological solutions are profound, impacting everything from operational efficacy to regulatory compliance.
Future Trends in DLP Networks
Future Trends in DLP networks hold significant weight for organizations aiming to enhance their data security strategies. The rapid evolution of technology presents new opportunities and challenges for protecting sensitive information. Companies must stay aware of these trends to effectively plan and implement DLP measures. In this section, we will explore several key trends that are shaping the future of Data Loss Prevention.
Integration with AI and Automation
The integration of Artificial Intelligence (AI) and automation into DLP systems is a transformative trend. Organizations are increasingly leveraging these technologies to enhance their data protection capabilities. AI helps in identifying patterns in data handling and detecting anomalies that might signal a breach. When combined with automation, DLP systems can react swiftly to potential threats without human intervention. This efficiency is critical given the rising number of cyber threats.
Benefits of AI integration include:
- Improved accuracy in detecting potential threats.
- Reduced response times during incidents.
- The ability to learn and adapt from ongoing data usage patterns.
However, organizations must consider the complexities involved in implementing AI within DLP frameworks. A clear understanding of how algorithms function and the potential biases they may introduce is essential for effective application.
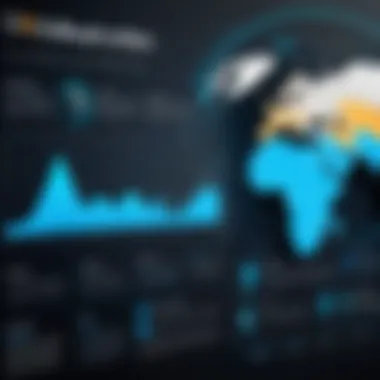
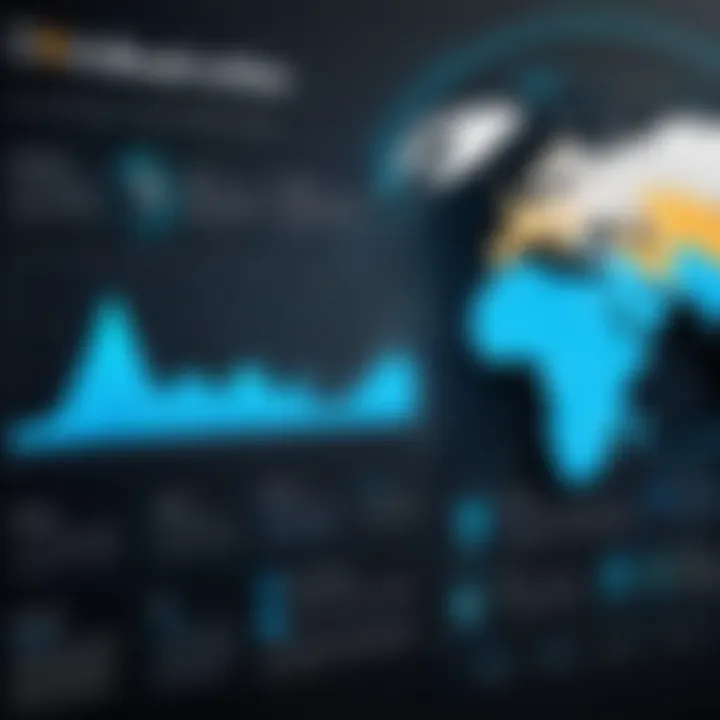
Zero Trust Security Frameworks
Zero Trust is a security model based on the principle of "never trust, always verify." This approach is increasingly adopted in DLP strategies. Organizations implement Zero Trust to ensure that data access is continuously monitored, and that Permission to access data is only granted after rigorous verification.
Key elements of a Zero Trust approach include:
- Multi-factor authentication for users.
- Continuous validation of user access levels.
- Strict monitoring of data flows within and outside the organization.
Adopting this framework significantly strengthens data protection. However, organizations need to address the complexity involved in fostering a Zero Trust environment, which includes comprehensive policies and the technology to enforce them.
Adapting to Remote Work Environments
The shift towards remote work has changed how organizations manage data protection. Many businesses now allow employees to access sensitive information from various locations. This change poses unique challenges, especially regarding data security.
Organizations must implement DLP tools that accommodate remote work. Some key considerations are:
- Developing clear remote work policies that outline data handling procedures.
- Utilizing end-user training to promote awareness of security best practices.
- Enabling secure access methods, such as Virtual Private Networks (VPNs).
By adapting DLP strategies for remote work environments, companies can ensure that sensitive data remains protected while maintaining operational flexibility.
In summary, the future trends in DLP networks emphasize the integration of advanced technology and the adaptation to new working conditions, highlighting the need for ongoing evaluation and adjustment of strategies.
Staying ahead of these trends will be critical for organizations aiming to bolster their data security frameworks.
Case Studies of DLP Network Implementation
Examining case studies of Data Loss Prevention (DLP) network implementations provides invaluable insights into real-world applications of DLP strategies. These examples highlight the practicalities, successes, and challenges faced by various organizations. Understanding these aspects equips cybersecurity professionals, IT specialists, and network administrators with knowledge that can foster more effective DLP strategies.
Success Stories in Various Industries
Numerous industries have effectively leveraged DLP networks to protect sensitive data. For instance, the finance sector often faces stringent regulatory compliance. A prominent bank adopted a DLP solution that integrated both endpoint and network monitoring capabilities. This approach significantly reduced data leakage incidents by 80% within the first year. By focusing on comprehensive data classification and continuous monitoring, the bank ensured that sensitive customer information remained secure.
Similarly, healthcare organizations are under constant pressure to protect patient information. A notable hospital system implemented a cloud-based DLP solution that monitored data transfers and flagged unauthorized access attempts in real-time. This proactive strategy not only safeguarded patient data but also improved staff awareness about data security protocols.
These examples illustrate that tailored DLP implementations can yield substantial benefits that align with industry-specific requirements. Organizations that prioritize DLP see enhanced trust from customers and regulatory bodies.
Lessons Learned from DLP Failures
Not all DLP implementations succeed. Several organizations have faced challenges that highlight critical lessons to be learned. For example, a global retailer invested significantly in a DLP solution but experienced multiple data breaches shortly after deployment. A thorough analysis revealed that the policies governing the DLP system were poorly defined. Employee interactions with sensitive data were not adequately addressed, leading to unintentional leaks.
Another case involves a technology firm that attempted to enforce a rigid DLP policy without prior user training. Employees found the system cumbersome, leading to workarounds that actually increased the risk of data loss. After a review, the company realized that involving employees in the policy formation could have fostered a culture of security awareness and compliance.
These examples show that DLP strategies must consider human factors. Effective implementation relies on clearly defined policies, comprehensive user training, and constant engagement with employees. Failure to do so can render even the most advanced DLP systems ineffective.
Legal and Compliance Considerations
Legal and compliance considerations play a crucial role in the effectiveness of Data Loss Prevention (DLP) networks. Organizations must navigate various laws and regulations that govern the handling of sensitive data. Failure to comply with these regulations can result in hefty fines, legal repercussions, and reputational damage. This section addresses the important aspects of compliance, highlighting regulatory frameworks and data sovereignty issues that every organization should be aware of when implementing DLP strategies.
Regulatory Frameworks Impacting DLP
Organizations are increasingly held accountable by a myriad of regulatory frameworks that directly impact their DLP initiatives. Some key regulations include General Data Protection Regulation (GDPR), Health Insurance Portability and Accountability Act (HIPAA), and the Payment Card Industry Data Security Standard (PCI DSS). Each framework lays down stringent guidelines on how organizations must manage, store, and protect sensitive data.
Adhering to these regulations requires a thorough understanding of specific elements:
- Data Identification: Organizations must identify what data is considered sensitive within the context of each regulation.
- Access Controls: Regulatory frameworks often mandate strict access controls and permissions to ensure that only authorized personnel can handle sensitive information.
- Audit Trails: Keeping detailed logs of who accesses data, where it is stored, and how it is transmitted is essential for compliance.
An organization's readiness to comply with these regulations can mitigate risks associated with data breaches, enhancing its credibility and trustworthiness in the eyes of customers and partners.
Data Sovereignty Issues
Data sovereignty refers to the legal concept that data is subject to the laws of the country in which it is collected and processed. In an increasingly globalized digital landscape, organizations must be aware of where their data resides and the associated legal implications. For example, storing data in a cloud service provider that operates in a foreign jurisdiction can complicate compliance with local laws.
Key considerations include:
- Storage Locations: Understanding where data is stored helps assess the legal obligations tied to that data.
- Cross-Border Data Transfers: Transferring data across borders can expose organizations to different laws and regulations, which may have conflicting requirements.
- Local Regulations: Some countries have their own strict laws governing data privacy, making it essential to align DLP strategies with local compliance requirements.
Addressing data sovereignty issues effectively safeguards sensitive information while affirming compliance with international regulations. Each organization must factor these considerations into their overall DLP strategy to ensure robust protection of their data assets and maintain regulatory compliance.
The End
In the critical discourse surrounding Data Loss Prevention (DLP) networks, the conclusion serves as both a culmination and a springboard for future action. Understanding the implications of DLP is paramount for organizations that handle sensitive information. It is evident that the execution of a robust DLP strategy is complex but necessary for safeguarding data integrity. By recognizing the varied components and challenges associated with DLP networks, organizations can navigate the landscape of data protection more effectively.
The benefits of implementing a DLP network are multifaceted. These solutions not only protect against data breaches but also enhance compliance with regulatory frameworks. A strong DLP framework fosters a culture of security awareness among employees, thereby minimizing insider threats that can compromise sensitive data.
When reflecting on the critical aspects mentioned throughout the article, professionals must contemplate the adaptability of their DLP strategies. This adaptability addresses not just the current threat environment, but also future-proofing against evolving risks. Addressing user privacy concerns, escalating user training, and establishing clear incident response procedures play significant roles in creating a holistic data protection environment.
Ultimately, as technological advancements continue to reshape the landscape of cybersecurity, organizations must be proactive in leveraging insights from case studies and industry best practices. The confluence of legal, technical, and human factors underscores the necessity for a comprehensive strategy in DLP management.
"Being proactive in adopting robust DLP solutions is not just an IT task; it is a core business imperativ that can safeguard an organization’s reputation and longevity."
Summary of Key Takeaways
- Understanding and Defining DLP: Organizations must comprehend what DLP entails and why it is crucial for protecting sensitive information.
- Components of DLP Networks: Essential features include data discovery, classification, and policy enforcement mechanisms, all integral to effective DLP implementation.
- DLP Deployment Strategies: Examination of endpoint, network, and cloud-based solutions helps tailor the approach to individual organizational needs.
- Challenges in DLP Implementation: Recognizing issues such as user privacy, security versus usability, and evolving technological threats is vital for success.
- Best Practices for Management: Regular policy updates, user training, and thorough monitoring should drive DLP efforts in any organization.
- Technological Solutions and Future Trends: Awareness of the integration of AI, Zero Trust frameworks, and adaptation to remote work settings will dictate future strategy and security.
- Legal and Compliance Implications: Understanding regulatory frameworks and data sovereignty issues can enhance compliance efforts and reduce legal risk.
By synthesizing all these elements, stakeholders in the realm of cybersecurity can gain pivotal insights into how to fortify their data protection frameworks effectively.