Data Integrity vs Data Quality: Understanding the Differences
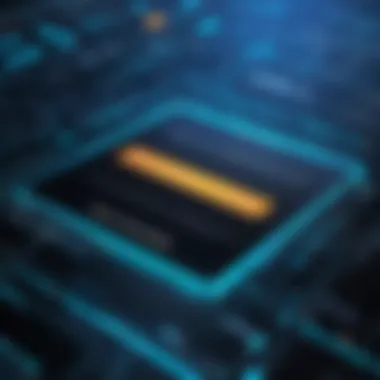
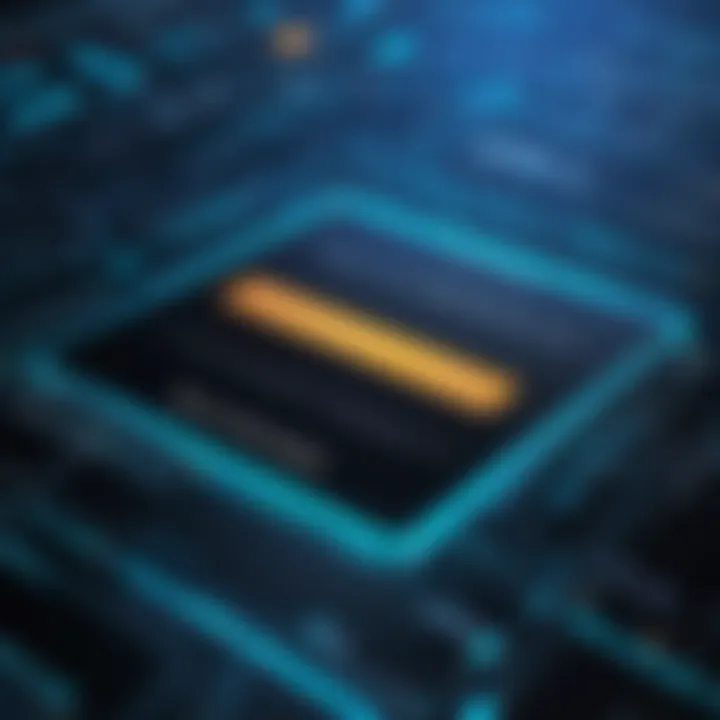
Intro
In todayās fast-paced digital landscape, where data flows at the speed of light, the concepts of data integrity and data quality weave a complex but critical narrative. Both elements play essential roles in guiding organizations towards smarter decision-making and enhanced performance. While they may seem similar at first glance, the distinctions between them are significant.
Data integrity often focuses on the accuracy and consistency of data throughout its lifecycle. It is about the reliability of that data, ensuring that what you have remains trustworthy from input to output. On the other hand, data quality spans broader aspects such as relevance, completeness, and comprehensiveness of the data gathered. Simply put, data integrity is about being correct, while data quality is about being useful.
As we dive deeper into this examination, we will explore various facetsādefinitions, implications, and the dynamic relationship between the two concepts. Making informed choices about data management can foster a culture that cherishes precision and effectiveness in organizations.
"Data is a precious commodity. The value of insight it provides is only as good as the integrity and quality of that data."
Throughout the sections that follow, we will dissect the relevance of these concepts in the modern data-driven era and highlight how aligning them can lead to successful organizational outcomes.
Understanding data integrity and data quality not only aids professionals but also sets the grounds for building trust in digital processes. With the underlying principles elucidated, let us march forward into the nuanced landscape of data management.
Understanding Data Integrity
In the realm of data management, comprehending data integrity serves as a critical foundation. This concept resonates through every layer of information systems, influencing how data is collected, stored, managed, and ultimately utilized. At its core, data integrity is about maintaining and assuring the accuracy and consistency of data over its entire lifecycle. The significance of this lies not just in the reliability of the data, but also in the trust and dependability organizations can place in their data-driven decisions. Without a solid grasp of data integrity, organizations can easily find themselves navigating murky waters, where data might mislead rather than empower.
It's important to note that data integrity is not merely an IT concern; it pervades all business areas. When integrity is assured, data can be relied upon for making decisions that have real-world implicationsāranging from minor operational shifts to major strategic directions.
Moreover, organizations that prioritize data integrity tend to exhibit enhanced operational efficiency. This improvement often arises from standardized processes that yield consistent results, which cascade through the entire data management strategy. Let's delve deeper into specific aspects of data integrity by defining it clearly, understanding its importance in data management, and outlining its key principles.
Definition of Data Integrity
At a granular level, data integrity refers to the accuracy and consistency of data. This encompasses various constructs, ensuring that data remains unaltered during processing, storage, and access. The term can be categorized into several forms:
- Physical Integrity: Deals with the physical storage of data. It ensures that data remains intact and recoverable despite hardware failures.
- Logical Integrity: Involves maintaining data's correctness and reliability, often through data validation protocols and constraints in a database.
In practice, data integrity ensures that every piece of information is precise and congruent, enabling organizations to depend on their data to execute tasks efficiently and effectively.
Importance in Data Management
When organizations understand and uphold data integrity, several vital benefits emerge:
- Trust in Data: A high level of data integrity nurtures trust among stakeholders. When data is reliable, it fosters a more transparent decision-making environment.
- Operational Efficiency: Steadfast data integrity can reduce operational costs linked to data errors and mishandling. This, in turn, streamlines processes across various departments.
- Regulatory Compliance: Numerous industries face stringent regulations regarding data management and reporting. Data integrity practices can assist organizations in aligning with compliance requirements, thus averting potential penalties.
Ultimately, data integrity is more than a technical standard; it is a strategic asset that underpins organizational effectiveness.
Key Principles of Data Integrity
To effectively manage data integrity, one should adhere to several key principles that determine how data is treated throughout its lifecycle:
- Validation: This involves implementing mechanisms to check the accuracy and quality of data as it is entered into a system.
- Consistency: Ensuring that data is presented uniformly across different platforms and usages. Inconsistent data can lead to confusion and misinterpretation.
- Security: Protecting data from unauthorized access or alterations, which directly safeguards its integrity.
- Accountability: Assigning responsibility for data management to specific individuals or teams is crucial. This helps ensure adherence to data integrity principles.
- Auditability: Creating trails that allow tracking of changes and movements within data systems is essential for maintaining integrity over time.
Implementing these principles forms a robust foundation for data integrity, significantly contributing to the overall effectiveness of data management within an organization.
Exploring Data Quality
Data quality can be a linchpin in the entire machinery of data management. Understanding this component is crucial, especially when organizations rely heavily on data analysis for decision making. When diving into data quality, we consider various aspects that contribute to its significance and impact.
Definition of Data Quality
Data quality refers to the overall utility of a dataset, illustrating how well it meets the requirements of its intended purpose. This encompasses accuracy, consistency, completeness, timeliness, and relevance. To put it plainly, if the data isn't good, then no matter how fancy the tools or how skilled the analysts, the results can be misleading. Poor data quality can lead organizations down the garden path, resulting in decisions based on faulty information.
Significance in Decision Making
When it comes to decision making, high-quality data is akin to a compass steering clear of the murky waters. Organizations need reliable information to maneuver market trends, customer behaviors, and operational strategies. Good data inspires confidence, while bad data breeds hesitation. We often hear the saying, "garbage in, garbage out," underscoring how poor input leads to subpar output.
Dimensions of Data Quality
Accuracy
Accuracy is about precision. It ensures that the data values are correct and reflect true values. For instance, a customer's address needs to be spot on; even a small error could lead to costly misdeliveries. The key characteristic of accuracy is that it provides a trustworthy foundation for data-driven decisions. This dimension becomes increasingly significant in sectors where details can make a world of difference, like in medical records.
Unique features include ongoing maintenance and validation processes that uphold accuracy over time. While it may require significant effort to achieve, its advantages far outweigh the drawbacks.
Completeness
Completeness looks at whether all necessary data is present. Just having some of the information may not cut it. Consider a sales report missing half of the sales figures; drawing any meaningful conclusion from this would be a shot in the dark. The primary trait of completeness ensures decision-makers have a holistic view, enabling comprehensive analysis.
A unique feature of completeness is that it requires a culture of diligence regarding data entry and collection protocols. Its disadvantage could be the resource investment needed to gather and maintain all relevant data, but itās often essential for contextual understanding.
Consistency
Consistency checks whether data values are in agreement. For instance, if one database shows a customer as being male and another indicates female, this inconsistency can confuse prior decisions and compromise business logic. This characteristic is critical as it fosters reliability in data across various systems and platforms. A unique feature of this dimension involves establishing data standards across departments. While it may demand extra time to reconcile differences, the result is harmonized datasets.
Timeliness
Timeliness relates to whether data is up to date. Data that once was solid might become stale; for example, using outdated market research can mislead a business strategy. Timeliness is crucial in quick-response environments where decisions may pivot on the most current data available. The key aspect is that timely data allows organizations to seize opportunities and mitigate risks without delay. The downside? Constantly updating data can be resource-intensive, yet it's paramount in maintaining a competitive edge.
Relevance
Lastly, data must be relevant. In an age inundated with information, it's important to filter out the fluff and focus on what truly matters for the issue at hand. Relevance involves aligning the data collected with the actual need or question being posed. Its hallmark is the continual assessment of what is necessary to support decision-makingārelevant data leads to sharper insights, while irrelevant data can cloud judgment. One unique aspect of relevance is its dynamic nature, needing periodic re-evaluation to ensure useful metrics. The challenge lies in maintaining a clear line between relevant and extraneous data, which can sometimes be subjective.
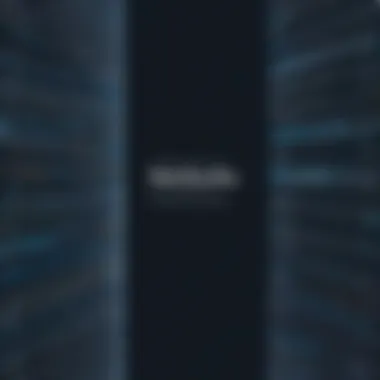
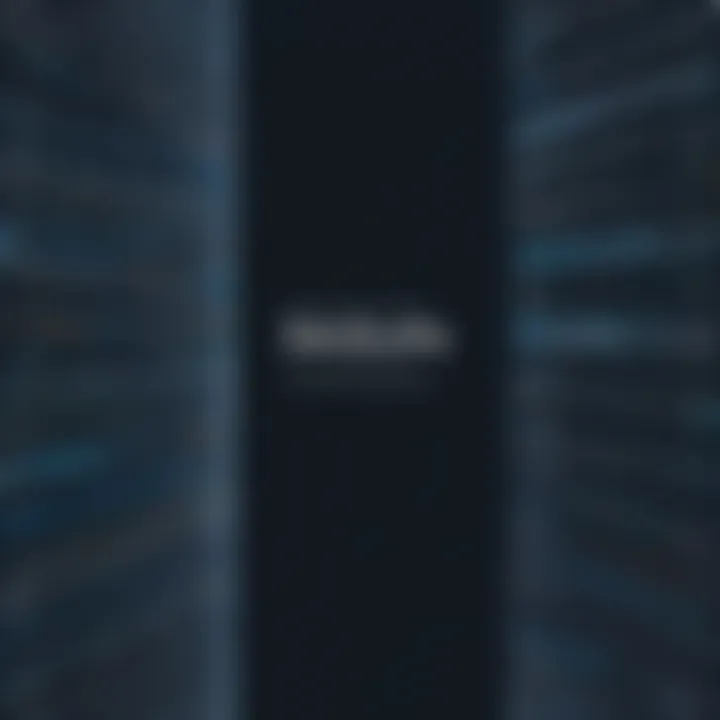
High-quality data isnāt just a luxuryāitās a necessity if organizations hope to thrive in a competitive landscape.
Comparative Analysis: Data Integrity vs Data Quality
Data integrity and data quality often get tossed around in the same basket, but they are two distinct concepts that play different roles in effective data management. Understanding the nuances between them is not just academic; it has real-world implications for anyone working with data, from cybersecurity professionals to IT specialists.
To illustrate their difference, think of data integrity as the gatekeeper of your data's reliability, while data quality focuses on its utility. If data integrity ensures that the information is accurate and cannot be tampered with, data quality ensures that it's actually useful and relevant for decision-making. Let's dig deeper into these aspects.
Core Differences
When dissecting these two entities, several contrasts become apparent:
- Data Integrity: Primarily concerned with the accuracy and consistency of data throughout its lifecycle. It's about ensuring that the data remains unchanged and intact from the moment it's captured to its eventual use.
- Data Quality: This revolves around how well data meets the needs of its users. This includes factors like accuracy, completeness, and timeliness. Quality data should not only be correct but also current and relevant to the task at hand.
"Data integrity lays the groundwork, while data quality crafts the masterpiece."
This perspective emphasizes the sequential relationship between the two: integrity sets the stage for quality. Just because the data is accurate and preserved doesnāt guarantee it delivers value if itās outdated or irrelevant.
Interdependencies between Integrity and Quality
Looking at the interdependencies shines a light on how these two facets influence each other:
- Best Practices: Maintaining data integrity leads to higher data quality outcomes. If the foundational integrity is compromised, the resulting quality can only suffer. Conversely, implementing quality measures can help reinforce integrity. Regular audit trails can validate consistency while validating the match between data elements.
- Stakeholder Confidence: Organizations with strong integrity frameworks boost data quality, thereby increasing stakeholder confidence. Users can trust their decisions based on reliable information.
- Regulatory Requirements: Many industries must comply with regulations that mandate both data integrity and quality. Ensuring that these two elements are in sync can be as critical for compliance as it is for operational effectiveness.
Real-World Implications
In practice, the divide between data integrity and quality has far-reaching implications:
- Business Decisions: Poor data quality, stemming from low data integrity, can lead to misguided strategies, wasted resources, and tarnished reputations.
- Operational Efficiency: Organizations that prioritize integrity manage their data better, which streamlines processes and reduces the duplication of efforts, significantly impacting operational efficiency.
- Customer Trust: Companies that uphold standards in both areas create a reputation for reliability. When customers know their information is handled with care, trust naturally grows, leading to deeper relationships.
In summary, a thorough comparative analysis reveals that understanding the core differences, interdependencies, and practical implications of data integrity versus data quality is essential for any professional in the cyber world. Not just at the theoretical level, but in everyday applications, these insights can guide strategy and operational improvements.
Challenges in Maintaining Data Integrity
In the intricate world of data management, maintaining data integrity isnāt just a checkbox on a project plan. Rather, itās a fundamental backbone that supports the trustworthiness and coherence of all data-driven decisions within an organization. Problems that arise in this area can ripple through an organization's workflow, leading to misinformation, poor analytics, and ultimately, flawed business strategies. Therefore, understanding the challenges is not optional; itās essential.
Identifying and tackling these hurdles can lead to stronger data governance and a more data-literate culture. Letās take a closer look at three primary adversaries in the bid to uphold data integrity.
Technical Vulnerabilities
Technology isnāt infallible; issues like software bugs, outdated systems, and hardware failures can derail even the best-laid plans for data integrity. When software tools become outdated, their effectiveness diminishes significantly. Organizations must remain vigilant, ensuring updates and patches are applied regularly. For example, a company reliant on a legacy database management system may be shin-deep in trouble when they discover that the system cannot handle the increased load during peak business periods.
- Programmatic Vulnerabilities: Many systems can inadvertently introduce errors due to coding mistakes, which can lead to data corruption.
- Connectivity Issues: Inconsistent network connections can affect the accuracy of data input and retrieval, which in turn, jeopardizes data integrity.
Addressing these technical vulnerabilities requires ongoing training for IT personnel, investment in modern technology, and maintaining a regular review cycle to assess software and hardware performance.
Human Errors
It's an age-old saying that to err is human, and in the data world, this couldnāt be truer. Human errors are one of the leading causes of compromised data integrity. Whether itās a simple typo when entering data or misunderstanding data inputs during quality checks, these mistakes can snowball into epic miscalculations that skew insights and decision-making.
- Training Accountabilities: A lack of training can lead to a mishmash of data entry techniques, creating inconsistencies across datasets.
- Process Adherence: When employees aren't conscientious about following data handling protocols, lapses can occur, leading to incorrect data propagation.
Employing robust training programs and standardizing procedures can significantly reduce human error's impact on data integrity.
Impact of Cybersecurity Threats
As organizations embrace digital transformation, they become targets for cybercriminals looking to exploit vulnerabilities. Data integrity is under constant threat from attacks such as ransomware, phishing, and data breaches.
The potential fallout from these threats can be damaging and far-reaching:
- Data Manipulation: Cybercriminals can invade systems and modify, delete, or steal data, compromising the very essence of data integrity.
- Erosion of Trust: Once data integrity is compromised through a breach, the resulting loss of customer trust can be catastrophic for an organizationās reputation.
In light of these threats, itās crucial for organizations to reinforce cybersecurity protocols. This means investing in advanced cybersecurity measures, including firewalls, intrusion detection systems, and regular vulnerability assessments.
"Data integrity isnāt just a checkbox; itās the very foundation upon which your analysis stands. If data is compromised, your conclusions are as good as dust."
Obstacles in Achieving Data Quality
To navigate the turbulent waters of data management, understanding the obstacles in achieving data quality is essential. High-quality data is the bedrock of sound decision-making, yet several challenges can creep in, leading to compromised data integrity and weakened data quality. By identifying these roadblocks, organizations can develop strategic approaches to mitigate their effects and enhance overall data governance.
Data Silos
Data silos are one of the most significant barriers to achieving quality data. When different departments or teams store their data independently, it creates an environment where information is not only fragmented but may also become inconsistent. This separation hinders effective data sharing and collaboration. For instance, if the marketing team has a dataset thatās not synchronized with sales, the insights generated may lead to conflicting strategies. The consequences can be dire ā organizations may end up with decisions based on outdated or incomplete information.
"Data silos breed confusion; a house divided against itself cannot stand."
To break down these silos, fostering an environment that encourages inter-departmental communication is vital. Implementing integrated data management solutions can facilitate access and ensure that everyone is on the same page. Additionally, a culture that values data sharing over top-secret hoarding can pave the way for synergy across departments.
Inconsistent Data Entry Practices
Inconsistent data entry practices can wreak havoc on data quality. When multiple individuals are tasked with entering data, variations in how they record information can lead to discrepancies. Think of a real estate firm where one agent uses abbreviations while another spells everything out. These differences can magnify when data is aggregated from various sources, leading to inaccuracies that eventually undermine trust in the data.
To combat this issue, organizations must standardize their data entry protocols. Training employees on best practices and utilizing predefined dropdowns or templates can help maintain consistency. As a result, accuracy is enhanced, making the data more reliable for analysis and reporting.
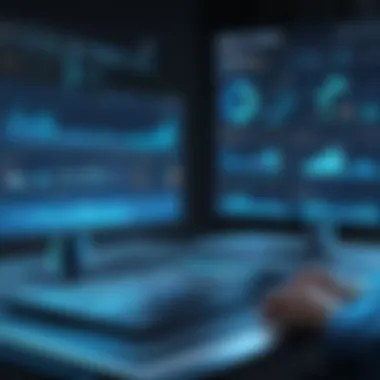
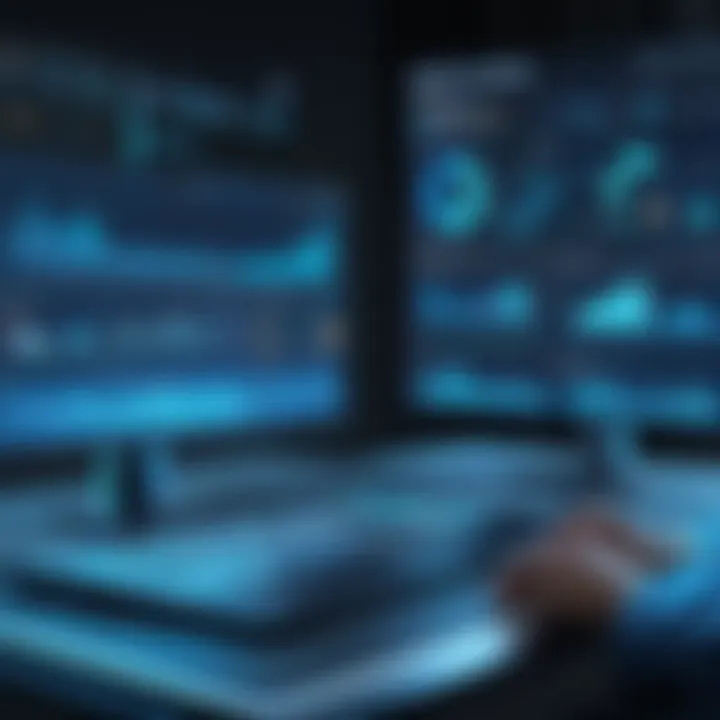
Legacy Systems Challenges
Legacy systems often become an albatross around the neck of data quality efforts. These outdated systems may not only lack compatibility with new technologies but also struggle to meet the evolving needs of organizations. The risk here is monumental: poor integration of legacy systems can lead to discrepancies, stymying the flow of accurate data.
Moreover, legacy systems can hinder the adaptation of data quality tools. For example, if a financial institution is still using older software for client records, it may not support modern data analytics tools designed to identify quality issues. This can leave organizations operating in the dark, where critical insights remain hidden.
To improve data quality in the context of legacy systems, a balanced approach is recommended. Gradually transitioning to modern systems while training staff on their use can ensure that the data pool remains clean and useful. Over time, this will foster a stronger data governance framework, resulting in better decision-making capabilities.
By addressing these obstacles, organizations can significantly improve their data quality. In a data-driven world, overcoming these challenges is not merely beneficial; it's imperative for sustained success.
Strategies for Enhancing Data Integrity
In todayās data-driven environment, ensuring data integrity is crucial for organizations seeking to maintain the trustworthiness of their information assets. Integrity means more than just keeping data intact; itās about guaranteeing that data is accurate, consistent, and reliable throughout its lifecycle. Employing robust strategies for enhancing data integrity is not just a technical requirement but a cornerstone for building a resilient data governance framework within any organization. Here we discuss several effective strategies that can be employed to achieve this goal.
Implementing Robust Access Controls
One of the foremost strategies for enhancing data integrity involves implementing robust access controls. This includes defining user roles and permissions to ensure that only authorized personnel have access to sensitive data. By implementing principles like least privilege access, organizations can significantly reduce the risk of unauthorized data manipulation or breaches.
Moreover, keeping a close eye on who accesses what data and when can stop many potential integrity issues before they arise. Setting up a multi-factor authentication system adds an extra layer of security, ensuring that even if credentials are compromised, unauthorized access remains limited. Establishing clear protocols for revoking access during employee transitions can also play a key role.
Conducting Regular Audits
Another key element in maintaining data integrity is the conducting of regular audits. Periodic reviews help uncover inconsistencies and gaps in data management practices that could lead to integrity issues. These audits should not only assess compliance with established protocols but also recognize areas for improvement.
Organizations should adopt a structured audit framework. This could include:
- Routine checks: Implement regular check-ups on database entries and alterations.
- Error tracking: Document and analyze errors to identify patterns.
- Remediation processes: Ensure that there are clear methods in place for addressing any discrepancies found during audits.
This proactive approach allows organizations to quickly address any issues, fostering a culture of accountability and continuous improvement that ultimately strengthens their data integrity.
Utilizing Encryption Techniques
Utilizing encryption techniques stands as a third pillar in strategies aimed at enhancing data integrity. Encryption secures data at rest and in transit, preventing unauthorized access that could impair data accuracy and reliability. Advanced encryption standards, such as AES (Advanced Encryption Standard), offer strong protection against data breaches.
Both symmetric and asymmetric encryption methods can be considered based on specific needs. When utilizing encryption:
- Data at Rest: Encrypt sensitive databases and backups so that even if a breach occurs, the data remains unreadable.
- Data in Transit: Always use secure protocols, like HTTPS or VPNs, to protect data being sent from one point to another.
By making encryption a standard practice in data management, organizations can further bolster data integrity through robust protection against threats and tampering.
"Data integrity is not just about keeping data intact but ensuring its accuracy and reliability, which ultimately supports trust in organizational decision-making processes."
By integrating these strategies into their data practices, organizations not only protect against data breaches but also foster greater trust in their information systems. The interplay of proper access controls, regular audits, and effective encryption creates a more resilient framework that upholds data integrity in a complex and evolving digital landscape.
Methods for Improving Data Quality
Ensuring robust data quality is not just a buzzword; itās a critical component of effective data management. With organizations increasingly reliant on data to drive decisions, the significance of maintaining high standards of data quality can't be overstated. Poor data can lead to flawed insights which in turn may harm strategic initiatives. Thus, understanding and implementing methods for improving data quality becomes vital.
Data Cleansing Processes
Data cleansing, often dubbed the backbone of effective data management, involves identifying and correcting inaccuracies and inconsistencies in datasets. Think of it as the tidying up of a messy room ā your data needs to be organized and accurate to be useful.
Key benefits of a solid data cleansing process include:
- Enhanced Accuracy: Regularly checking for errors minimizes the risk of making decisions based on faulty information.
- Reduced Duplication: Cleansing eliminates duplicated entries, ensuring that each data point is unique. This is particularly essential in customer databases.
- Improved Reliability: Clean data builds trust among stakeholders; when data is reliable, so too is the decision-making grounded in it.
To execute data cleansing effectively, tools can be employed such as OpenRefine or specialized software like Talend. These solutions automate the arduous task of identifying errors and integrating them into a clean dataset.
Standardizing Data Entry Procedures
Standardization in data entry practices ensures consistency across datasets. Imagine trying to piece together a puzzle where some pieces have different shapes ā that's what inconsistent data entry feels like.
There are numerous benefits to this approach:
- Uniformity: Having a defined template for data entry minimizes variations. For instance, deciding whether to enter dates in DD/MM/YYYY or MM/DD/YYYY formats is crucial.
- Efficiency: Training staff on a standard process speeds up data entry as everyone follows the same format. This makes it easier to teach new employees.
- Error Reduction: When everyone uses the same procedures, the chance of mistakes decreases significantly, resulting in cleaner data.
Itās beneficial to provide clear guidelines and training materials for staff, emphasizing the importance of standard practices. Tools like Microsoft Excel allow for data validation rules that can aid in this standardization effort.
Adopting Data Governance Frameworks
A strong data governance framework lays a solid foundation for maintaining data quality. In essence, data governance governs the availability, usability, integrity, and security of the data utilized in an organization. Itās the structure that supports the overall management of data assets.
Implementing a good framework comes with various considerations:
- Accountability: Establishing clear ownership of data elements helps ensure that someone is always responsible for maintaining data quality.
- Policy Development: Well-defined policies regarding data usage and management practices foster a culture of respect for data quality.
- Regulatory Compliance: A robust governance framework assists in meeting industry regulations, such as GDPR or HIPAA, which mandates how personal data should be handled.
Additionally, requiring regular review of governance policies keeps them relevant as the organization evolves. Effective governance can significantly elevate the integrity and quality of data, leading to informed decision-making.
Ultimately, a trifecta of practicesādata cleansing, standardization of procedures, and robust data governanceāacts as a protective umbrella over the organizationās data management efforts, promoting a culture where quality is paramount.
"Data quality is not an achievement; itās a continuous process."
By embracing these methods, professionals in the cybersecurity and IT spheres can foster environments rich in quality data. This ensures the insights drawn from this data are both accurate and actionable.

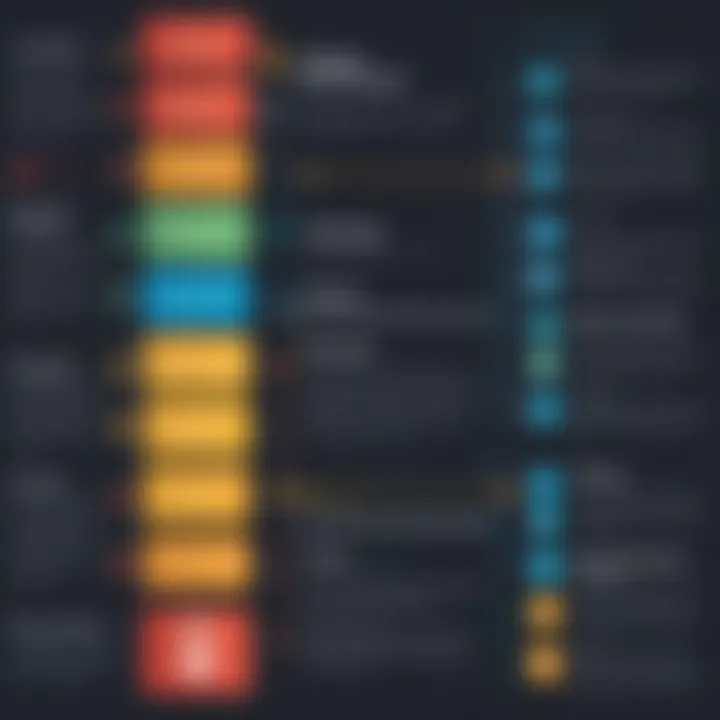
The Role of Technology in Data Management
In today's digital landscape, managing data effectively isn't just optional; it's a necessity. With the explosion of data generation, technology plays a pivotal role in ensuring that both data integrity and data quality are maintained. The integration of advanced tools and methodologies allows organizations to navigate the complex data terrain with precision and confidence. Here, we will explore the significance of technology in this context and break down its various components.
Data Integrity Tools
Data integrity tools are designed to ensure that information remains accurate and reliable throughout its lifecycle. One key tool for maintaining data integrity is database management systems. These systems, such as Microsoft SQL Server or MySQL, not only manage data efficiently but also implement safeguards such as referential integrity constraints and access controls.
Another important tool is the hash function. This algorithm creates a unique string of characters for data, making it easy to verify that the information hasnāt been altered. For instance, when a sensitive file is transmitted, a hash value can be generated and compared upon receipt to check for any unauthorized changes.
Additionally, tools like data governance platforms help ensure that policies around data integrity are met while providing auditing capabilities. This encompasses features such as monitoring user behavior, tracking changes, and maintaining version history. These platforms are crucial not just for compliance, but for instilling trust in the data.
Data Quality Frameworks
Maintaining high data quality is crucial for informed decision-making. Data quality frameworks, such as the DAMA-DMBOK (Data Management Body of Knowledge), provide comprehensive guidelines that can help organizations structure their data management practices.
These frameworks emphasize several key dimensions of data quality, including accuracy, completeness, and consistency. They outline formal processes for data cleansing, ensuring that obsolete or erroneous data is routinely identified and corrected.
Moreover, organizations can adopt technology-driven solutions like ETL (Extract, Transform, Load) programs and data profiling tools. ETL solutions, such as Talend or Informatica, allow users to pull together data from disparate sources, transform it according to the defined frameworks, and load it into a unified system. Data profiling tools help assess the quality of data and identify areas that need improvement.
Emerging Technologies Impact
Looking ahead, emerging technologies are set to revolutionize data management as we know it. Artificial intelligence, machine learning, and blockchain technology are forging new paths for integrity and quality. For example, AI-driven algorithms can analyze vast datasets to identify patterns and anomalies that might indicate data breaches or quality issues. These tools can flag potential errors before they lead to significant problems, allowing for proactive management.
Blockchain offers a transparent way of ensuring data integrity, as it creates a tamper-proof ledger that records every transaction. This can be vital for sectors like finance or healthcare, where trust in data authenticity is paramount. With blockchain, any changes to the data can be traced back to the original source, providing a clear audit trail.
In summary, technology isnāt just a supplementary aspect of data management; itās the backbone that supports the intricate dance between data integrity and data quality. As methods continue to evolve, staying current with these tools and frameworks is essential for any organization aiming to thrive in a data-driven world.
"The challenge is not to rely on technology alone but to utilize it wisely to bolster our data practices."
By investing in the right tools and embracing innovative frameworks, organizations can enhance their decision-making processes and, consequently, their success.
Case Studies: Data Integrity vs Data Quality
In the fast-paced world of data management, case studies play an essential role in illuminating the practical implications of data integrity and data quality. These case studies serve as concrete illustrations of how these two elements operate in real-world scenarios, shedding light on their significance and the intricate relationships they share. Through various examples, we can observe the profound impact these concepts can have on organizations, particularly in terms of decision-making, operational efficiency, and risk management.
Exploring specific cases not only emphasizes the value of sound data practices but also serves to highlight the pitfalls that can occur when either integrity or quality is compromised. As data continues to be touted as a critical asset for organizations, understanding the lessons gleaned from past experiences becomes invaluable.
Successful Integrations
Successful implementations of data integrity and quality can significantly enhance an organizationās operational efficiency. One notable example is a healthcare provider that integrated advanced data management systems to ensure accurate patient records. By leveraging data validation techniques and regular audits, the organization was able to maintain high levels of data integrity. This fidelity in their data led to effective patient care decisions, improved collaboration across departments, and overall strengthened trust from patients and regulatory bodies alike.
- Key aspects of this successful integration:
- Automated Data Entry: Incorporating automation reduced human error, thus preserving data accuracy.
- Real-Time Data Monitoring: Through constant oversight, the organization was able to rectify inconsistencies quickly.
- Training Programs: Comprehensive staff training ensured that everyone understood the importance of maintaining data standards.
The amalgamation of these factors not only facilitated the layering of work processes but also made them resilient against potential data breaches. This case emphasizes that focusing on both data integrity and quality is not just about compliance; itās about ensuring that every piece of information serves its intended purpose efficiently.
Failures and Lessons Learned
Failures in managing data integrity and quality often expose organizations to severe consequences. A well-known case involved a retail giant that faced a massive data breach. The company had neglected its data security protocols and had not kept its data entries consistent across different platforms. Following the incident, it was discovered that the integrity of their customer data was severely compromised. Sensitive information was leaked, resulting in a loss of consumer trust and significant financial penalties.
- Lessons to be taken from this failure:
- Implement Comprehensive Data Governance: All levels of data management should involve strict governance policies to prevent lapses.
- Regular Training and Updates: Employees need continuous education on best practices. This includes understanding data privacy regulations and the implications of compromised data.
- Invest in Technology: Relying on outdated systems can lead to inconsistencies and security risks. Employing modern tools that enhance both integrity and quality is essential.
Failures like this remind us of the dire consequences of overlooking these critical areas. Organizations must understand that while data is an asset, maintaining its integrity and quality is a responsibility that cannot be ignored.
A well-managed data landscape ensures not only operational excellence but also aligns with strategic goals.
Future Trends in Data Management
In an ever-evolving landscape where data reigns supreme, understanding the future trends in data management isn't just relevant; itās essential. With the continuous surge of dataāsome estimates suggest that the global data sphere will hit 175 zettabytes by 2025āorganizations must adapt to ensure data integrity and quality remain prioritized. This section presents a roadmap that highlights notable elements, benefits, and considerations regarding future trends in the field.
The Evolution of Data Practices
As business environments shift towards digitalization, data management practices are also going through a significant metamorphosis. Cloud computing has radically transformed how organizations store and manipulate data. It allows for scalability, enabling businesses to expand while managing their data effectively. Furthermore, data lakes have become popular, facilitating the storage of vast amounts of structured and unstructured data.
Another crucial evolution is the application of real-time data analytics. With the aid of technologies like Apache Kafka, organizations are now able to harness real-time information to make immediate decisions. This shift not only enhances decision-making processes but also aligns with the need for timely data quality assessment.
"In the age of immediacy, lagging behind in data practices could result in losing competitive edge."
Adopting agile methodologies in data management allows teams to be more responsive to change. Adding automation processes enhances both data integrity and quality, reducing the risk of human errorāan Achilles heel for many organizations.
Increased Importance of Data Governance
As organizations acknowledge the value of their data, the focus on data governance is sharpening. Companies are recognizing that effective governance can set the foundation for maintaining data integrity while also enhancing data quality. Proper data governance frameworks govern how data is accessed, shared, and utilized, safeguarding it from misuse and ensuring its reliability.
The compliance landscape is becoming increasingly strict. Regulations such as GDPR and CCPA underscore the need for robust frameworks that not only maintain data quality but address ethical considerations. For organizations, the hammer of regulatory compliance can be daunting, emphasizing the importance of having clear data governance protocols in place.
Data stewardship roles are becoming more prevalent, ensuring a dedicated team oversees governance policies while working to enhance data quality and integrity consistently. This systemic approach supports not just compliance but generates trust among stakeholders.
AI and Automation's Role
The integration of Artificial Intelligence and automation into data management is not merely a trend; it is a necessary evolution. AI algorithms support data cleaning and validation tasks that were conventionally performed manually. By automating these processes, organizations can maintain high standards of data integrity while reducing costs and time spent on mundane tasks.
Machine learning models can be trained to identify anomalies in large datasets, ensuring that data quality remains uncompromised. With their predictive capabilities, organizations can anticipate potential data quality issues before they become a bane.
Moreover, AI tools are being utilized to enhance data governance by mapping data flows, helping organizations to better understand their data landscape and how different elements interrelate. The wise implementation of AI tools provides an edge that distinguishes forward-looking companies from their competitors.