Data Classification Strategies for Cloud Readiness
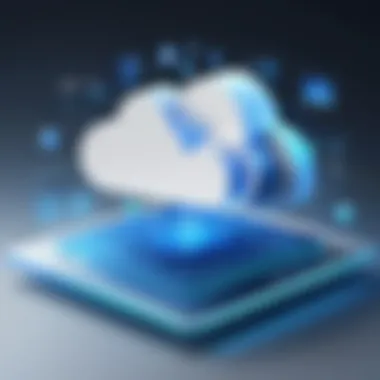
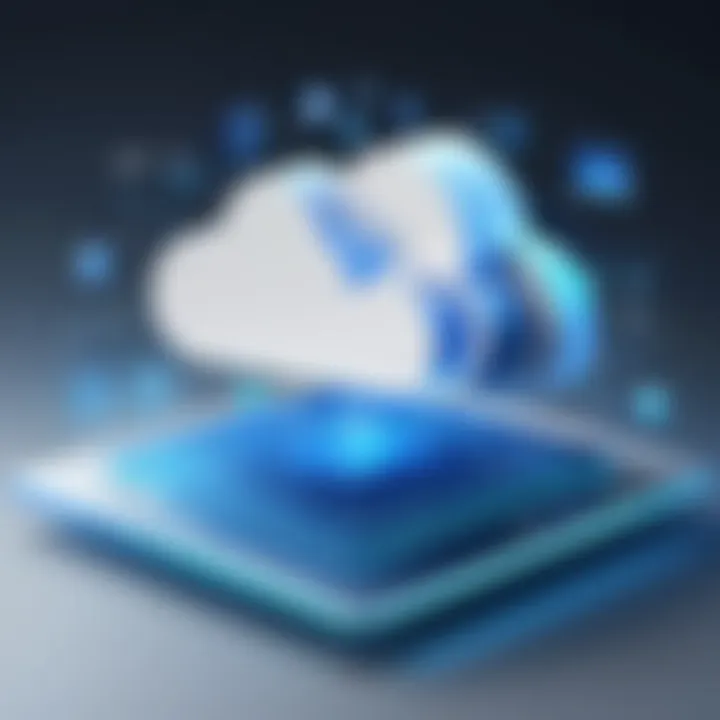
Foreword to Cybersecurity and Network Security Convergence
In our ever-connected world, the lines between networking and security have blurred. The ascendancy of cloud technology has ushered in a new era, where data, once confined within traditional boundaries, now resides in a vast, dynamic environment. Cybersecurity is no longer an afterthought; it’s the linchpin that holds the integrity of digital operations together.
As organizations migrate assets to the cloud, they are faced with a myriad of challenges that necessitate a robust data classification framework. This framework is essential for ensuring compliance with diverse regulations while simultaneously optimizing resource allocation.
The Significance of Cybersecurity Today
The importance of cybersecurity cannot be overstated. Daily, countless users depend on online services, whether for banking, shopping, or communication. Effective data classification not only protects sensitive information but also fosters trust between entities and their users. In today's climate, where breaches are ubiquitous, the imperative to safeguard digital assets is greater than ever.
The Evolution of Security and Networking Integration
The evolution from isolated security mechanisms to a unified approach is indicative of the changing threat landscape. Once, network security was a standalone entity, often reactive. Today’s paradigm encourages proactive measures, merging cybersecurity practices with networking strategies. This convergence ensures that protective measures are embedded directly within the infrastructure that supports cloud environments.
"Data classification isn't just a box to check; it's the backbone of any effective cybersecurity strategy, especially in the cloud."
Understanding Data Classification
Data classification pertains to the systematic identification and organization of data based on various criteria. This sets the groundwork for efficient data management and security planning. Without a well-defined data categorization strategy, organizations expose themselves to significant security vulnerabilities and compliance risks.
Why It Matters in Cloud Readiness
In the context of cloud readiness, properly classifying data allows organizations to allocate resources effectively and maintain regulatory compliance. By categorizing data according to its sensitivity, businesses can tailor their security measures, ensuring that the most critical data enjoys the highest protections.
Practical Approaches to Data Classification
Implementing an effective data classification strategy is not without its challenges. Here are several methodologies that organizations can adopt to enhance their cloud readiness:
- Sensitivity labeling: Assign clear labels for different levels of data sensitivity, such as public, internal, confidential, and restricted.
- Automated Classification Tools: Leverage machine learning algorithms to identify and classify data in real-time based on established policies. This can greatly reduce manual errors and enhance efficiency.
- Regular Audits: Frequent assessments of data classification and protection measures ensure that organizations can adapt to changing data landscapes and emerging threats.
Impact on Security Posture
Every data classification decision feeds into the organization's overall security posture. The better the classification process, the more effective the protective strategies. This interdependence between classification and security must be emphasized, as neglecting classification can lead to systemic vulnerabilities.
Closure
In navigating the complexities of cloud data management, professionals in the field must appreciate the significance of data classification. It interlinks with broader themes of cybersecurity, compliance, and resource management, providing a foundation for sound strategic decisions. As threats evolve and new technologies emerge, remaining agile and adaptable in data classification practices will be crucial for ensuring long-term security and cloud readiness.
Understanding Data Classification
In today's rapidly evolving digital landscape, understanding data classification is no longer just a nicety; it's a necessity. With businesses increasingly dependent on cloud solutions coupled with advanced technologies, the way we handle and protect data has become paramount. Data classification serves as the bedrock of a robust cybersecurity strategy, enabling organizations to effectively categorize and manage their information assets based on their sensitivity and importance.
The focus here is on enhancing cloud readiness. The essence of this topic transcends simple categorization; it's about arming businesses with the information they need to make informed decisions regarding data utilization, compliance, and security.
Defining Data Classification in the Digital Age
In the digital age, data classification can be seen as a systematic approach to organizing data based on predefined criteria. This criteria can be anything from data sensitivity levels to the intended use of the information. By categorizing data appropriately, organizations can apply the necessary security measures, ensure faster retrieval, and streamline data management.
For instance, think of data classification like sorting fruit at a market. You wouldn't want to mix up the delicate berries with rough-skinned oranges. The same logic applies to data – sensitive data should be isolated and given extra protection, while less sensitive data can be stored with fewer constraints. One could argue that without proper classification, businesses are metaphorically throwing all their data into one basket, leaving them vulnerable to leaks and non-compliance.
Importance of Data Classification
The significance of data classification cannot be overstated. First and foremost, it enhances security. When data is classified effectively, businesses can pinpoint which information needs the most protection. This ensures that sensitive data, such as personal customer information or intellectual property, is adequately shielded from unauthorized access.
Additionally, effective classification aids in compliance with various regulations. Many industries are governed by strict requirements on how data should be handled. By implementing a structured classification system, organizations can demonstrate their commitment to compliance and minimize the risk of hefty fines and legal ramifications.
Some key points regarding the importance of data classification include:
- Resource Allocation: By identifying the most critical data, businesses can prioritize their resources better, ensuring that they focus on protecting what truly matters.
- Operational Efficiency: A well-organized data structure allows for quicker access to necessary information, thus speeding up decision-making processes.
- Risk Management: Understanding the classification of data helps in identifying potential risks and planning appropriate mitigative measures.
Frameworks for Data Classification
In today's digital landscape, where data is often likened to the new oil, having a robust framework for data classification can make all the difference in optimizing cloud readiness. Frameworks offer a structured way to categorize and manage data, inflating the visibility and effectiveness of data handling processes. By implementing a classification framework, organizations can streamline their data governance practices, enhance compliance efforts, and bolster their overall security posture.
The significance of these frameworks is far-reaching. They not only simplify decision-making on data usage but also arm organizations with the tools to prioritize data sensitivity and compliance requirements. Embracing these frameworks allows businesses to understand their data environment better and address specific concerns like security gaps, risk exposure, and operational inefficiencies. While there’s flexibility in using various models and approaches, sticking to a well-defined classification framework can yield multiple benefits.
Overview of Classification Models
Classification models serve as the backbone of any data classification framework, guiding organizations through the process of categorizing data systematically. They can be as simple as basic manual categorizations or as complex as advanced machine learning models.
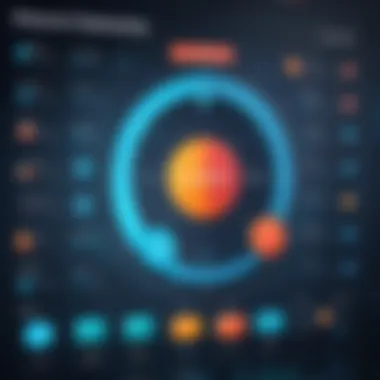
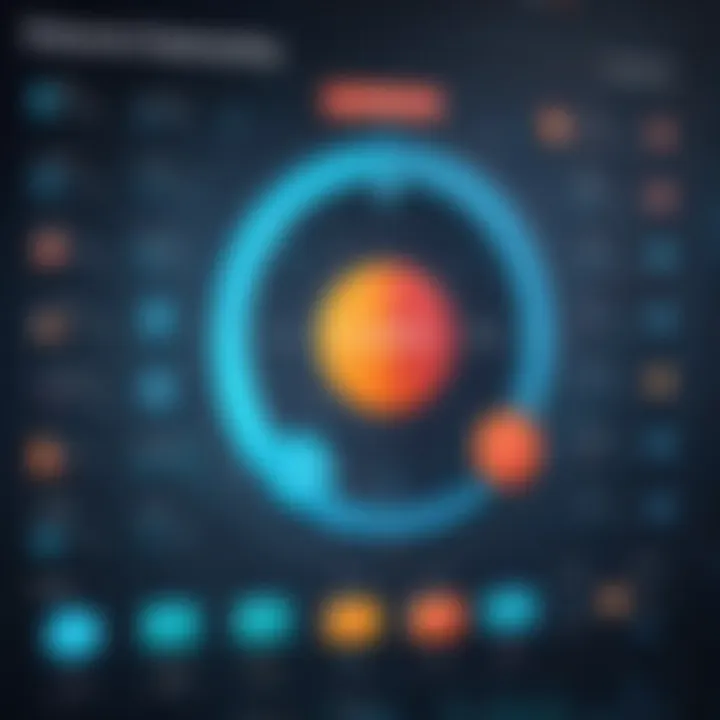
For instance, consider a financial institution. Its data may include sensitive personal information (like social security numbers) and non-sensitive transaction data. A useful classification model could segment this data into tiers or categories such as:
- Confidential: Data needing stringent access controls, like customer bank details.
- Internal Use: Information meant for internal management, perhaps operational reports.
- Public: Material that can be freely shared, like marketing materials.
Each level in this structure dictates how data is to be handled, stored, and protected, aligning with both regulatory obligations and organizational policies.
Automated vs. Manual Classification
In discussions about frameworks, the choice between automated and manual classification often emerges as a hot topic. Each method carries its pros and cons, depending on the scale and sensitivity of the data involved.
Manual classification involves human intervention, where professionals categorize data based on their knowledge and intuition. This method may provide more nuance but can be labor-intensive and prone to error due to oversight.
Automated classification, conversely, leverages software to analyze and categorize data according to predefined rules or machine learning algorithms. While it allows organizations to scale their efforts and enhances efficiency, care must be taken that the system is set up properly to avoid misclassification.
An optimal strategy could very well combine the two approaches, utilizing automation for initial sorting and manual oversight for critical categorizations.
Industry-Specific Classification Standards
Organizations across different industries face unique regulations and standards that dictate data classification practices. For example, in healthcare, regulations like HIPAA impose strict regulations on how patient data must be handled, categorized, and shared. In contrast, financial organizations may interact with standards like PCI DSS, focusing on the protection of cardholder information.
Adhering to these standards not only ensures legal compliance but also demonstrates accountability to clients and stakeholders. It becomes necessary for organizations to integrate these industry-specific requirements into their data classification frameworks, possibly requiring tailored models that align with their particular data challenges.
"Aligning your data classification framework with industry standards is not just wise but essential. Non-compliance can lead to hefty fines and reputational damage."
By mapping classification strategies to industry standards, organizations position themselves to better mitigate risks and enhance their overall cybersecurity posture. In the face of evolving technologies and regulatory climates, maintaining adaptability in classification methods becomes crucial.”
Data Classification Strategies
Data classification serves as a foundational element in cloud readiness. By effectively categorizing data, organizations can implement security measures that align with the nature of the information they are handling. This isn’t just about keeping the bad actors at bay; it’s also about ensuring compliance, optimizing resource allocation, and maintaining customer trust. Each strategy outlined here can influence how data is managed, accessed, and protected, leading to vital improvements in an organization’s overall data governance approach.
Categorization Based on Sensitivity
When discussing data classification, categorizing information based on sensitivity is paramount. Sensitive data can include personally identifiable information (PII), financial records, and health information—any piece of data that could harm or compromise individuals if mishandled.
By classifying data according to its sensitivity, organizations can prioritize their cybersecurity efforts. For instance, data designated as highly sensitive may warrant stronger access controls, encryption, and more robust monitoring than less sensitive information.
For example, an organization in the healthcare sector would treat patient health records with the highest level of security as any breach could lead to substantial fines and loss of trust. This distinction lets cybersecurity professionals allocate resources wisely and minimizes potential breaches. Here are some core levels of sensitivity that could be used:
- Highly Sensitive: Medical records, financial data, trade secrets.
- Moderately Sensitive: Employee details, internal communications, non-public financial reports.
- Low Sensitivity: Promotional materials, general company announcements.
"By aligning security protocols with data sensitivity, organizations can mitigate risks effectively while ensuring compliance with regulations such as HIPAA or GDPR."
Classifying by Functionality
Another strategic approach to data classification is categorizing information by its functionality within the organization. This involves organizing data based on its use cases, workflows, and processes. Functional classification not only helps streamline operations but also aids in navigation during data retrieval or audits.
For example, different departments within a large enterprise may utilize data differently. Sales might need quick access to customer interaction logs, while the finance team may prioritize data around transactions and budget allocations. By classifying data by its function:
- The enterprise can enhance responsiveness.
- It can improve overall visibility into its data landscape.
- User experiences can be tailored based on data access needs.
This method allows organizations to ensure that employees have the right level of access to the data they need to do their jobs, without unnecessary hurdles. Less sensitive data—such as marketing materials—could be more accessible, whereas vital financial documents would require stricter access controls.
Best Practices for Data Classification
Implementing data classification is not without its challenges; thus, adopting best practices can significantly ease the process. Here’s how organizations can effectively navigate this terrain:
- Develop Clear Classification Policies: Establish guidelines that define each classification level and the handling requirements associated with it. This clarity ensures everyone in the organization understands the protocol to follow.
- Empower Employees with Training: Regular training sessions for employees can help foster a culture of awareness. This training should cover the importance of data classification and the individual roles they play in it.
- Regularly Review and Update Classifications: Data is a living entity, constantly changing and evolving. Hence, classifications should be reviewed periodically to ensure they stay relevant.
- Leverage Automation Tools: Utilizing automated tools for classification can enhance accuracy and efficiency. Technologies that rely on machine learning can identify patterns and nuances in data that may elude manual classification efforts.
- Conduct Audits: Regular audits should be performed to ensure compliance with classification standards and to identify any gaps in the process. This step helps mitigate risks and underscores the commitment to data governance.
By following these best practices, organizations can embed data classification into their culture, ensuring a more proactive approach to data security that aligns with their cloud readiness initiatives.
Challenges in Implementing Data Classification
Implementing data classification is vital for organizations striving toward cloud readiness. The complexities involved can often trip up even the most adept teams. Understanding these challenges is key to uncovering the pathways that lead to effective classification strategies. From internal resistance to overwhelming data environments, each hurdle carries its weight and can impact overall success. Let's delve deeper into these challenges and explore their implications.
Resistance to Change Within Organizations
When it comes to data classification, change is a tricky business. People are creatures of habit. Getting employees to switch gears and adapt to new data classification protocols can be likened to pulling teeth. Many folks tend to cling to the old ways as they are familiar, even if they don’t serve the organization well anymore.
Resistance often stems from several sources. First, there's fear. Employees might worry that new systems will disrupt their workflow or, worse, jeopardize their job security. Then there's the lack of awareness; many might not grasp the importance of effective data classification. It’s not unusual to hear, "It’s always been done this way," echoing through the halls of companies when the subject comes up.
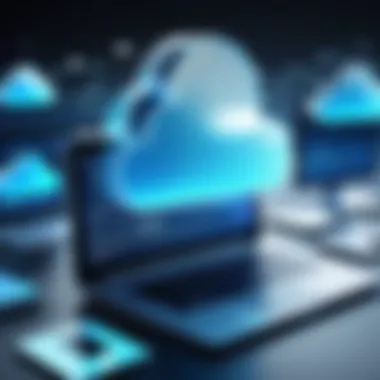
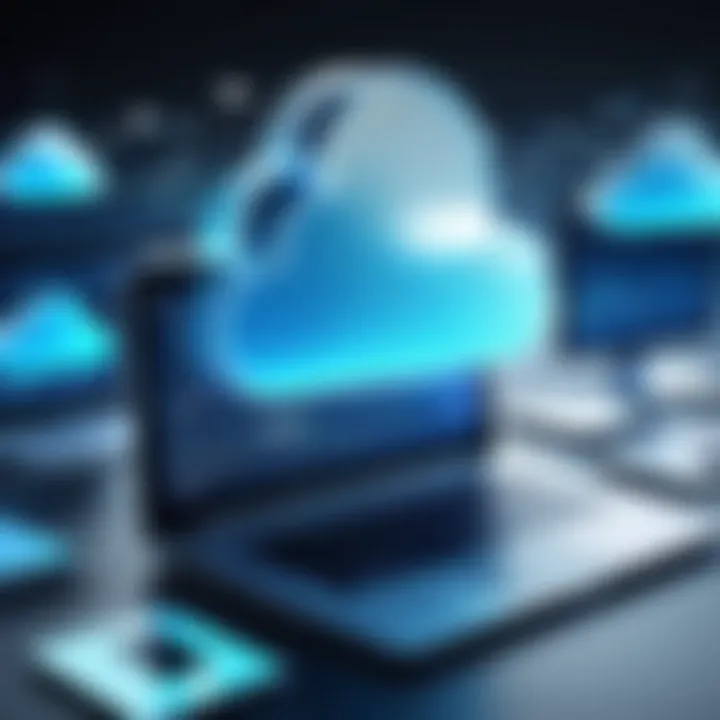
Engaging employees through training and communication becomes key. A collective understanding of the 'why' behind classification can help break down resistance. Layering in incentives for adaptation can be a game changer as well.
Complexity of Data Environments
Data environments today are like a messy room after a storm; they can be sprawling and chaotic. Organizations accumulate data across multiple platforms, ranging from on-premise servers to various cloud solutions. Each thread of data may vary in format, sensitivity, and purpose, making it difficult to entwine them into a coherent classification scheme.
Navigating these waters requires solid frameworks. But here's the kicker: The more intricate the environment, the harder it becomes to maintain an accurate classification system. Especially for organizations undergoing mergers or acquisitions, integrating disparate data systems can be daunting. In fact, many companies struggle with outdated or inconsistent metadata that complicates classification efforts even further.
Determining where to classify specific data types or sets could feel like finding a needle in a haystack. Without robust strategies and unified approaches, unnecessary risks arise. A systematic method could help bring clarity to this chaos.
Budget Constraints in Implementation
No matter how savvy an organization is, one constant will always be true: money talks. Budget constraints are often cited as a primary obstacle in implementing effective data classification processes. Setting aside funds for classification initiatives can feel like pulling from a barren well for many companies.
These constraints might stem from competing priorities. Organizations must balance expenses across numerous potential projects. It's not always easy to justify budget allocations for data classification when immediate costs seem steep.
Moreover, the longer organizations wait to invest in classification, the more costly it becomes in the long run, with potential regulatory penalties and security issues creeping in. To navigate these financial hurdles, organizations can consider phased implementation. Prioritizing the most critical systems for classification could ease budget strain while laying the groundwork for future enhancements.
Data classification is no longer a luxury; it is a prerequisite for success in the cloud era.
In summary, the challenges of implementing data classification shouldn't deter organizations from embarking on this essential journey. It demands introspection, innovative solutions, and a commitment to evolving with the times, but in the end, the benefits far outweigh the burdens.
The Role of Compliance in Data Classification
Navigating the labyrinthine landscape of data classification necessitates a firm grasp on the role of compliance. In today's increasingly digital era, organizations must adhere to a myriad of regulations designed to safeguard sensitive information. Compliance isn't just about ticking boxes; it acts as a catalyst for establishing standards that ensure data is handled properly, thereby generating trust among stakeholders.
Often, businesses that overlook compliance as a core aspect of their data classification strategy find themselves grappling with potential pitfalls. This reality underscores the need for rigorous compliance efforts that not only help in mitigating risks but also foster a culture of accountability within the organization. From ensuring data integrity to establishing clear protocols for access, compliance mechanisms play a vital role in shaping effective classification strategies.
Regulatory Requirements for Data Classification
Regulatory mandates, such as the General Data Protection Regulation (GDPR) and the Health Insurance Portability and Accountability Act (HIPAA), impose specific data classification requirements that organizations must adhere to. These regulations often delineate how data should be categorized based on its sensitivity and the implications of its misuse. For instance, GDPR emphasizes the need for data minimization and requires organizations to classify personal data to ensure it's handled appropriately.
Organizations need to conduct regular audits and assessments to understand their compliance obligations properly. Missing the mark on regulatory requirements can lead to not just hefty fines but also significant damage to reputation. To illustrate:
- GDPR Penalties: Companies can be fined up to €20 million or 4% of total global turnover for data breaches.
- HIPAA Violations: Non-compliance can result in fines ranging from $100 to $50,000 per violation, depending on the level of negligence.
This further reinforces the importance of embedding compliance into the heart of data classification processes. It’s not merely a checklist; it’s an ongoing commitment that shapes how data is managed.
Impact of Non-Compliance on Organizations
Failing to comply with data classification regulations can lead to disastrous consequences, both in terms of financial repercussions and operational setbacks. The fallout from non-compliance can significantly derail an organization's momentum, creating a ripple effect that permeates various facets of the business.
Firstly, the financial ramifications often hit hardest. Companies may face fines and penalties that can range into millions, directly impacting their bottom line. Such economic strain can hinder future growth prospects and affect investor confidence. Beyond the numbers, the reputational damage associated with data breaches can lead to a loss of customer trust, an invaluable currency in today’s marketplace.
"Protecting data is not just about following the law; it’s about maintaining your brand’s integrity."
In addition to financial aspects, organizations also deal with operational disruptions. Staff may need to be retrained, systems upgraded, and processes reevaluated to align with new compliance mandates. This can be taxing, diverting resources and attention away from critical business initiatives.
By proactively addressing regulatory requirements, organizations not only protect themselves but also set the stage for stronger security postures and a more reliable approach to data management.
Data Classification and Cloud Security
In an age where data reigns supreme, the interplay between data classification and cloud security becomes critically important. For organizations that rely on cloud services, adopting effective data classification strategies is not just a matter of efficiency; it also holds the key to safeguarding sensitive information against a growing array of cyber threats. Without a robust classification approach, businesses risk exposing themselves to significant vulnerabilities, which can have dire consequences on their reputation and bottom line.
By classifying data accurately, companies can implement security measures tailored to the specific sensitivity and compliance requirements of each dataset. This leads to improved data governance, which is crucial for preserving customer trust and ensuring regulatory adherence.
Ensuring Data Integrity in the Cloud
Data integrity refers to the accuracy and consistency of data throughout its lifecycle, and it is a cornerstone of cloud security. When a company fails to classify its data, the risk of unauthorized access becomes magnified. For example, lets say a financial firm stores both public and private client data in cloud repositories without any categorization. In the event of a breach, sensitive information may be exposed, leading to loss of client trust and potential legal issues.
To ensure data integrity, organizations should implement specific protocols that include:
- Regular audits: Conducting routine check-ups aids in identifying any unauthorized changes or anomalies in data.
- Version Control: Keeping track of changes to documents can prevent data loss and corruption.
- Backup Strategies: Maintaining up-to-date backups ensures that data can be restored to its original state in case of a breach or data corruption incident.
The integration of data classification with these protocols messages a strong commitment to safeguarding vital information, effectively closing the gaps that attackers might exploit.
Using Classification to Enhance Security Protocols
With classification comes the ability to apply customized security protocols. Different categories of data call for different protective measures. For instance, categorized data like Personally Identifiable Information (PII) or financial records should be treated with a higher level of scrutiny compared to lesser sensitive data.
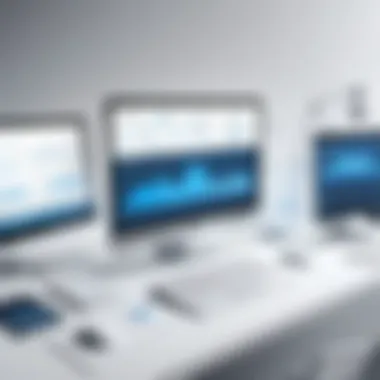
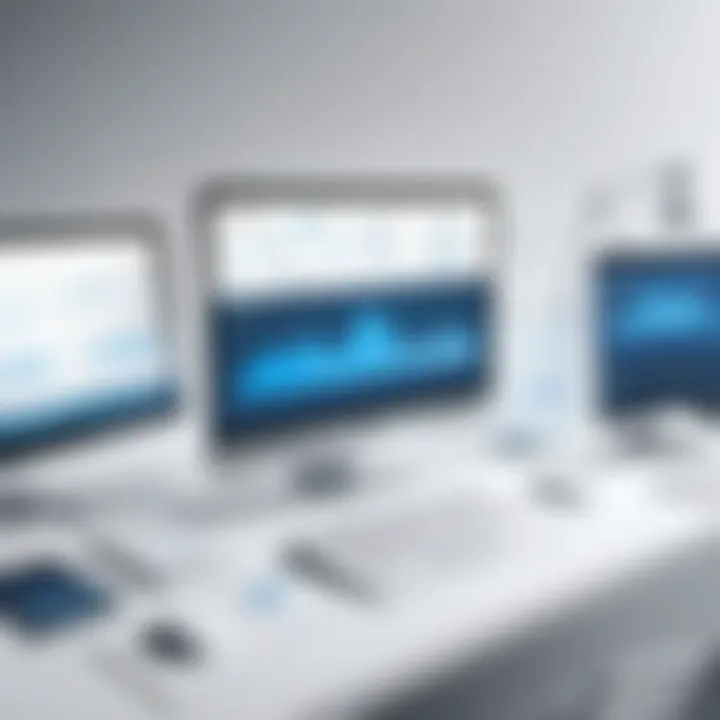
Consider the following practices:
- Role-Based Access Control (RBAC): Implementing RBAC allows companies to limit data access based on user roles, ensuring that only authorized personnel can access sensitive information.
- Encryption: Certain classes of data should be encrypted, both at rest and in transit. This provides an additional layer of security that makes it extremely difficult for unauthorized users to decipher the data, even if they gain access to it.
- Sensitive Data Discovery Tools: Automated tools help in identifying and tagging sensitive information, significantly reducing manual errors that could potentially expose sensitive data.
In summary, the interplay between data classification and cloud security is substantial. Classified data leads to meaningful insights, efficient resource allocation, and greater overall security posture. By implementing a solidified data classification framework, organizations can not only mitigate risks but also thrive in an increasingly data-driven world.
"A strong data classification approach acts as the shield protecting sensitive information, creating a resilient defense against ever-evolving threats."
Organizations must recognize that cloud readiness is not solely about moving data; it’s about understanding and managing that data intelligently.
Future Trends in Data Classification
In an age where data is ceaselessly proliferating, understanding future trends in data classification not only keeps organizations ahead of the curve but also ensures they're insulating themselves against potential threats. The evolving landscape of technology demands that data classification methods evolve too, adapting to new challenges and opportunities presented by the digital age. This isn’t simply about keeping pace; it’s about strategically positioning oneself to leverage the latest advancements to mitigate risks and boost efficiencies.
Advancements in Machine Learning for Classification
Machine Learning (ML) is paving new avenues for data classification that were previously unthinkable. Traditional methods often relied heavily on manual sorting, leaving room for error and inconsistencies. However, with the burgeoning capabilities of ML algorithms, organizations can automate data classification with remarkable efficiency.
This automation means classifying vast amounts of data with pinpoint accuracy and in real-time, thereby reducing human error significantly. ML not only speeds up the process but enhances the capability to analyze patterns within data that may be too complex for manual evaluation.
For example, an e-commerce company might use ML to identify sensitive customer data by training a model to recognize patterns related to payment information and personal identification numbers. These algorithms learn from data that is labeled over time, adapting with each interaction. It is akin to teaching a pet new tricks—it requires patience, but once learned, the benefits are substantial. Moreover, ML can continuously refine its approaches, adapting to changes in data trends or regulations without needing an overhaul of existing frameworks.
"The ability of machine learning to identify and group data dynamically cannot be understated; it allows businesses to become more agile in their data management strategies."
Integrating Data Classification with Emerging Technologies
As technology evolves, so too should our methods for integrating these advancements into our data classification frameworks. The seamless integration of classification processes with emerging technologies, such as blockchain, Internet of Things (IoT), and artificial intelligence (AI), enhances data security and management.
For instance, blockchain offers a decentralized method for tracking data classification. This means changes made to categorized data can be recorded in a tamper-proof manner, ensuring integrity and transparency. Organizations leveraging IoT can automatically classify and manage data generated from smart devices, mitigating the risk of overlooking sensitive information.
Furthermore, AI can help predict which types of data will require reclassification as the organization scales and evolves. By looking at current usage trends, AI can inform how data should be categorized in the future, making the process less reactive and more proactive. This adaptive approach minimizes downtime and enhances the overall efficiency of data management practices.
Case Studies of Effective Data Classification
Understanding how data classification functions in real-world situations provides invaluable lessons for organizations contemplating their own cloud readiness. Case studies offer a practical lens through which the theories of data classification can be evaluated, allowing us to highlight both success and failure. Embracing lessons from these situations influences organizational growth, reduces risks, and enhances overall data management strategies. This section serves to illuminate how various industries have tackled data classification challenges, illustrating the importance of applied knowledge and adaptive strategies in a rapidly evolving digital landscape.
Success Stories from Industry Leaders
In the realm of data classification, case studies often reveal telltale signs of best practices and effective approaches that organizations have taken to streamline their operations. Let’s delve into some noteworthy instances:
- Healthcare Innovations: A prominent healthcare provider utilized advanced data classification tools to sort through patient records. With patient data categorized into sensitive, restricted, and public categories, they effectively minimized the risk of breaches. The outcome was a significant reduction in data loss incidents, ultimately saving them from regulatory fines and improving patient trust.
- Financial Services Transformation: One global bank restructured its data classification system after experiencing a data breach. By implementing a multi-tiered classification framework based on data sensitivity and regulatory requirements, the bank achieved a dramatic improvement in its security posture. Consequently, they noticed a 40% decrease in data-related incidents within the first year.
- Retail Sector Dynamics: A major e-commerce platform transformed its approach to user data privacy. By categorizing customer information as personal, transactional, or analytics, they managed to enhance user targeted marketing campaigns while ensuring compliance with GDPR. The dual benefit of promoting service efficiency and mitigating compliance risks reaped substantial financial rewards for their business.
Much can be learned from these success stories. It’s apparent that when organizations prioritize a robust data classification strategy, they not only protect their assets but also unlock potential avenues for increased efficiency and compliance responsiveness.
Lessons Learned from Classification Failures
On the flip side, data classification can also serve as a cautionary tale when mishandled. Here are some lessons highlighted by organizations that faced setbacks:
- Inadequate Training and Awareness: A notable technology firm suffered severe data leaks due to employees not understanding classification protocols. Misclassified data was routinely sent to the wrong recipients. The incident emphasized the vital role of training and policy comprehension. Organizations learned that without a strong foundation in rules and responsibilities, even the most sophisticated systems could fail.
- Static Classification Systems: An energy company discovered that their static classification system could not accommodate the rapid influx of new data streams and varied data types. This rigidity led to numerous inconsistencies in data handling, causing workflow nightmares during audits. Organizations realized that adaptability in data classification processes is pivotal in meeting the dynamic needs of changing data landscapes.
- Neglecting Ongoing Assessments: A governmental agency faced backlash after its data classification system became outdated, exposing sensitive information. Without regular assessments, existing classifications failed to reflect the current state of information. The moral here was clear: Regular reviews of classification schemes are crucial to maintain security and compliance.
The journey through data classification is fraught with opportunities for both success and failure. Yet, through careful case study analysis, one can derive actionable insights to enhance organizational preparedness and resilience in cloud environments.
“Learning from the past is not just wise; it is essential for sustainable growth.”
In summary, these cases underline the necessity of thoughtful, tailor-made data classification strategies within organizations. They reveal that both triumphs and missteps, if scrutinized and understood, can shape responsive and progressive classification practices that ultimately lead to enhanced cloud readiness.
Epilogue and Key Takeaways
In the rapidly evolving landscape of cloud technology, the intricate art of data classification emerges as a cornerstone for achieving cloud readiness. As organizations transition to cloud-based infrastructures, they encounter an ocean of information that must be accurately categorized and protected. This segment wraps up the pivotal discussions held in this article, his elaborating on the necessity of data classification to improve compliance, bolster security, and optimize resource allocation. By systematically identifying and managing data, businesses can effectively navigate legal landscapes while preserving the integrity of sensitive information.
Summarizing the Value of Data Classification
Data classification isn't just a technical process; it’s a strategic imperative that offers myriad benefits. First, it provides a structured approach to understanding what data resides within an organization. Recognizing the varying sensitivity levels of data allows organizations to apply appropriate security measures. Furthermore, it aids in regulatory compliance, ensuring that businesses meet the demands laid down by governing bodies such as GDPR or HIPAA. Failing to classify and protect data can have dire consequences, including hefty fines or irreversible reputational damage.
Additionally, analysts and IT teams can streamline their data management processes, making it easier to discover and retrieve relevant information when needed. For example, a marketing team might classify customer data into segments—such as engagement level or purchasing history—leading to more targeted and effective campaigns. This signifies just how data classification can transform raw data into a valuable asset, driving informed decisions.
"The best way to predict the future is to create it." - Peter Drucker
Encouraging Continuous Improvement
In the realm of data classification, stagnation is not an option. Continuous improvement is vital; as the technology landscape changes and threats evolve, organizations must routinely revisit and refine their classification processes. This means keeping up with emerging technologies, such as machine learning and automation, which can drastically enhance classification capabilities.
Regular assessments should be conducted to evaluate both the methodology and effectiveness of data classification strategies. Are they still relevant? Are they yielding the intended results? Companies might find it beneficial to incorporate feedback from various sectors within the organization, fostering a culture that prioritizes data stewardship.
Moreover, engaging in ongoing training for staff regarding data classification policies can enhance overall awareness and buy-in. An informed workforce is instrumental in maintaining secure and compliant data practices, thus creating a robust security posture.