Understanding Data Governance: Risks and Controls
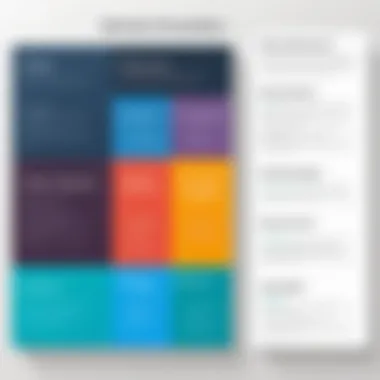
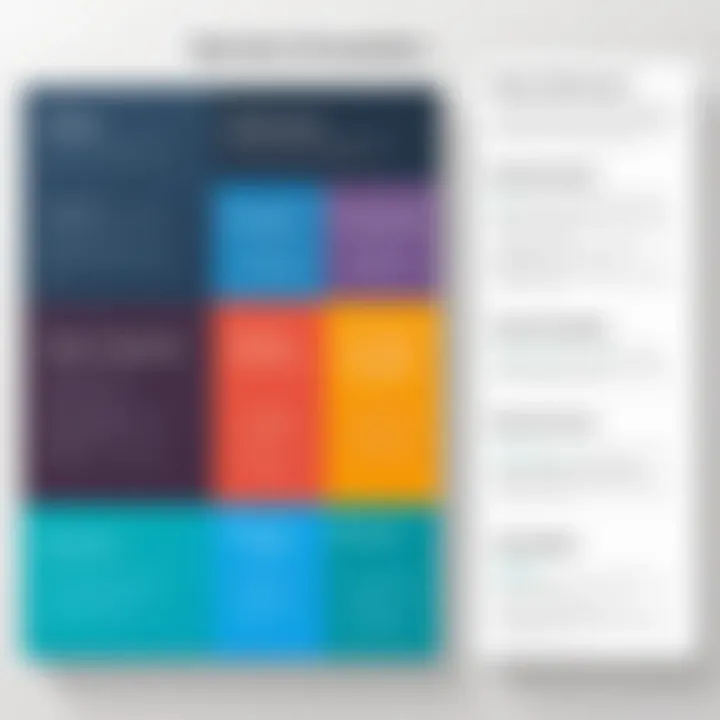
Intro
Data governance plays a critical role in today's digital ecosystem. As organizations increasingly rely on data to inform their decisions, the need for comprehensive governance frameworks becomes more urgent. The risks associated with mismanaged data can be profound, affecting not only internal operations but also external stakeholder trust and regulatory compliance.
The significance of data governance cannot be overstated. In an environment where data breaches and cybersecurity threats are ever-present, it is essential for businesses to implement strong governance measures. These measures serve to bolster data integrity, ensuring that data is accurate, available, and secure. The following sections will discuss the key components of data governance, categorize the various risks, and propose effective strategies for mitigating these risks through robust controls.
Understanding Data Governance Frameworks
A data governance framework encompasses policies, standards, and practices designed to ensure effective data management. This framework defines who can access what information and under what circumstances. It delineates roles and responsibilities, ensuring accountability while promoting data quality and security.
Importance of a Governance Framework
Organizations without proper governance expose themselves to multiple risks:
- Data quality issues: Poor data governance often leads to data quality challenges.
- Compliance failures: Regulatory compliance matters require well-established data governance.
- Operational inefficiencies: Without clear guidelines, data management can become chaotic.
Categories of Data Risks
Understanding the risks associated with data is vital for any organization. These risks can be broadly categorized as follows:
- Compliance Risks: Non-compliance with regulations can lead to severe penalties.
- Security Risks: Data breaches pose significant threats to organizational integrity.
- Operational Risks: Failure in data processes can disrupt business continuity.
- Reputational Risks: Public perception can be damaged through mismanagement of data.
Effective Controls for Data Management
To manage these risks effectively, organizations must implement controls that include:
- Data Classification: Identifying and classifying data based on sensitivity and importance.
- Access Controls: Limiting data access to authorized personnel only.
- Monitoring and Auditing: Regular reviews to ensure compliance with policies.
- Training and Awareness: Engaging employees with best practices related to data management.
"Effective data governance is not a one-time project but an ongoing process that requires dedication and investment."
End
Prologue to Data Governance
In today's data-driven landscape, the concept of data governance has emerged as a linchpin for many organizations. Data governance encapsulates a myriad of practices, standards, and policies that help manage the availability, usability, integrity, and security of data used in an organization. Understanding this area is crucial as organizations strive to make informed decisions based on accurate and reliable information. The necessity of data governance becomes even more pronounced when considering the risks associated with poor data management.
By implementing robust data governance strategies, organizations can mitigate risks, enhance compliance, and boost overall data quality. Furthermore, it aids in establishing clear data ownership and accountability. This clarity not only protects the organization against potential legal repercussions but also fosters a culture of responsible data use.
As we delve into the nuances of data governance, the focus will be on defining the core components of the practice and understanding its significance in the modern digital ecosystem.
Defining Data Governance
Data governance is fundamentally about the management of data assets. It involves a structured framework whereby policies, procedures, and standards are put in place to ensure that data is accurate, available, and secure.
It encompasses not just the technology that manages data but also the people responsible for data stewardship. This collaborative effort ensures that data remains trustworthy and aligned with organizational goals.
A key element of effective data governance is establishing clear roles and responsibilities for data management. This often involves identifying data stewards or custodians who will oversee data lifecycle processes, including creation, storage, access, and deletion.
Importance in the Digital Age
The importance of data governance has escalated in the digital era, where data serves as a primary asset for decision-making. As organizations increasingly rely on data analytics to drive strategies, the integrity and security of this data become paramount.
The confluence of technology and regulatory demands makes it essential for organizations to adopt robust governance measures. For example, risks such as data breaches and non-compliance with regulations such as the General Data Protection Regulation (GDPR) and California Consumer Privacy Act (CCPA) pose significant threats.
Each of these factors underscores the value of having a solid data governance framework. The framework not only safeguards data but also enhances trust and transparency among stakeholders.
"In an age where data is considered new oil, its effective governance will dictate the success or failure of organizations."
Embracing data governance is no longer optional. It has transitioned into a necessity for maintaining competitive advantages and ensuring operational efficiency.
The Landscape of Data Governance Risks
The landscape of data governance risks plays a critical role in the overall data management strategy of organizations. Understanding these risks is essential for ensuring that data is accurate, secure, and compliant with relevant regulations. Recognizing the various types of risks allows organizations to prioritize their efforts in creating a robust data governance framework. This section will discuss the specific categories of data governance risks, their implications, and the importance of addressing them effectively.
Types of Data Governance Risks
Data governance risks can be categorized into three main types: data security risks, compliance risks, and operational risks. Each type presents unique challenges that organizations must address to protect their data assets.
Data Security Risks
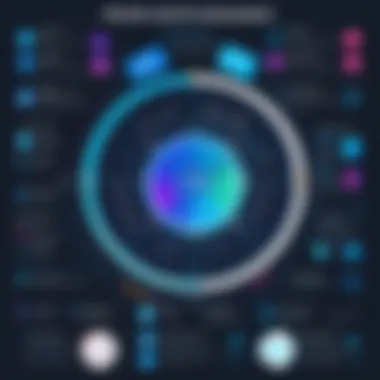
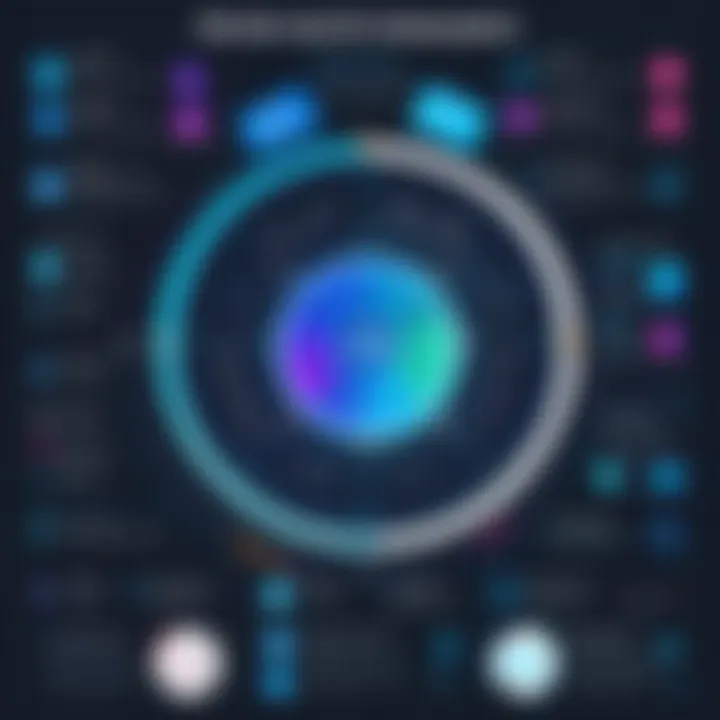
Data security risks refer to the potential threats that may lead to unauthorized access, loss, or corruption of sensitive information. These risks are increasingly significant in the digital landscape, as cyberattacks and data breaches become more prevalent. A key characteristic of data security risks is their ability to compromise confidential data, leading to financial losses and damage to reputation. This aspect makes it a crucial focus area in this article.
The unique feature of data security risks is that they can often originate from both internal and external sources. They may arise from malicious actors, technical vulnerabilities, or human errors. The advantages of addressing data security risks include enhanced protection of sensitive information, greater compliance with industry standards, and increased trust from stakeholders. However, the disadvantages may involve high costs associated with implementing security measures and the continuous need for monitoring and updating security protocols.
Compliance Risks
Compliance risks entail the potential for failing to adhere to laws and regulations that govern data usage and management. Organizations are required to follow various regulations, such as the General Data Protection Regulation (GDPR) and the California Consumer Privacy Act (CCPA). A key characteristic of compliance risks is their legal implications, as non-compliance can lead to hefty fines and legal actions. This makes it a significant aspect of this article.
The unique feature of compliance risks is that they can affect an organizationโs operational processes. Achieving compliance requires organizations to have clear policies and procedures in place. Advantages of effective compliance risk management include avoiding penalties, improving operational efficiency, and fostering a positive corporate image. On the other hand, the challenges may involve the complexity of regulations and the resource requirements for maintaining compliance.
Operational Risks
Operational risks pertain to the risks that arise from inadequate or failed internal processes, people, and systems. These risks can significantly impact day-to-day operations and undermine the effectiveness of data governance strategies. A key characteristic of operational risks is their direct influence on the organization's capacity to effectively manage data. This aspect further highlights its relevance to this article.
The unique feature of operational risks is that they can be mitigated through proper training, process improvements, and technology upgrades. Strong risk management practices can lead to improved data quality and more efficient processes. Advantages of managing operational risks include smoother operations, reduced downtime, and increased organizational resilience. However, the drawbacks may include a reactive stance rather than a proactive one, leading to potential operational interruptions.
Identifying Potential Threats
Identifying potential threats is a crucial component of understanding the risks in data governance. Organizations must conduct thorough assessments to uncover vulnerabilities and threats that can jeopardize their data governance efforts. This proactive approach fosters better preparedness and resilience against potential data violations and losses. By prioritizing the identification of these threats, organizations can develop tailored strategies that address their specific risk landscape.
Frameworks for Data Governance
In the realm of data governance, frameworks serve as essential blueprints that guide organizations in managing their data more effectively. They provide structure and consist of essential concepts that align data management with business objectives. By establishing a clear framework, organizations can address the complexities of data governance while mitigating associated risks. The emphasis here is on ensuring that data integrity, quality, and security are maintained consistently across the organization. Without such a framework, organizations risk falling into chaos, where data becomes unreliable, thereby hindering decision-making processes.
Key Components of a Governance Framework
Policies and Procedures
Policies and procedures are the backbone of any governance framework. They set the standards for data management practices and serve as a reference point for organizational behavior regarding data usage. A prominent characteristic of policies is that they offer clear guidelines that all employees must follow, promoting accountability and transparency. This consistency makes them a popular choice in data governance.
One unique feature of policies is their ability to adapt to compliance regulations. For instance, organizations often develop policies to align with GDPR or CCPA requirements, which enhances their legal standing. However, it's also essential to recognize that poorly defined or overly complex policies can lead to misunderstandings and non-compliance.
Roles and Responsibilities
Clearly defined roles and responsibilities ensure that everyone within the organization understands their obligations concerning data governance. This aspect is crucial because a lack of defined roles can lead to gaps in accountability, making it easier for data mishandling to occur. A critical characteristic of defining roles is the clarity it provides. Employees know who to approach for specific issues, making problem resolution more effective.
The concept of role definition often includes the designation of data stewards and compliance officers, who become champions of data governance within the organization. This creates a systematic approach that often proves beneficial for organizational memory and data integrity. However, it must be noted that if roles are not implemented properly, they can result in confusion and overlaps in responsibilities, which can complicate governance efforts.
Data Stewardship
Data stewardship focuses on the management and oversight of data assets, ensuring they are accurately maintained and protected. A key characteristic of data stewardship is that it promotes a culture of responsible data usage. By involving individuals whose sole responsibility is to uphold data quality and compliance, organizations can achieve a deeper level of governance.
The unique feature of data stewardship lies in its proactive approach. Data stewards actively work to monitor data quality and address data issues before they escalate. This responsibility can help prevent data breaches and ensure that data remains a reliable asset for the organization. However, implementing data stewardship can be resource-intensive, which may discourage smaller organizations from adopting a comprehensive stewardship program.
Best Practices in Governance Framework Design
When designing a governance framework, certain best practices can enhance its efficacy:
- Involvement of Stakeholders: Engage various departments from the onset to capture a broad perspective on data needs and issues.
- Regular Reviews and Updates: Establish mechanisms for continuous review and adjustment of policies and procedures in light of changing regulations or organizational dynamics.
- Comprehensive Training: Train employees on data governance policies and the importance of compliance to facilitate adherence and understanding.
Ultimately, a well-defined framework acts as the scaffold that supports a data governance program, ensuring that risks are mitigated while leveraging the value from data.
Mitigating Data Governance Risks
Mitigating data governance risks is a critical facet of modern data management strategies. As organizations increasingly rely on data for decision-making, the need for effective control measures becomes paramount. Data governance risks can stem from various sources such as technical failures, human errors, and evolving regulations. Implementing a robust framework for mitigating these risks helps to ensure data integrity and compliance, ultimately safeguarding organizational reputation and operational viability.
Implementing Effective Controls
Data Classification
Data classification is a foundational step in mitigating data governance risks. By categorizing data based on sensitivity and importance, organizations can better manage their information assets. This practice enables the prioritization of security measures and access controls according to the risk level associated with each data type.
A key characteristic of data classification is that it provides a systematic approach to data management. This systematic nature makes it a beneficial choice in the context of this article. Properly executed, data classification can significantly enhance an organization's ability to protect sensitive information and comply with various regulatory requirements.
One unique feature of data classification is its ability to facilitate data discovery. Advantages include reducing the risk of unauthorized access and ensuring compliance with laws such as GDPR and CCPA. However, over-reliance on automated classification tools can sometimes lead to misclassification, which poses a potential disadvantage.
Access Controls
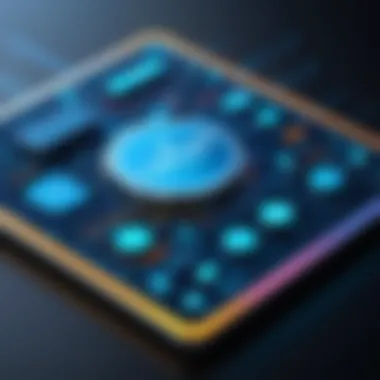
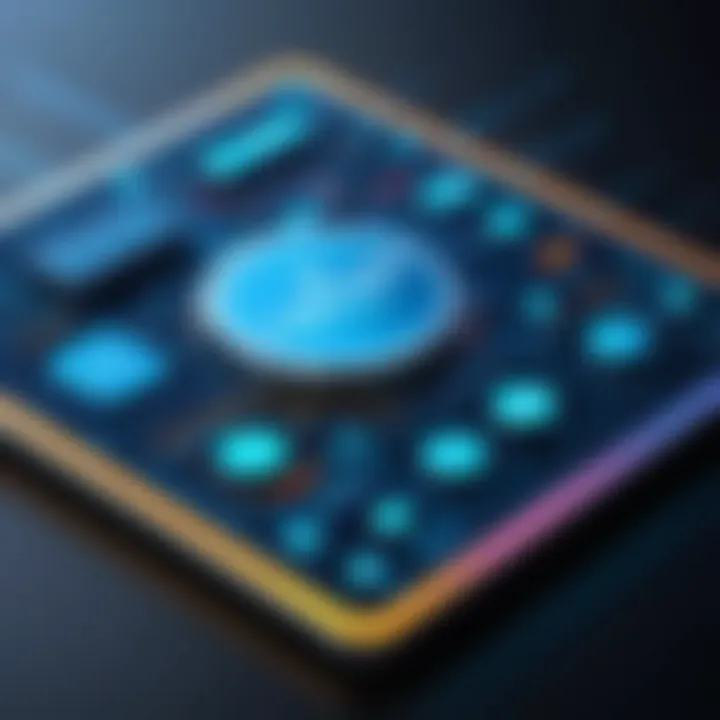
Access controls play a pivotal role in the broader scope of data governance risk mitigation. By defining who can view or manipulate sensitive data, organizations can proactively prevent unauthorized access. The effectiveness of access controls lies in their ability to enforce policies that align with data classification.
A notable key characteristic of access controls is the concept of least privilege. This principle restricts data access to only those individuals who need it for their work. This makes access controls a popular choice for reinforcing data security in the context of this article.
The unique feature of access controls is their adaptability. Organizations can implement various types of access controls, including role-based access and discretionary access models tailored to their specific needs. The advantages of these controls include reduced risk of insider threats, while the disadvantages can be the complexity in management and potential challenges in balancing user access with security.
Monitoring and Audit Trails
Monitoring and audit trails are essential components of an effective data governance strategy. They provide visibility into how data is accessed and used within an organization. This transparency is crucial for detecting and responding to potential threats quickly.
A prominent characteristic of monitoring and audit trails is that they create a historical record of data access and modifications. This capability makes it a beneficial choice for organizations seeking to ensure compliance with strict regulations while managing risks effectively.
The unique feature of monitoring systems is their real-time analysis capability. This allows for prompt responses to suspicious activities, which can significantly enhance data security. Advantages include the ability to deter misconduct and track data lineage, while disadvantages might include the storage and management challenges posed by the accumulation of large volumes of logs.
Training and Awareness Programs
Implementing training and awareness programs is essential for supporting data governance efforts. These programs equip employees with the knowledge necessary to understand the significance of data policies and the implications of their actions regarding data management.
Such initiatives not only promote a culture of security but also reduce human error, which is a leading cause of data breaches. Organizations that invest in training can expect a more informed workforce that is aligned with their data governance objectives.
Compliance and Regulatory Considerations
Compliance and regulatory considerations are crucial to effective data governance. Organizations today work in an environment shaped by a myriad of regulations that govern data usage, privacy, and security. Understanding these regulations informs organizations how to handle data responsibly and mitigate legal risks. By aligning data governance strategies with compliance frameworks, organizations can not only stay within legal boundaries but also build trust with customers. Compliance fosters data integrity, ensuring that data is accurate, accessible, and appropriately managed.
Understanding Regulatory Frameworks
GDPR
The General Data Protection Regulation, commonly known as GDPR, is a regulation in EU law. It focuses on data protection and privacy for individuals within the European Union and the European Economic Area. One key characteristic of GDPR is the emphasis it places on data subject rights. It grants individuals significant control over their personal data and mandates organizations to explicitly obtain consent when collecting data. This becomes beneficial for organizations aiming to establish transparency. A unique feature of GDPR is the accountability principle which requires organizations to demonstrate compliance.
Advantages of GDPR include heightened consumer trust and competitive advantage in data privacy. However, its strict fines for non-compliance can be a challenge for smaller entities lacking resources.
CCPA
The California Consumer Privacy Act, or CCPA, enhances privacy rights for residents of California. It is notable for its broad definition of personal information, encompassing various data points that organizations may collect. One of the primary benefits of CCPA is that it allows consumers rights to know what data is collected, to whom it is sold, and the ability to opt-out of data selling. The unique feature of CCPA is its focus on providing consumers with a clear choice regarding their data.
On the downside, businesses may face difficulties in adjusting to CCPA requirements, particularly if they operate in multiple jurisdictions. Additionally, the regulations are still evolving, adding complexity to compliance.
HIPAA
The Health Insurance Portability and Accountability Act, or HIPAA, sets the standard for protecting sensitive patient data in the healthcare industry. A significant aspect of HIPAA is its Privacy Rule, which protects individually identifiable health information. This characteristic makes HIPAA a vital choice for organizations in healthcare. A unique feature of HIPAA is its Security Rule, which mandates safeguarding electronic protected health information (ePHI) through various administrative, physical, and technical safeguards.
While HIPAA ensures stringent data protection, its complexity can overwhelm smaller healthcare providers, potentially leading to compliance challenges.
Integrating Compliance into Governance
Integrating compliance into data governance allows organizations to align their data strategy with regulatory requirements effectively. It requires creating policies that not only meet compliance standards but also promote ethical data handling practices. Regular audits and assessments ensure that data governance frameworks stay relevant and effective. Moreover, the involvement of all stakeholders, including executives and IT teams, is key to fostering a culture of compliance.
"Organizations must view compliance not just as a legal necessity but as a strategic advantage in building trust and credibility with their stakeholders."
The Role of Technology in Data Governance
In the context of data governance, technology acts as a cornerstone that supports and enhances various governance strategies. The rise of technology not only simplifies the management of data but also plays a vital role in ensuring security, compliance, and the overall efficiency of processes. Organizations increasingly turn to technological solutions to navigate the complexities of data governance, as they address both inherent risks and operational challenges.
Technology enables the implementation of structured frameworks that facilitate data integrity and reliability. By leveraging specific tools, organizations can better manage data throughout its lifecycle, ensuring that it remains accurate, secure, and compliant with applicable regulations. The evolving landscape of data management requires these technology solutions to adapt rapidly; therefore, organizations must choose the right tools that can integrate seamlessly into their governance strategies.
Data Management Tools
Data Catalogs
Data catalogs function as a central repository where all data assets are organized and managed efficiently. They provide a comprehensive overview of the data landscape within an organization, making it easier to locate, access, and utilize data. A key characteristic of data catalogs is their ability to tag and classify data assets, streamlining the search process. This characteristic makes data catalogs a beneficial choice for organizations aiming to enhance collaboration and maintain data transparency.
The unique feature of data catalogs is the user-friendly interface that allows non-technical stakeholders to engage with data. By simplifying data discovery, organizations can empower more users to utilize data for decision-making, ultimately promoting a data-driven culture. However, implementing a data catalog may require initial investment and ongoing maintenance, which can be viewed as a disadvantage for some organizations.
Metadata Management
Metadata management encompasses the processes of handling data that describes other data. This aspect is crucial for ensuring that data is understandable, consistent, and reliable. A key characteristic of metadata management systems is their capability to maintain a connection among various data entities, helping organizations track data lineage and provenance effectively.
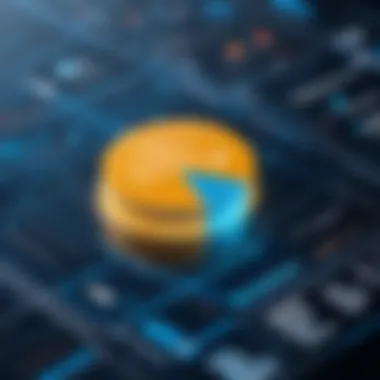
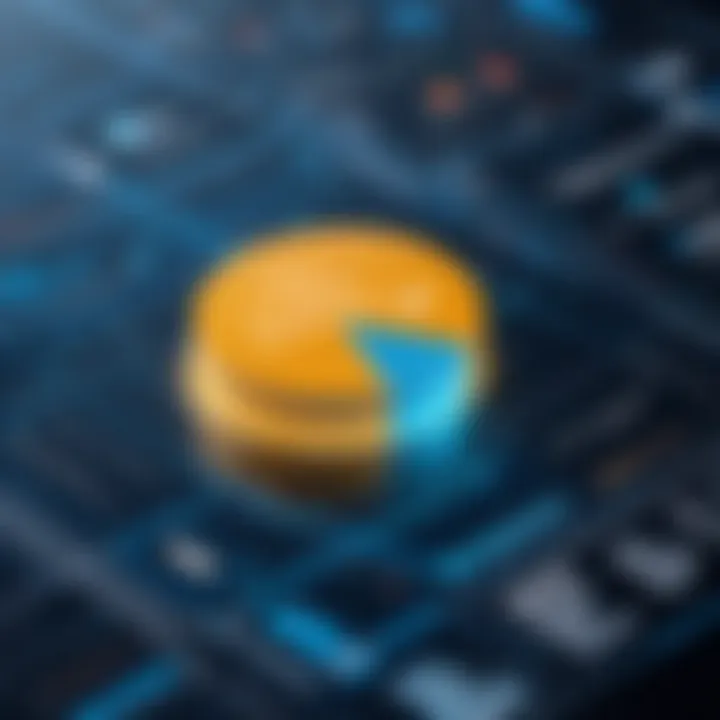
The unique feature of metadata management systems lies in their ability to facilitate data governance by providing context. With proper metadata, organizations can enforce policies and standards more effectively. However, a potential disadvantage is the complexity that arises in managing extensive metadata, which may overwhelm some teams if not structured properly.
Data Quality Tools
Data quality tools focus on preserving the accuracy and usability of data by identifying and rectifying errors. A notable feature of these tools is their capacity for automated data cleansing, which saves time and reduces manual intervention. This makes data quality tools a popular choice for organizations seeking to maintain high data standards.
The unique aspect of data quality tools is the ability to perform continuous monitoring and validation of data records. This constant oversight can drastically improve overall data reliability. However, some users might find these tools to be costly or complicated to implement, especially if systems are not properly aligned with organizational needs.
Automation in Governance Processes
Automation serves as a pivotal element in enhancing the efficiency of governance processes. By automating repetitive tasks related to data management, organizations can minimize human error and free up valuable resources. This can lead to significant improvements in compliance adherence, data discovery, and reporting accuracy.
Automation also allows organizations to respond quickly to changes in regulatory requirements, ensuring that compliance demands are met promptly. Furthermore, automated workflows facilitate consistency across processes, allowing organizations to establish standard operating procedures. Although automation presents various benefits, it is essential to ensure that the underlying systems are designed correctly to mitigate potential risks, such as over-reliance on automated processes.
Challenges in Data Governance Implementation
Data governance is a vital aspect of modern data management strategies. However, implementing an effective data governance framework is fraught with challenges. Understanding these challenges is essential for organizations to develop a resilient strategy in the face of potential data-related threats. This section will delve into two significant challenges: resistance to change and scalability issues.
Resistance to Change
One of the primary challenges facing organizations when establishing data governance is resistance to change. Employees often have established workflows and may feel threatened by new policies or systems. This resistance is not merely a reluctance to adapt; it is often rooted in uncertainty about how the change will impact individual roles and responsibilities.
Organizations need to manage this resistance proactively. Effective communication about the reasons for change can help alleviate concerns. Employees must understand the benefits of data governance, such as enhanced data quality and improved security. Leadership should also involve team members in the governance process, promoting a sense of ownership and commitment. Workshops and training sessions can be instrumental in easing the transition, thus reducing resistance over time.
Scalability Issues
Another critical challenge in data governance implementation is scalability. As organizations grow, their data management needs become more complex. A governance framework that works well for a small team may not be sustainable as the organization expands. The challenge lies in creating a scalable solution that can adapt to increasing data volumes without compromising governance standards.
Organizations should consider the following strategies to address scalability issues:
- Modular Framework Design: Incorporating flexibility in governance structures can facilitate adjustments as data needs evolve.
- Technological Integration: Leveraging automation and advanced tools can streamline governance processes and enhance scalability.
- Regular Review: Implementing a schedule for regular reviews of governance policies ensures alignment with organizational growth.
"A proactive approach to managing resistance and planning for scalability can significantly enhance the effectiveness of data governance initiatives."
Case Studies in Data Governance
Analyzing case studies in data governance offers valuable insights into real-world applications. These case studies illustrate how organizations have successfully implemented data governance frameworks and how they have learned from their missteps. Understanding these practical examples helps cybersecurity professionals, IT specialists, and other stakeholders grasp the complexities of data management and governance.
Successful Implementations
Successful implementations of data governance highlight the beneficial outcomes of establishing robust frameworks. For example, a healthcare organization may implement rigorous data governance policies to enhance patient privacy and compliance with health regulations. By assigning data stewardship roles and utilizing data classification techniques, they ensure that sensitive information is managed properly.
Such cases often demonstrate that aligning data governance strategies with business objectives is critical. Benefits include improved decision-making capabilities, increased operational efficiency, and reduced risk of compliance violations. Some key elements of successful implementations are:
- Clear Leadership: Effective governance starts at the top. Engaging executive leaders ensures that data governance receives the necessary attention and resources.
- Inclusiveness: Bringing diverse teams together can foster better practices, inviting perspectives from various departments.
- Continuous Assessment: Regular audits and assessments allow organizations to refine their governance processes over time, adapting to new challenges and risks.
Lessons from Failures
While successful implementations provide guidance, failures present essential teachings. Even large corporations struggle with governance challenges, leading to significant repercussions. A notable case was a tech giant that faced data breaches due to inadequate access controls and failure to comply with data protection regulations. The fallout included financial loss, reputational damage, and legal scrutiny.
Key lessons learned from failures in data governance include:
- Inadequate Planning: Insufficient frameworks often lead to poor execution. Organizations should develop comprehensive plans that outline not just policies but also the resources needed for implementation.
- Neglecting Employee Training: Without ongoing training, even the best policies can falter. Employees must understand their roles within the governance framework to prevent inadvertent breaches.
- Ignoring Regulatory Changes: Organizations must actively keep up with changes in regulations to ensure compliance. A failure to adapt can lead to hefty fines and sanctions.
By examining these successes and failures, stakeholders can draw actionable insights to enhance their own governance strategies. This reflective approach fosters a deeper understanding of data governance, providing meaningful directions for professionals in the field.
Future Trends in Data Governance
Future trends in data governance are shaped by a constantly changing technological landscape and evolving compliance requirements. Understanding these trends is vital for organizations striving to enhance their data strategy while mitigating risks associated with data management. As professionals in this space navigate through issues like data integrity, security, and compliance, recognition of these future trends will help in crafting proactive strategies.
Evolving Governance Models
Evolving governance models reflect an adaptation to the complexities of modern data environments. Traditional top-down governance approaches struggle to keep pace with the dynamic nature of data usage in organizations today. Instead, organizations are leaning toward models that encourage collaboration among cross-functional teams. These models empower data owners, stewards, and users, allowing for greater flexibility and responsiveness.
Moreover, these models integrate aspects like agility and scalability, which address the varied data needs across departments. This shift encourages a more decentralized approach to governance, enabling units within an organization to tailor their governance strategies according to specific contexts. By fostering collaboration, organizations can enhance accountability and improve data quality, which are essential for data-driven decisions.
The Impact of AI and Machine Learning
The integration of artificial intelligence (AI) and machine learning (ML) in data governance demonstrates significant potential. AI and ML technologies can streamline data discovery and classification, making it easier to manage vast amounts of data. Automated processes reduce human error and enhance the efficiency of data governance initiatives.
For instance, AI can help in identifying patterns and anomalies within data, aiding in risk assessment. Tools employing these technologies can also automate compliance checks, making it easier to adhere to regulations like GDPR and CCPA.
However, leveraging AI and ML also presents challenges. The accuracy of these automated processes depends on the quality of the underlying data. Also, ethical considerations around AI usage must be taken into account, especially concerning data privacy and transparency.