Understanding AIOps: Transforming IT Operations Management
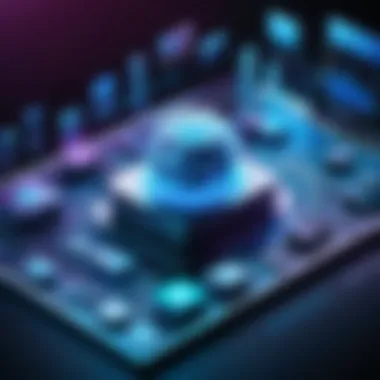
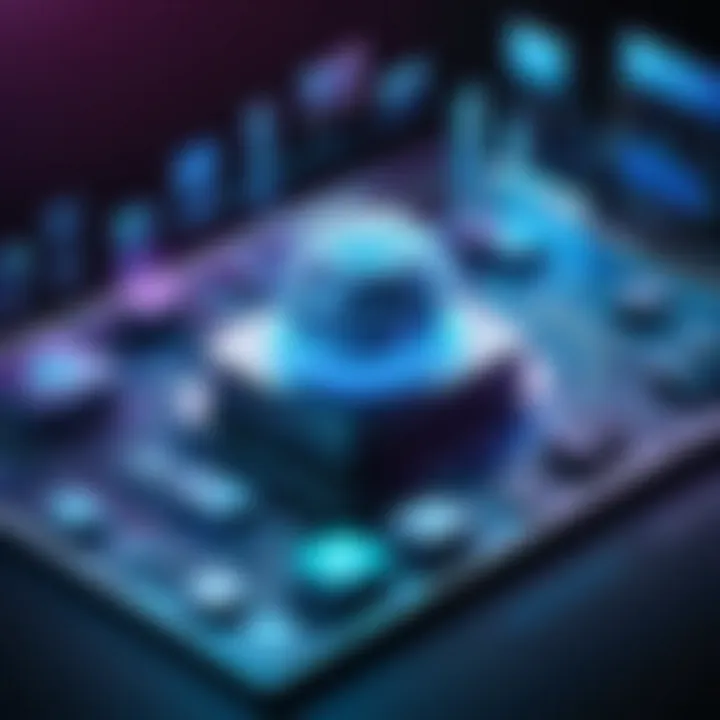
Intro
AIOps, short for Artificial Intelligence for IT Operations, represents a pivotal evolution in the management of IT operations. In an age where technology drives business processes, AIOps utilizes advanced technologies, including artificial intelligence and machine learning, to address complex operational challenges. This section will explore AIOps, discussing its importance, its benefits, and the pressing need for IT professionals to adapt to this paradigm shift within their organizations.
Understanding AIOps
AIOps is characterized by the integration of analytics and machine learning into IT operations tools. This amalgamation enables automation, helping teams identify and respond to incidents more efficiently. The significance of AIOps cannot be underestimated, as it directly contributes to minimizing downtime and enhancing service delivery.
The Technological Foundation of AIOps
To fully grasp AIOps, it is crucial to understand the technologies that power it. The core technologies include:
- Artificial Intelligence: Helps in analyzing large sets of data to predict and detect issues.
- Machine Learning: Learns from past incidents to improve response times.
- Big Data: Affects the scale and speed with which data is processed, making it integral to IT operations.
Together, these technologies create a robust framework that supports smarter decision-making and more proactive incident management.
Benefits of AIOps
Adopting AIOps can yield several benefits for organizations:
- Increased Efficiency: Automates routine tasks, allowing teams to focus on more strategic initiatives.
- Improved Incident Response: Enables quicker identification of incidents, reducing the time to resolution.
- Enhanced Visibility: Provides real-time insights into the performance of IT operations, aiding in informed decision-making.
The potential improvements in operational efficiency through AIOps are significant and warrant consideration from IT professionals in their strategic planning.
Challenges to Consider
While AIOps offers notable advantages, some challenges still persist:
- Data Quality: Success hinges on the quality of the input data; poor data can lead to misguided analyses.
- Integration Complexity: Incorporating AIOps into existing IT environments may require significant restructuring.
- Skill Gaps: Organizations may struggle to find talent that possesses both a strong IT operations background and knowledge of AI technologies.
Real-World Applications
Many companies are already reaping the rewards of AIOps:
- Netflix utilizes AIOps for seamless streaming experiences, anticipating and responding to network congestion.
- IBM employs AIOps within its Watson product to enhance customer service through predictive insights.
These examples demonstrate the transformative impact of AIOps, suggesting a trend that is likely to become standard practice across various industries.
"As digital infrastructures evolve, embracing AIOps is not just an option; it is crucial for sustaining competitive advantage."
Ending
Understanding AIOps is essential for IT professionals striving to leverage new technologies for efficiency and effectiveness. Its integration holds the potential for substantial gains while addressing the complexities of modern IT environments. As organizations continue to depend on digital solutions, AIOps will play an increasingly vital role in shaping the future of IT operations management.
Foreword to AIOps
AIOps, or Artificial Intelligence for IT Operations, represents a transformative shift in how businesses manage their IT environments. As organizations increasingly rely on digital infrastructure, the management of IT operations becomes vital for ensuring optimal performance. This section serves as a foundation for understanding AIOps, illustrating its significance and highlighting its relevance to IT professionals.
The increasing complexity of IT systems necessitates a more refined approach to operations management. Traditional methods often struggle to keep pace with ever-growing data volumes and rapid technological advancements. AIOps integrates artificial intelligence to analyze vast datasets, providing insights that facilitate faster decision-making and more efficient operations.
In the coming sections, the discussion will delve deeper into the specific definitions and historical contexts of AIOps, as well as its core components. Understanding these underlying principles is essential for professionals aiming to enhance operational efficiency through automation and AI.
Defining AIOps
In simple terms, AIOps incorporates AI technologies into the management of IT operations. This includes various functionalities such as data ingestion, analysis, and automated incident response. Essentially, AIOps aids in filtering relevant information from a sea of data, thus enabling IT teams to make informed decisions quickly.
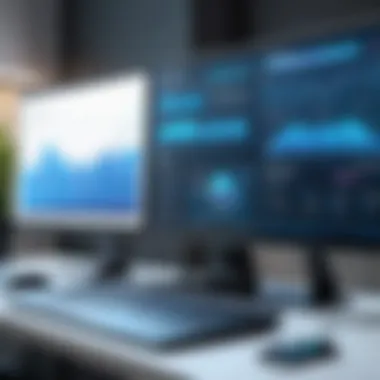
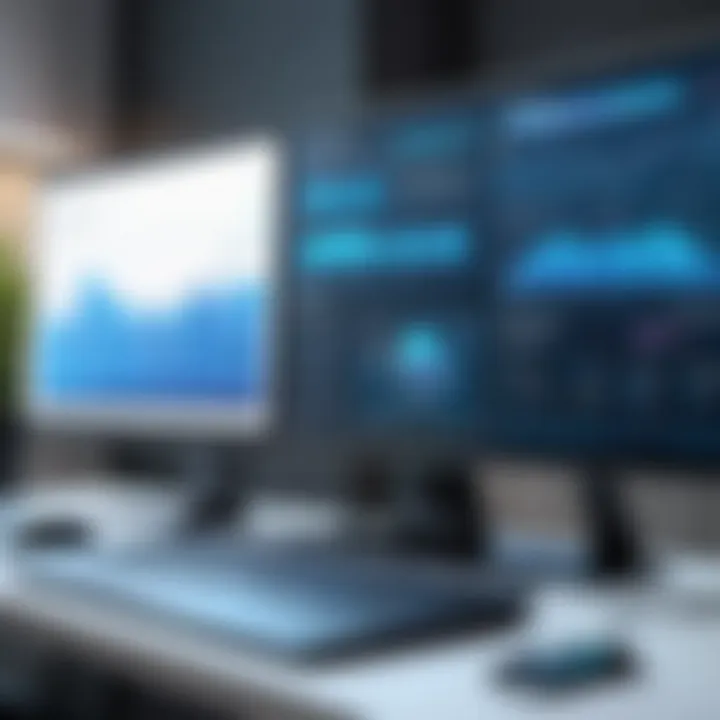
Key features of AIOps:
- Data Aggregation: Collects data from diverse sources within the IT environment.
- Real-time Analysis: Processes information in real-time, allowing for immediate insights and responses.
- Predictive Capabilities: Utilizes machine learning algorithms to predict potential issues before they escalate.
Unquestionably, AIOps represents a fundamental evolution in IT operations, shifting focus from manual processes to intelligent automation. This transition aims to reduce downtime and optimize resources, echoing a growing demand for more efficient practices in IT management.
Historical Context
Understanding the historical backdrop of AIOps provides insight into its evolution and importance today. Initially, IT operations relied on manual processes, with teams sifting through logs and alerts to identify problems. This approach was time-consuming and often resulted in delays in response times, leading to increased operational risks.
The advent of big data changed the landscape significantly. Organizations began to gather an unprecedented volume of data, which, while beneficial, also introduced new challenges. As data sources grew, the traditional IT operations model could not keep up, necessitating a new approach.
By the early 2010s, solutions started to emerge that utilized automation and machine learning techniques to better manage data overload. Companies began to implement AI technologies, paving the way for AIOps.
AIOps now stands at a pivotal juncture, addressing the demands of modern IT environments that require agility, accuracy, and efficiency. Understanding how AIOps developed from earlier models will aid professionals in leveraging its full potential.
The Role of Artificial Intelligence in IT Operations
Artificial Intelligence (AI) has reshaped many sectors, and IT operations are no exception. Incorporating AI into IT operations improves decision-making processes, automates repetitive tasks, and enhances overall efficiency. The complexity of modern IT infrastructures demands innovative approaches. AIOps leverages AI to analyze data from various sources, enabling organizations to respond swiftly to potential issues.
Impact of AI on IT Management
The integration of AI in IT management brings numerous advantages. First, it significantly reduces response times during incidents. AI can quickly identify and categorize issues, allowing teams to prioritize their responses. This speed is crucial to minimize downtime and maintain service levels, which are critical for business continuity.
Moreover, using AI diminishes the burden on IT staff. By automating routine tasks, such as system monitoring and data analysis, teams can focus on more strategic initiatives. This shift in focus is vital in todayโs fast-paced environment, where agility is essential. Furthermore, AI enables data-driven insights, which help organizations make informed decisions about resource allocation and risk management.
Additionally, AI can predict patterns and potential issues before they escalate. This proactive approach allows IT divisions to address problems before they impact users or systems. Employing predictive analytics leads to enhanced reliability in IT service management.
Machine Learning and Its Applications
Machine Learning (ML) is a critical component of AIOps. By applying algorithms and statistical models, ML enables systems to automatically learn and improve from experience without being explicitly programmed. This adaptability is vital for dealing with the dynamic nature of IT environments.
One of the primary applications of ML in AIOps is anomaly detection. Traditional methods often overlook subtle signs of trouble, but ML models excel in identifying outliers that signify potential issues. Recognizing these anomalies allows teams to investigate further and take corrective measures quickly, ensuring sustainable operations.
Another significant application is in predictive maintenance. By analyzing historical data, ML can anticipate failures in system components. This foresight allows organizations to perform maintenance tasks before issues arise, thus optimizing resources and reducing operational costs. With the increasing reliance on cloud-based systems and services, ML continues to prove its worth by adapting to ever-changing workloads.
"AI technology is revolutionizing IT operations. It allows for unprecedented levels of efficiency and insight."
ML also enhances the incident response process. By learning from previous incidents, AI systems can recommend appropriate responses to new challenges. These recommendations can improve response accuracy and speed, allowing for more effective resolution.
Core Components of AIOps
AIOps, or Artificial Intelligence for IT Operations, hinges on several key components that together enhance the efficiency and effectiveness of IT management. Understanding these components is essential for professionals aiming to leverage AIOps in their organizations. The integration of AI technologies helps streamline operations, paving the way for enhanced productivity and quicker responses to incidents. In this section, we will examine three core components: Data Ingestion and Management, Event Correlation and Analysis, and Automation and Remediation Processes.
Data Ingestion and Management
Data ingestion refers to the process of collecting and importing data from various sources into a centralized system for analysis. In the context of AIOps, this aspect ensures that vast amounts of data generated from servers, applications, and network devices can be effectively captured and utilized.
The management of this data is of equal importance. Proper data storage and organization are critical to derive actionable insights. Issues such as data silos or unstructured data can hinder operational efficiency. Thus, adopting robust data management practices is essential. Processing techniques like filtering and normalization prepare the data for further analysis. This enables IT professionals to make informed decisions based on aggregated information.
Key points about Data Ingestion and Management include:
- Collection from diverse sources such as logs, metrics, and events.
- Ensuring data quality through validation and cleansing processes.
- Enhancing accessibility with unified data platforms.
Event Correlation and Analysis
Event correlation and analysis is vital for identifying relationships between different events and incidents. AIOps leverages machine learning algorithms to detect patterns and anomalies in time-series data. This component facilitates a deeper understanding of how various incidents may be interrelated. By correlating events, IT teams can prioritize responses and reduce the time spent diagnosing root causes.
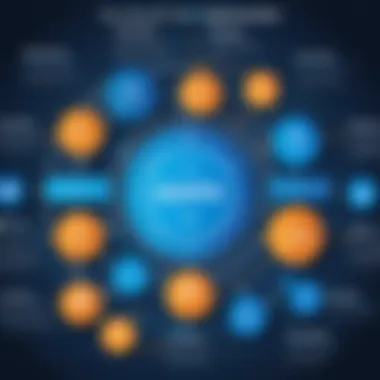
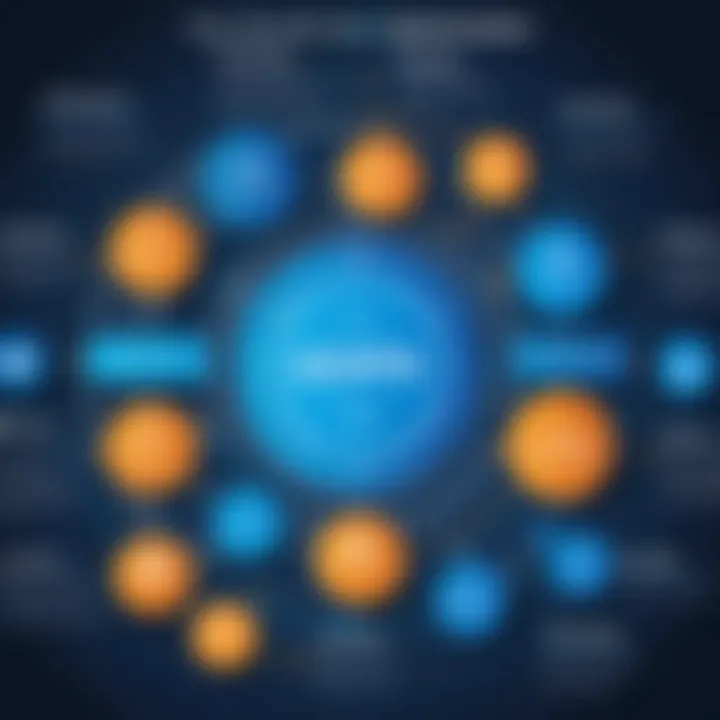
The ability to analyze these events in real-time also offers the opportunity for proactive incident management. Sophisticated algorithms can highlight recurring issues, leading to preemptive actions and minimizing disruptions.
"Effective event correlation can drastically reduce incident resolution times and enhance service availability."
Important aspects of Event Correlation and Analysis are:
- Real-time monitoring of system performance.
- Automated grouping of related incidents for easier resolution.
- Use of predictive analytics to foresee potential issues before they manifest.
Automation and Remediation Processes
Automation represents one of the most compelling aspects of AIOps, allowing for the reduction of manual efforts in IT operations. Automated workflows enable continuous monitoring, swift problem identification, and immediate remediation. This leads to decreased downtime and increased overall efficiency.
Remediation processes can often be executed automatically based on predefined rules and heuristics. For instance, if a server experiences a performance drop, AIOps can trigger predefined remediation steps, such as scaling resources or restarting services. This capability ensures that IT teams can focus on more strategic tasks rather than getting bogged down by routine maintenance duties.
Some critical points about Automation and Remediation Processes are:
- Streamlined workflows reduce human errors and improve efficiency.
- Immediate response to incidents minimizes impacts on business operations.
- Continuous improvement through machine learning entails refining processes based on past incidents.
Benefits of Implementing AIOps
Implementing AIOps brings multiple advantages to organizations in the realm of IT operations. This approach leverages artificial intelligence to streamline myriad processes, ultimately enhancing efficiency. Here, we will explore core benefits that highlight the significance and relevance of AIOps in modern IT environments.
Enhanced Operational Efficiency
AIOps significantly enhances operational efficiency by automating routine tasks and improving overall workflow. With the ability to collect and analyze massive amounts of data in real-time, AIOps tools help IT teams identify bottlenecks quickly. This leads to a more effective allocation of resources, as IT personnel can shift their focus from repetitive tasks to more strategic initiatives.
Moreover, AIOps solutions enable organizations to respond to incidents with greater speed. They provide actionable insights that assist teams in prioritizing issues based on severity and potential impact. By automating alerting and monitoring processes, AIOps reduces human error and increases reliability. For IT specialists, the outcome is a more fluid and productive work environment.
Improved Incident Response
Improving incident response is another critical component of AIOps deployment. These systems are designed to analyze incidents and correlate events to provide a clearer picture of underlying issues. As a result, IT teams can investigate problems more effectively, reducing downtime and the business impact of any disruptions.
By using advanced analytical techniques, AIOps can distinguish between relevant alerts and irrelevant noise. The significance of this is profound; it reduces alert fatigue, allowing IT teams to concentrate on what truly matters. Faster diagnoses and resolutions lead to higher uptime and user satisfaction. In today's 24/7 operating environments, the speed of incident response is vital for maintaining service levels.
Proactive Problem Resolution
AIOps encourages a proactive approach to problem resolution rather than a reactive one. By continuously monitoring systems and analyzing data trends, AIOps can identify potential issues before they escalate into significant problems. This predictive capability allows IT teams to address vulnerabilities and performance dips, ultimately enhancing service reliability.
Moreover, AIOps systems can implement automated remediation strategies. Once a potential problem is detected, the system can execute predefined tasks to resolve the issue. This means that many concerns are managed without human intervention, freeing up IT professionals for more complex tasks. The proactive stance of AIOps is particularly advantageous as it cultivates an environment of continuous improvement.
In summary, AIOps not only improves efficiency and incident response but also enables organizations to stay ahead of potential challenges, fostering a more resilient IT infrastructure.
By understanding these benefits, cybersecurities professionals and IT specialists can appreciate how AIOps contributes to their organizations' success. It is clear that the integration of AIOps is not just a trend but a necessity in the evolving landscape of IT operations management.
Challenges and Limitations of AIOps
As organizations embrace the potential of AIOps, it is essential to recognize the challenges and limitations that come with this technology. Understanding these hurdles is crucial for IT professionals who seek to implement AIOps effectively while maximizing its benefits. Addressing issues such as data quality, integration, and skill gaps within teams can facilitate smoother adoption.
Data Quality and Integration Issues
Data is the backbone of AIOps. It is the driving force behind the insights and automation that AIOps offers. However, if the data quality is poor, the outcomes may also be disappointing. Inconsistent, incomplete, and outdated data can lead to erroneous insights. This makes it hard for organizations to trust the systems and processes informed by AIOps.
Moreover, the integration of data from different sources can present significant trouble. Companies often operate with legacy systems that may not easily connect with modern AIOps platforms. This creates silos, hindering a unified approach to data management. A fragmented data landscape complicates the ability of AIOps to deliver holistic insights, ultimately affecting operational efficiency.
"Organizations must focus on ensuring data quality and seamless integration to fully leverage AIOps capabilities."
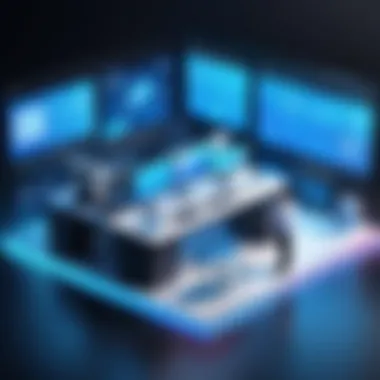
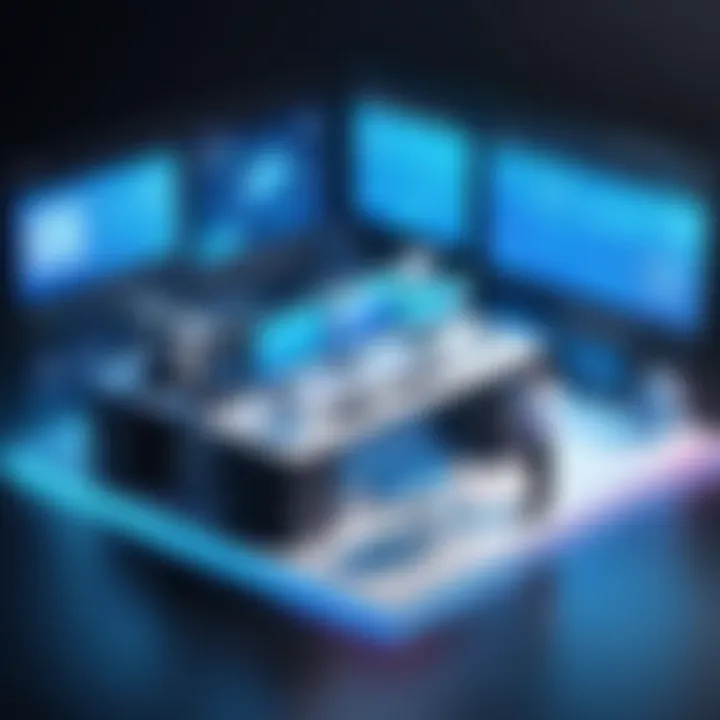
Skill Gaps in IT Teams
Even with cutting-edge technology at their disposal, organizations might struggle due to skill gaps within their IT teams. Implementing AIOps requires a strong understanding of both artificial intelligence and operational processes. Not all IT professionals have the expertise to maximize AIOps tools effectively. This shortfall can lead to suboptimal use of resources, resulting in underwhelming results.
Organizations need to invest in training and development to help their teams understand AIOps better. Providing education on machine learning, data analytics, and automation practices is essential to bridge these gaps. Without this investment in human capital, the full potential of AIOps will remain untapped, and companies may find themselves behind in the competitive IT landscape.
Real-world Applications of AIOps
Exploring the real-world applications of AIOps offers valuable insight into its transformative potential in IT operations. AIOps facilitates efficiency and agility, enabling organizations to optimize their resources better. Not only does it enhance incident management but also drives proactive measures that reduce downtime. It allows IT teams to improve decision-making through data-driven insights, making it essential for modern digital landscapes.
The significance of AIOps in practical scenarios can be viewed through its effectiveness across various industries, ranging from finance to healthcare. Each sector leverages AIOps differently, addressing unique challenges and requirements. By implementing AIOps, organizations can streamline processes, minimize human error, and utilize automation to handle repetitive tasks.
"AIOps creates an environment where IT teams can focus on strategic tasks instead of getting bogged down by routine operational issues."
Case Studies in Different Industries
Examining specific case studies highlights how various industries apply AIOps effectively. For example, in the finance sector, companies like JPMorgan Chase have adopted AIOps to enhance fraud detection. By analyzing vast amounts of transaction data, AIOps algorithms identify patterns that signal potential threats, enabling swift action to mitigate risks.
In contrast, the healthcare industry implements AIOps to improve patient care through better management of IT systems. Organizations can monitor patient data, system integrity, and network performance to ensure that critical services remain online. For instance, a healthcare provider using AIOps can diagnose issues in real-time with minimal human intervention, leading to quicker responses during emergencies.
Moreover, the retail sector benefits from AIOps by optimizing supply chain operations. With tools that analyze inventory levels and consumer behavior, retailers can make informed decisions that leverage operational efficiency.
Success Stories of AIOps Implementation
Successful implementations of AIOps demonstrate its capacity to drive operational excellence. For instance, a telecommunications company, AT&T, utilized AIOps to enhance service reliability. By deploying machine learning models that analyze system performance, AT&T reduced network downtime significantly, resulting in improved customer satisfaction.
Similarly, Netflix employs AIOps principles to manage its streaming infrastructure. By analyzing user behavior and system metrics, Netflix can predict demands and prevent service interruptions. This has not only elevated user experience but also optimized resource allocation across data centers.
Furthermore, companies in the manufacturing industry have started leveraging AIOps for predictive maintenance. By using data collected from machinery, these organizations can anticipate failures and perform timely maintenance, enhancing productivity and reducing costs.
Future Trends in AIOps
The landscape of IT operations management is undergoing a significant transformation. The concept of AIOps is vital in shaping how organizations approach IT challenges. Understanding future trends in AIOps offers insight into the next phase of operational excellence. It is essential for IT professionals to be aware of these shifts as they position their organizations for greater efficiency and effectiveness.
Emerging Technologies and Innovations
As AIOps evolves, several technologies are poised to redefine its capabilities. Cloud computing remains a cornerstone of AIOps innovation. It allows for scalable resources that can adapt to varying workloads. Technologies like Kubernetes and Docker also enable streamlined deployment of applications, enhancing operational agility.
Another noteworthy technology is the use of edge computing. With the increase of IoT devices, processing data closer to the source enhances real-time decision making. This trend aligns with AIOps, which thrives on quick data analysis and response. Integrating these technologies into AIOps frameworks can minimize latency and improve user experience.
Automation is also becoming a hallmark of AIOps. Robotic Process Automation (RPA) works alongside machine learning algorithms, allowing IT teams to offload routine tasks. This can lead to improved focus on strategic objectives rather than operational minutiae.
Predictions for AIOps Evolution
Looking ahead, AIOps will likely expand its role within IT operations. One prediction is the increasing integration of multi-cloud environments. As organizations adopt hybrid solutions, AIOps will need to consolidate data across platforms effectively. This can provide a unified view for quicker analysis and streamlined processes.
Furthermore, the role of predictive analytics is expected to grow. AIOps will utilize data not just reactively but proactively. Identifying potential incidents before they escalate may become a norm, leading to improved incident management.
Security operations will also see a notable emphasis. The convergence of AIOps and security frameworks can help organizations address vulnerabilities within their operations. A focused approach towards securing data as it flows through various systems is a future necessity.
The End
In this article, we have explored various facets of AIOps and its role in transforming IT operations management. The importance of AIOps cannot be overstated, especially in an era where efficiency and speed are critical. As organizations become increasingly dependent on digital solutions, understanding how to leverage AIOps is essential for IT professionals.
Summary of Key Insights
Several key insights emerge from our discussion.
- Integration of AI Technologies: AIOps utilizes advanced artificial intelligence and machine learning algorithms to analyze large volumes of data in real-time. This capability enhances decision-making and helps identify issues before they escalate, reducing downtime.
- Operational Efficiency: By automating routine tasks and incidents, AIOps allows IT teams to focus on strategic initiatives rather than day-to-day operational concerns. This shift is crucial for optimizing resource allocation and improving overall productivity.
- Challenges and Solutions: While the benefits of AIOps are substantial, challenges such as data quality and skill gaps persist. Addressing these issues is vital for successful implementation. Training personnel and ensuring data integrity are steps organizations must take.
- Real-world Applications: Case studies illustrate how various industries apply AIOps for improved incident response and proactive problem resolution. These applications highlight the practical benefits of AIOps and its versatility across different sectors.
Final Thoughts on AIOps
The future of IT operations management will likely depend heavily on AIOps. As the technology matures, the focus should be on fostering collaboration between AI systems and human experts. This partnership has the potential to unlock new efficiencies and innovations in the tech landscape.