Unveiling the Indispensable Role of Data Loss Prevention (DLP) in Cyber Security Measures
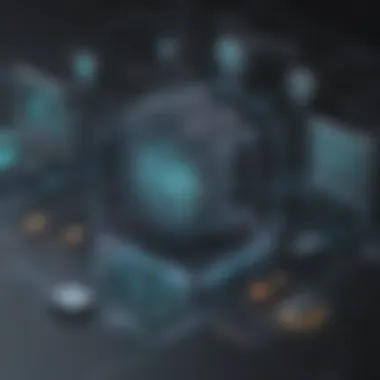
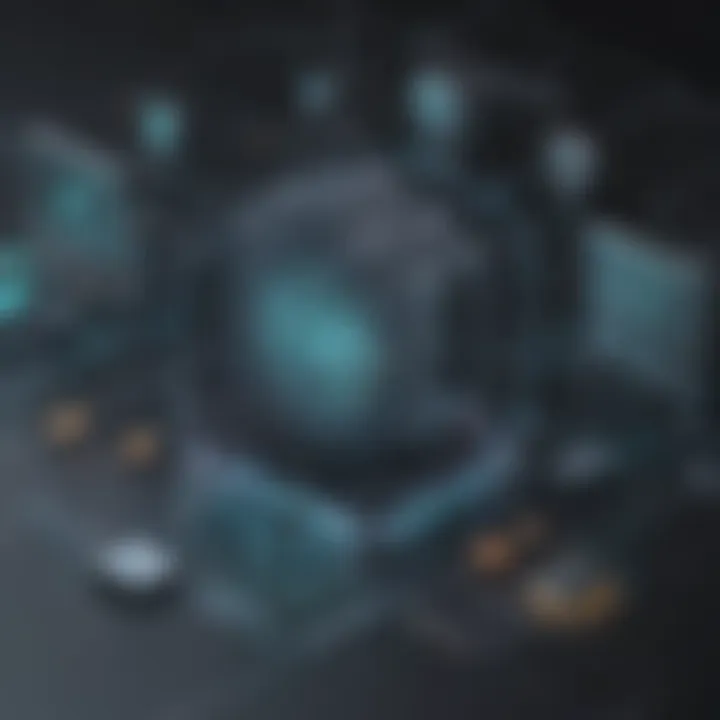
Introduction to Cybersecurity and Network Security Convergence
In today's interconnected digital landscape, where information travels at lightning speed across vast networks, the significance of cybersecurity cannot be overstated. As technology continues to advance, the fusion of cybersecurity with network security has become imperative in safeguarding sensitive data from malicious threats. The evolution of networking and security convergence has paved the way for more sophisticated cyber defense mechanisms, enhancing the resilience of organizations against evolving cyber threats.
Securing People, Devices, and Data
In the realm of cybersecurity, the security of people, devices, and data is paramount. Implementing robust security measures across all facets of digital data is essential to prevent unauthorized access, data breaches, and cyber attacks. Securing personal devices, networks, and sensitive information requires a multi-layered approach that encompasses encryption, access controls, and regular security updates to mitigate vulnerabilities effectively.
Latest Trends in Security Technologies
The dynamic landscape of cybersecurity is witnessing the emergence of innovative technologies that are reshaping the traditional paradigms of network security. Artificial Intelligence (AI), Internet of Things (IoT), and cloud security have revolutionized the way organizations approach cybersecurity. The integration of AI-powered threat detection systems, IoT security protocols, and robust cloud security measures have bolstered network defenses, providing comprehensive protection against a wide array of cyber threats.
Data Breaches and Risk Management
Recent data breaches have underscored the critical importance of robust risk management practices in cybersecurity. By analyzing case studies of prominent data breaches and their far-reaching implications, organizations can gain valuable insights into identifying vulnerabilities and strengthening their defenses. Implementing best practices for risk identification, such as regular security audits, employee training, and incident response plans, is essential for minimizing the impact of potential cyber attacks.
Future of Cybersecurity and Digital Security Technology
As technology continues to evolve at a rapid pace, the future of cybersecurity holds promise and challenges in equal measure. Predictions for the cybersecurity landscape point towards increased automation, threat intelligence, and cybersecurity tool sophistication. Innovations and advancements in digital security technology are shaping a more resilient and adaptive digital security ecosystem that is poised to defend against emerging cyber threats with agility and efficacy.
Introduction to Data Loss Prevention (DLP)
Data Loss Prevention (DLP) plays a critical role in cybersecurity by ensuring the protection of sensitive information from unauthorized access or exfiltration. In the context of this article, understanding the fundamental principles of DLP is paramount to grasping its significance within cyber defense strategies. By implementing DLP solutions, organizations can establish robust controls over data flows, detect and prevent potential breaches, and enforce compliance with industry regulations. This section will delve into the key components, benefits, and considerations surrounding the Introduction to Data Loss Prevention.
Understanding the Basics of DLP
Definition of DLP
DLP, in essence, encompasses a set of tools, processes, and policies designed to prevent the unauthorized transmission of sensitive data. This fundamental aspect of DLP enables organizations to identify, monitor, and protect crucial information assets throughout their lifecycle. The unique offering of DLP lies in its ability to provide granular visibility into data usage patterns, facilitating proactive risk mitigation and incident response. While the advantages of DLP include enhanced data security and regulatory compliance, challenges such as implementation complexity and false positives may arise, necessitating a nuanced approach to its integration.
DLP Objectives
The primary objectives of DLP revolve around safeguarding sensitive data, maintaining the integrity of critical assets, and preempting security incidents. By aligning DLP objectives with organizational security policies, businesses can fortify their defenses against insider threats, external attacks, and inadvertent data leaks. The distinct feature of DLP objectives lies in their emphasis on continuous monitoring, real-time threat detection, and adaptive security controls. While the advantages of pursuing DLP objectives are evident in risk reduction and incident response efficiency, potential drawbacks may manifest in resource-intensive deployments and operational complexities.
Components of DLP Solutions
DLP solutions are multifaceted, comprising integral components such as content discovery, policy management, incident response, and reporting functionalities. These components work in concert to ensure comprehensive data protection across diverse environments, including on-premises, cloud, and endpoint devices. The salient characteristic of DLP solutions lies in their customizable nature, allowing organizations to tailor security policies based on data classification, user roles, and regulatory requirements. While the advantages of leveraging DLP components encompass improved data visibility and regulatory alignment, challenges such as integration complexities and scalability limitations may necessitate careful planning and implementation.
Significance of DLP in Cyber Security
Preventing Data Breaches
One of the primary imperatives of DLP is to prevent data breaches by proactively identifying and mitigating vulnerabilities within the data ecosystem. By implementing robust data loss prevention mechanisms, organizations can detect anomalous behaviors, enforce encryption protocols, and restrict unauthorized data access. The key characteristic of preventing data breaches through DLP lies in its focus on risk mitigation, data encryption, and user activity monitoring. While the advantages of this approach include reduced breach incidents and minimized financial losses, potential disadvantages may stem from user privacy concerns and performance impact on system operations.
Protecting Intellectual Property
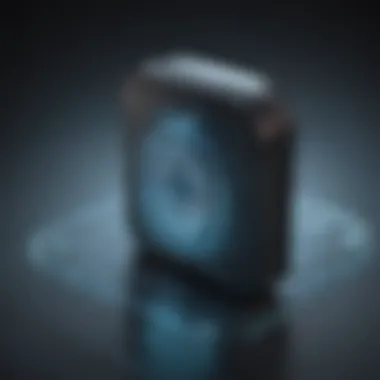
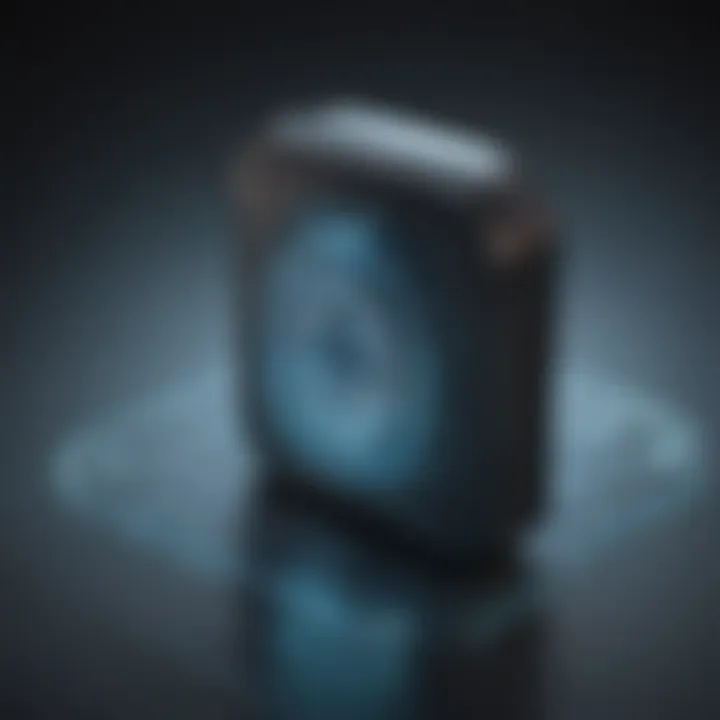
DLP plays a pivotal role in safeguarding intellectual property by establishing controls over critical data assets and intellectual capital. By enforcing policies that govern data usage, sharing, and retention, organizations can safeguard proprietary information from unauthorized disclosure or theft. The unique feature of protecting intellectual property through DLP lies in its support for data classification, digital rights management, and threat intelligence integration. While the advantages of this protection mechanism include intellectual asset preservation and competitive advantage sustenance, challenges such as content classification errors and endpoint vulnerabilities may necessitate ongoing refinement of IP protection strategies.
Compliance Requirements
Compliance with regulations and industry standards is a core element of cyber resilience, with DLP serving as a cornerstone for meeting legal mandates and data protection obligations. By aligning DLP practices with regulatory frameworks such as GDPR, HIPAA, and various cybersecurity standards, organizations can avoid penalties, reputational damage, and data privacy violations. The key characteristic of compliance requirements in DLP lies in their emphasis on policy enforcement, audit trail maintenance, and incident response readiness. While the advantages of complying with legal mandates include enhanced trust with stakeholders and minimized legal risks, potential drawbacks may arise from evolving regulatory landscapes and the dynamic nature of compliance standards.
Integration of DLP into Security Strategies
DLP and Endpoint Security
The integration of DLP with endpoint security solutions is essential for extending data protection mechanisms to individual devices and terminals within enterprise networks. By combining endpoint security controls with DLP technologies, organizations can establish a defense-in-depth strategy that safeguards data both at rest and in transit. The key characteristic of DLP and endpoint security integration lies in their seamless collaboration in monitoring user behavior, enforcing data encryption, and blocking unauthorized transmissions. While the advantages of this integration encompass comprehensive endpoint protection and data loss prevention, challenges such as compatibility issues and performance overhead may necessitate strategic deployment and configuration adjustments.
Role of DLP in Network Security
DLP plays a pivotal role in network security by monitoring data flows, detecting anomalies, and preventing unauthorized data exfiltration across network infrastructure. By integrating DLP capabilities with network security protocols, organizations can bolster their defenses against external threats, malicious activities, and data leakage incidents. The unique feature of the role of DLP in network security lies in its ability to provide visibility into network traffic, apply data loss prevention policies, and respond to potential security incidents in real time. While the advantages of leveraging DLP for network security include enhanced threat detection and network visibility, challenges such as performance impact and secure data transmission may require meticulous network architecture design and continuous monitoring.
Collaboration with SIEM Solutions
The collaboration between DLP and Security Information and Event Management (SIEM) solutions enhances cyber threat intelligence, incident correlation, and security incident response capabilities. By integrating DLP event data with SIEM platforms, organizations can streamline the detection, investigation, and remediation of security incidents through centralized log management and advanced analytics. The unique feature of collaborating with SIEM solutions using DLP lies in the amalgamation of data protection policies with threat intelligence feeds, correlation rules, and security event monitoring. While the advantages of this collaboration involve enhanced situational awareness and proactive incident mitigation, challenges such as data volume management and alert fatigue mitigation may necessitate refined SIEM integration strategies and continuous tuning of correlation rules.
Advanced Concepts in DLP Technology
In the realm of cyber security, Advanced Concepts in DLP Technology play a pivotal role in enhancing data protection measures and fortifying defense mechanisms against evolving threats. Understanding these advanced concepts is crucial for organizations looking to safeguard their sensitive information effectively. By incorporating Behavioral Analytics and Machine Learning into DLP solutions, businesses can stay ahead of potential breaches and unauthorized data access.
Behavioral Analytics and Machine Learning in DLP
Behavior Monitoring
Behavior Monitoring within DLP technology involves tracking and analyzing user actions and behavioral patterns to identify any anomalies or suspicious activities that could indicate a potential security threat. This proactive approach allows organizations to detect unauthorized access or data misuse in real-time, minimizing the risk of data breaches. However, the continuous monitoring process can increase the complexity of security operations and may require additional resources to manage effectively.
Anomaly Detection
Anomaly Detection is a core component of DLP technology that focuses on identifying deviations from normal behavior within the network or system. By leveraging machine learning algorithms, anomaly detection can detect unknown security threats and zero-day attacks that traditional security measures may miss. While enhancing threat detection capabilities, anomaly detection may sometimes generate false positives, leading to resource-intensive investigations.
Predictive Analysis
Predictive Analysis in DLP technology utilizes historical data and machine learning algorithms to forecast potential security incidents and risks. By analyzing patterns and trends, predictive analysis empowers organizations to implement proactive security measures and mitigate threats before they materialize. However, the effectiveness of predictive analysis highly depends on the quality of data input and the accuracy of the underlying algorithms, posing challenges in achieving reliable predictions.
Cloud-based DLP Solutions
The adoption of Cloud-based DLP Solutions presents both opportunities and challenges for organizations seeking to secure their data in cloud environments. Addressing the Challenges in Cloud Security is essential to ensure the integrity and confidentiality of data stored and transmitted through cloud services. Organizations must navigate issues such as data sovereignty, shared responsibility models, and compliance requirements when implementing cloud-based DLP solutions.
On the flip side, the Benefits of Cloud DLP offer scalability, flexibility, and cost-efficiency compared to traditional on-premises solutions. Cloud DLP solutions enable seamless data protection across diverse cloud platforms and applications, supporting modern work environments with remote workers and distributed teams. However, organizations must carefully consider factors such as data encryption, access controls, and regulatory compliance when deploying cloud DLP solutions.
Implementation Considerations play a critical role in the successful deployment of cloud-based DLP solutions. Organizations need to evaluate factors like data migration strategies, integration with existing security tools, and vendor capabilities to ensure a smooth transition to the cloud. While cloud-based DLP solutions offer numerous advantages, organizations must address implementation challenges like data visibility, user training, and incident response protocols to maximize the effectiveness of their security posture.
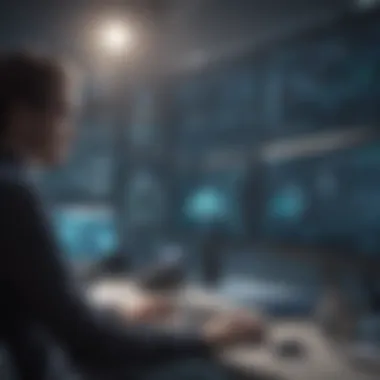
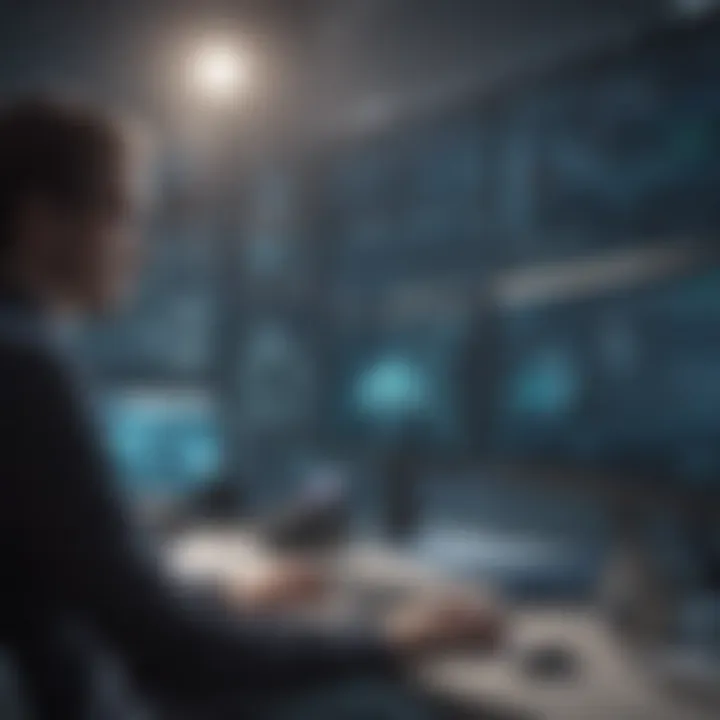
Mobile Device Management () and DLP Integration
The integration of Mobile Device Management (MDM) with DLP solutions is essential for securing sensitive data accessed and shared through mobile devices. Securing Mobile Data requires comprehensive policies and controls to prevent data leakage or unauthorized access from mobile endpoints. Organizations must implement encryption, remote data wiping, and device authentication mechanisms to protect data at rest and in transit.
Policy Enforcement mechanisms ensure compliance with organizational security policies and regulatory requirements across mobile devices. By enforcing access controls, data retention policies, and application restrictions, organizations can mitigate the risks associated with mobile data usage and enforce consistent security practices. However, balancing security measures with user convenience and productivity remains a challenge in mobile device management and DLP integration.
Cross-Platform Compatibility is vital for ensuring seamless DLP capabilities across a diverse range of operating systems and device types. Organizations must consider the interoperability of DLP solutions with mobile platforms, operating system updates, and third-party applications to maintain effective data protection measures. Achieving cross-platform compatibility requires continuous monitoring, regular updates, and agile adaptation to emerging technologies and security threats.
Best Practices for Implementing DLP Strategies
Data Loss Prevention (DLP) strategies play a pivotal role in enhancing cybersecurity measures. The implementation of DLP strategies involves a meticulous approach to safeguard sensitive data, mitigate potential risks, and ensure compliance with industry regulations. By focusing on best practices for implementing DLP strategies, organizations can fortify their security posture and proactively address data protection challenges. These practices encompass various elements, including robust policy development, user awareness programs, and monitoring mechanisms, to establish a comprehensive DLP framework.
Effective DLP Policy Development
Risk Assessment
Risk assessment serves as a foundational element in DLP policy development, facilitating a systematic evaluation of potential threats and vulnerabilities. Conducting a thorough risk assessment allows organizations to identify critical assets, assess the likelihood of security incidents, and prioritize risk mitigation strategies. The key characteristic of risk assessment lies in its ability to provide a data-driven perspective on security risks, enabling informed decision-making and resource allocation. Despite its benefits, risk assessment may entail certain challenges, such as the complexity of assessing evolving threats and ensuring continuous risk monitoring.
Data Classification
Data classification is instrumental in organizing and categorizing data based on its sensitivity and confidentiality levels. By assigning appropriate classification labels to data assets, organizations can enforce access controls, encryption protocols, and data retention policies effectively. The key advantage of data classification lies in its ability to streamline data management processes, enhance data visibility, and streamline compliance efforts. However, challenges may arise in maintaining consistent classification standards across diverse data sets and ensuring effective data labeling practices.
Incident Response Planning
Effective incident response planning is paramount in DLP policy development to mitigate the impact of security breaches and unauthorized data access. Incident response plans outline the steps and protocols to be followed in case of a data breach, ensuring timely detection, containment, and recovery efforts. The key characteristic of incident response planning is its proactive approach to cybersecurity incidents, enabling organizations to minimize downtime, reputational damage, and financial losses. However, the effectiveness of incident response plans relies on regular testing, updates based on emerging threats, and coordination across internal teams and external stakeholders.
User Training and Awareness Programs
Phishing Awareness
Phishing awareness programs are essential components of DLP strategies, aiming to educate users on recognizing and mitigating phishing attacks. These programs typically involve simulated phishing campaigns, interactive training modules, and security awareness assessments to enhance user vigilance. The key advantage of phishing awareness lies in its ability to empower users to identify phishing red flags, avoid clicking on malicious links, and report suspicious emails promptly. Nevertheless, maintaining user engagement, addressing evolving phishing tactics, and measuring the effectiveness of awareness programs pose ongoing challenges.
Social Engineering Prevention
Social engineering prevention initiatives focus on raising awareness about manipulative techniques used by threat actors to deceive individuals and gain unauthorized access to sensitive information. By educating users on common social engineering tactics, such as pretexting and baiting, organizations can bolster their defenses against social engineering attacks. The key characteristic of social engineering prevention is its emphasis on human behavior and psychological manipulation, requiring a blend of technical controls and user education. However, the dynamic nature of social engineering schemes necessitates continuous training updates and adaptive prevention strategies.
Data Handling Guidelines
Data handling guidelines establish clear protocols for data access, storage, sharing, and disposal to maintain data integrity and confidentiality. These guidelines define proper data usage practices, data classification criteria, and data protection measures to minimize data leakage risks. The key feature of data handling guidelines is their role in promoting data security awareness, fostering a culture of data privacy, and ensuring regulatory compliance. Challenges may arise in aligning data handling guidelines with evolving data privacy laws, training diverse user groups on guideline adherence, and enforcing consistent data handling practices.
Monitoring and Auditing DLP Effectiveness
Real-time Monitoring
Real-time monitoring capabilities are critical for assessing DLP solution performance and detecting security incidents in real-time. By monitoring data flows, user activities, and policy violations in real-time, organizations can swiftly respond to unauthorized data access attempts and anomalous behaviors. The key advantage of real-time monitoring is its ability to provide immediate threat visibility, enable fast incident response, and support forensic investigations. However, the scalability of real-time monitoring systems, data volume challenges, and alert fatigue may pose operational hurdles.
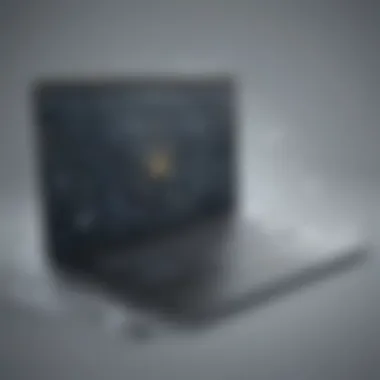
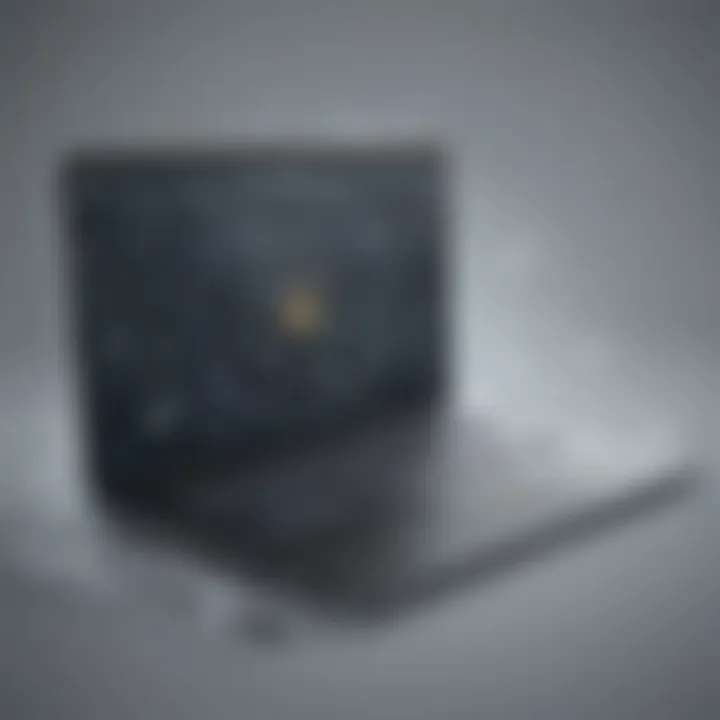
Compliance Audits
Conducting compliance audits is essential to evaluate the effectiveness of DLP measures in aligning with regulatory requirements and internal security policies. Compliance audits encompass assessments of data protection controls, access permissions, audit trails, and incident response procedures to ensure regulatory adherence. The key characteristic of compliance audits is their role in verifying DLP compliance gaps, addressing non-compliance issues, and demonstrating due diligence to regulatory bodies. Nonetheless, the complexity of regulatory frameworks, evolving compliance standards, and audit process intricacies can present challenges during compliance audits.
Incident Analysis
Incident analysis entails a comprehensive review of security incidents, data breaches, and DLP alerts to identify root causes, patterns of compromise, and areas for enhancement. By analyzing incident data, organizations can refine their incident response procedures, update security controls, and address underlying vulnerabilities. The key feature of incident analysis is its contribution to continuous improvement in security postures, incident response efficacy, and threat intelligence integration. However, challenges may arise in resource-intensive incident investigations, cross-team collaboration for incident analysis, and prioritizing remediation actions based on incident findings.
Emerging Trends in DLP and Cyber Security
Emerging trends in Data Loss Prevention (DLP) and cybersecurity are crucial aspects to consider in the ever-evolving landscape of data protection. As technology advances, new challenges and opportunities arise, making it imperative for organizations to stay ahead of potential threats. AI and Automation play integral roles in enhancing DLP capabilities. These trends enable quicker detection of anomalies and potential breaches, bolstering overall cybersecurity posture. Machine Learning Algorithms drive AI capabilities within DLP systems, allowing for adaptive threat detection and real-time response. Their key characteristic lies in their ability to learn from data patterns and make predictions based on past behaviors, making them a popular choice for organizations seeking proactive security measures. Despite their advantages, ensuring the accuracy and relevancy of machine learning models poses challenges that require continuous refinement. On the other hand, Automated Response Systems streamline incident handling processes by automating threat containment and mitigation actions based on predefined rules. Their key characteristic is the rapid response time, minimizing potential damages from cyber incidents. However, ensuring the appropriate calibration of automated responses to prevent false positives or negatives remains essential. Lastly, Threat Intelligence Integration enriches DLP solutions with real-time data on emerging threats and vulnerabilities. Their key characteristic involves providing context and insights to security incidents, empowering organizations to make informed decisions promptly. While valuable, integrating threat intelligence sources seamlessly can be complex due to varying data formats and sources. These emerging trends underscore the importance of AI-driven solutions and streamlined automation in strengthening cybersecurity defenses.
AI and Automation in DLP
Machine Learning Algorithms
Machine Learning Algorithms form a critical component of enhancing DLP capabilities by enabling adaptive threat detection and real-time response. Their unique feature lies in their ability to analyze large datasets, identify patterns, and predict potential threats. Leveraging machine learning algorithms enhances the overall accuracy and efficiency of threat detection in DLP systems. Despite their advantages, such as reducing false positives and providing predictive insights, challenges like model interpretability and tuning complexity must be addressed to ensure optimal performance in cybersecurity settings.
Automated Response Systems
Automated Response Systems streamline incident response processes by automatically executing predefined actions to contain and mitigate security threats. Their key feature is the rapidity in response, enabling organizations to address cyber incidents promptly and reduce potential damages. However, organizations must continuously assess and refine automated response configurations to align with evolving threat landscapes and minimize the risk of erroneous actions.
Threat Intelligence Integration
Integrating Threat Intelligence into DLP solutions enhances security posture by providing real-time insights on emerging threats and vulnerabilities. The unique feature of Threat Intelligence Integration lies in its ability to offer context and actionable information to support timely decision-making. Organizations benefit from enriched threat data that enables proactive threat detection and response. Yet, challenges like data quality and ensuring compatibility with existing security systems necessitate careful attention and integration strategies to maximize the effectiveness of threat intelligence in cybersecurity defenses.
Regulatory Impact on DLP Implementation
GDPR Compliance
GDPR Compliance plays a vital role in shaping DLP strategies, particularly in safeguarding personal data and ensuring data privacy. Its key characteristic lies in establishing stringent guidelines on data protection and user consent, aiming to harmonize data privacy laws across the EU. Organizations are compelled to implement robust data protection measures, including encryption, access controls, and data breach notification protocols to achieve GDPR compliance. Despite the benefits of enhancing data security and bolstering customer trust, meeting GDPR requirements poses challenges related to data governance, compliance audits, and regulatory complexities.
HIPAA Regulations
HIPAA Regulations dictate stringent requirements for safeguarding protected health information (PHI) within healthcare settings, influencing DLP implementations in the sector. The key characteristic of HIPAA regulations is the emphasis on maintaining the confidentiality, integrity, and availability of PHI to ensure patient privacy and data security. Healthcare organizations must adhere to strict data handling practices, such as encryption, access controls, and audit trails to comply with HIPAA regulations. While enhancing patient trust and promoting data security, HIPAA compliance demands ongoing risk assessments, staff training, and adaptation to evolving healthcare technologies.
Cybersecurity Frameworks
Cybersecurity Frameworks provide essential guidelines and best practices for organizations to establish robust cybersecurity measures and frameworks. Their key characteristic involves outlining proactive steps for risk management, incident response, and security controls to enhance overall cybersecurity resilience. Organizations benefit from aligning with established frameworks like NIST Cybersecurity Framework or ISO 27001 to strengthen their cybersecurity posture and streamline compliance efforts. However, achieving compliance and operationalizing cybersecurity frameworks necessitate resource investments, regular assessments, and alignment with industry-specific regulations to address sector-specific threats effectively.
Global Shifts in Data Protection Laws
Data Sovereignty Issues
Data Sovereignty Issues refer to the legal jurisdiction over data collected and processed within a specific geographical location, impacting data management and storage practices. The key characteristic of data sovereignty issues lies in regulating data residency requirements, ensuring data processing complies with local laws and regulations. Organizations must navigate data sovereignty regulations when deploying cloud services or managing cross-border data transfers to avoid legal infractions and data protection breaches. While data sovereignty enhances data control and legal compliance, adhering to diverse regulations across jurisdictions poses challenges in data management and operational flexibility.
Cross-border Data Transfers
Cross-border Data Transfers involve transmitting data across international boundaries, subject to varying data protection laws and regulations in different jurisdictions. The key characteristic of cross-border data transfers is navigating legal requirements, such as data localization and transfer mechanisms, to ensure lawful and secure data exchange. Organizations must implement appropriate data transfer mechanisms like Standard Contractual Clauses or Binding Corporate Rules to safeguard data privacy and comply with data protection regulations during cross-border transfers. While enabling global business operations, cross-border data transfers necessitate compliance with stringent data protection laws and agreements to mitigate data breach risks and regulatory penalties.
Privacy Shield Framework
The Privacy Shield Framework facilitates data transfers between the EU and the U.S. by ensuring adequate data protection standards and privacy principles are in place. Its unique feature lies in providing a legal mechanism for EU-U.S. data flows, enhancing transatlantic data privacy and security. Organizations certified under the Privacy Shield Framework commit to upholding stringent data protection principles and undergoing regular compliance checks to maintain certification. Despite promoting international data transfers and aligning with GDPR standards, challenges like legal uncertainties and the adequacy of data protection measures in the U.S. pose concerns in ensuring the continuity of data flows under the Privacy Shield Framework.