Unraveling the Intricacies of Cloud Data Classification in Cybersecurity
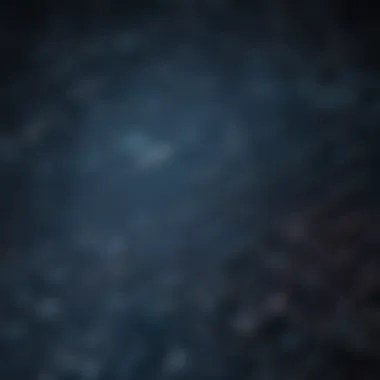
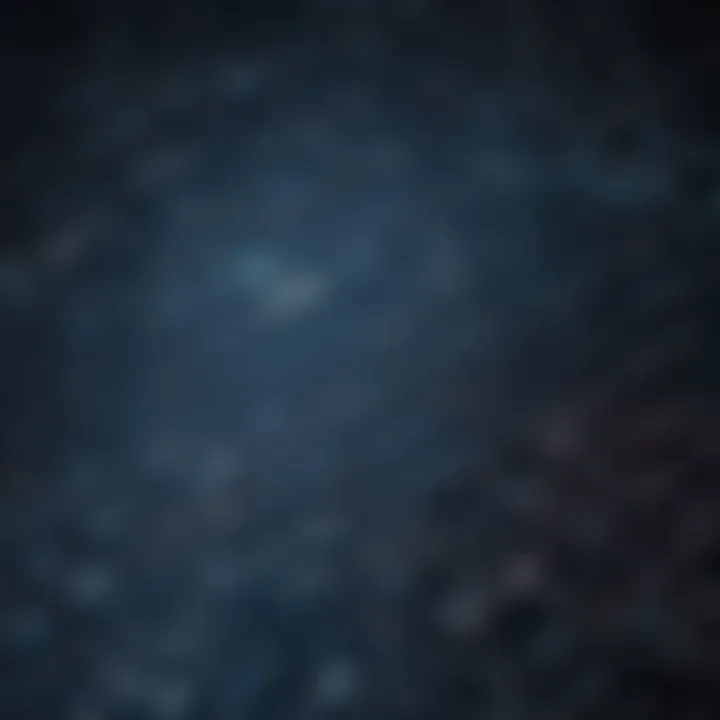
Introduction to Cloud Data Classification in Cybersecurity
In the ever-evolving landscape of cybersecurity, the paradigm of cloud data classification plays a pivotal role in ensuring the integrity and security of sensitive information. As organizations shift towards cloud computing models, understanding the nuances of data classification is imperative for fortifying digital defenses and preempting cyber threats. This section will delve into the foundational concepts and principles underpinning cloud data classification, emphasizing its criticality in safeguarding confidential data.
Significance of Cloud Data Classification
Cloud data classification serves as the cornerstone of cybersecurity frameworks, delineating data based on sensitivity levels and access permissions. By categorizing information into distinct tiers, organizations can implement targeted security measures, encryption protocols, and access controls to mitigate risks effectively. This section will elucidate the importance of structured data classification in bolstering cyber resilience and regulatory compliance within cloud environments.
Evolution of Data Classification in Cloud Environments
The evolution of data classification methodologies within cloud environments mirrors the dynamic nature of cyber threats and technological advancements. From traditional manual classification processes to automated tagging mechanisms, the evolution of data classification tools has revolutionized how organizations manage and protect their digital assets. Delving into this evolution offers valuable insights into the scalability, efficiency, and accuracy enhancements brought forth by modern cloud data classification methodologies.
Securing Cloud Infrastructure and Data Assets
Cybersecurity encompasses a multi-faceted approach towards safeguarding digital ecosystems, encompassing people, devices, and data assets residing in cloud repositories. This section extrapolates the imperative of securing every facet of cloud infrastructure, from endpoint devices to centralized data servers, against potential threat vectors and vulnerabilities.
Importance of Comprehensive Security Measures
Implementing comprehensive security measures is paramount in fortifying cloud infrastructure and protecting sensitive data from malicious actors and external breaches. From multi-factor authentication to data encryption protocols, this section will elucidate the criticality of layered security defenses in mitigating cyber risks and preserving data integrity in cloud environments.
Strategies for Enhanced Data Security
Strategies for enhancing data security in cloud environments encompass a holistic approach that integrates encryption, access controls, auditing mechanisms, and user awareness training. By amalgamating these strategies, organizations can instill a culture of security consciousness, proactively combatting emerging threats and adhering to stringent data protection mandates.
Analyzing Emerging Technologies in Cloud Security
The cybersecurity landscape is continually shaped by disruptive technologies that drive innovation and transform conventional security paradigms. This section delves into the latest trends in cloud security technologies, from AI-driven threat detection to blockchain-based data integrity solutions, elucidating their impact on fortifying cloud environments against sophisticated cyber threats.
Role of Artificial Intelligence in Cloud Security
The integration of artificial intelligence algorithms in cloud security infrastructure heralds a new era of proactive threat detection and real-time incident response. By leveraging AI-driven anomaly detection and behavioral analytics, organizations can identify and neutralize security risks before they escalate, fortifying their cloud ecosystems against impending cyber threats.
Harnessing IoT for Enhanced Data Protection
The proliferation of Internet of Things (IoT) devices augments the attack surface of cloud environments, underscoring the need for robust security measures and device authentication protocols. This section expounds on leveraging IoT technologies to bolster cloud security, mitigate risks associated with interconnected devices, and enhance data protection measures in IoT-integrated cloud architectures.
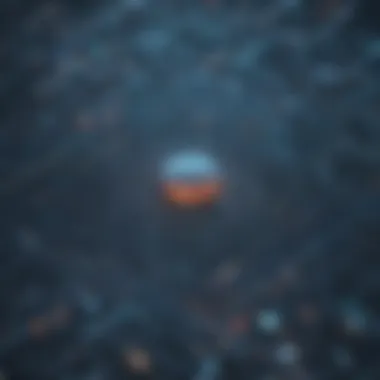
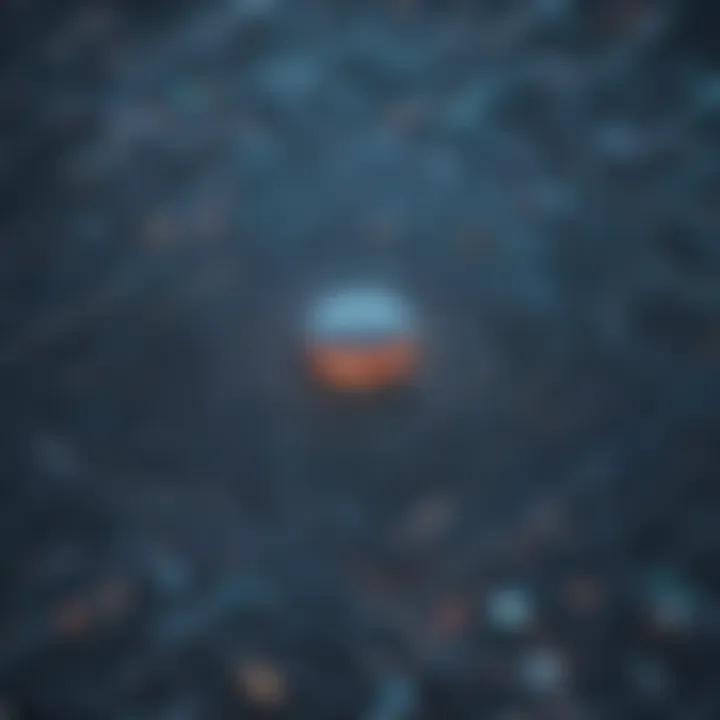
Incidents of Data Breaches and Risk Mitigation Strategies
Data breaches constitute a looming threat in today's digital landscape, underscoring the significance of proactive risk management and incident response strategies. By analyzing recent data breach incidents and their ramifications, organizations can glean valuable insights into preemptive risk mitigation tactics and data protection best practices.
Case Studies in Cybersecurity Incidents
The elucidation of prominent case studies shedding light on cybersecurity incidents offers a holistic perspective on the vulnerabilities, attack vectors, and aftermath of data breaches in various industry verticals. By dissecting these case studies, stakeholders can glean actionable insights for bolstering their cybersecurity posture and thwarting potential data breach scenarios proactively.
Implementing Risk Mitigation Best Practices
Best practices for identifying, assessing, and mitigating cybersecurity risks in cloud environments form the cornerstone of a robust risk management framework. This section will expound upon proactive risk mitigation strategies, incident response protocols, and resilience-building measures that underpin effective cybersecurity risk management, fostering a culture of vigilance and preparedness amidst evolving threat landscapes.
Envisioning the Future of Cloud Security and Digital Resilience
The future trajectory of cloud security is intrinsically linked to the convergence of disruptive technologies, regulatory mandates, and evolving cyber threat landscapes. This section prognosticates on the future of cloud security and digital resilience, extrapolating potential trends, challenges, and transformative innovations that will redefine the cybersecurity paradigm.
Evolution of Cybersecurity Ecosystem
Predicting the evolution of the cybersecurity ecosystem entails envisioning how emerging technologies, regulatory frameworks, and global cyber threats will shape the future security landscape. By analyzing current trends and anticipating future disruptions, organizations can proactively align their security strategies and investments to mitigate risks, enhance resilience, and adapt to the dynamic cybersecurity terrain.
Innovations Shaping Digital Security
Innovations in digital security technologies are poised to revolutionize how organizations safeguard their data assets, combat emerging threats, and ensure regulatory compliance in an increasingly interconnected world. This section delves into transformative innovations such as quantum-resistant cryptography, decentralized identity management, and threat intelligence platforms, elucidating their potential impact on fortifying cloud security architectures and enabling adaptive threat response mechanisms.
Introduction
In the expansive realm of cybersecurity, delving into the intricate mechanisms of cloud data classification is not merely advantageous but a necessity. Data classification serves as the backbone of data security protocols, ensuring that information is organized, secured, and accessed by the right individuals. By understanding the nuances of cloud data classification, organizations can fortify their defenses against evolving cyber threats and breaches, making it a cornerstone in contemporary digital security strategies.
Defining Cloud Data Classification
The concept of data classification
Data classification revolves around the categorization of information based on its sensitivity, importance, and access levels. This systematic approach allows organizations to label and organize data according to predefined criteria, facilitating streamlined management and protection. The key characteristic of data classification lies in its ability to tailor security measures to different data types, providing a personalized shield against potential threats. This process aids in fostering a proactive security stance, enabling preemptive actions to safeguard critical information.
Key distinctions in cloud data classification
Within cloud environments, data classification takes on unique distinctions, primarily driven by the diverse nature of data handling in cloud infrastructures. The key characteristic of cloud data classification stems from its adaptability to dynamic data storage and processing requirements, offering scalable solutions for complex data landscapes. Organizations benefit from the flexibility inherent in cloud data classification, allowing them to implement granular controls and encryption protocols tailored to the cloud environment. While these distinctions enhance data security, they also introduce challenges related to data sovereignty and regulatory adherence, necessitating careful alignment with compliance frameworks.
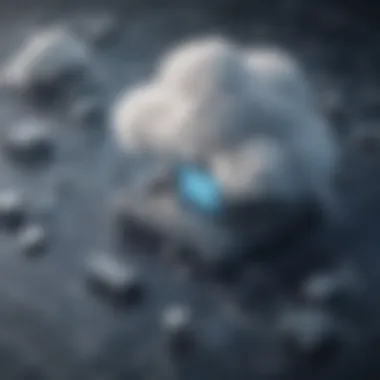
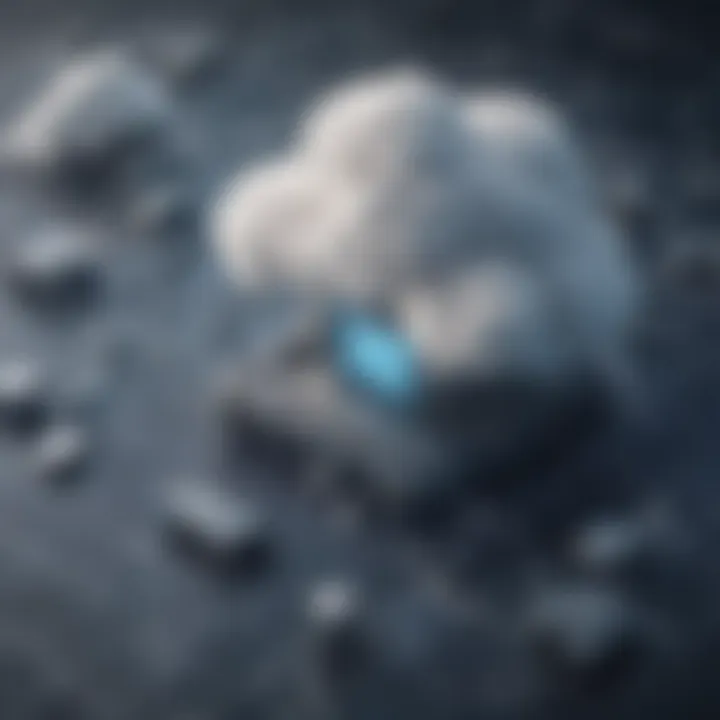
Importance of Data Classification in Cloud Security
Minimizing data breaches
One of the pivotal roles of data classification in cloud security is the mitigation of data breaches through preemptive measures. By categorizing data based on sensitivity levels and access rights, organizations can implement targeted security protocols to limit unauthorized access and reduce the surface area for potential breaches. This proactive approach minimizes the impact of security incidents, safeguarding sensitive information from malicious actors.
Enhancing data accessibility controls
Data classification not only bolsters data security but also empowers organizations to enhance data accessibility controls. By categorizing data according to relevance and importance, businesses can streamline data retrieval processes, ensuring efficient access for authorized personnel while maintaining stringent controls for sensitive information. This balance between accessibility and security optimizes data utilization within cloud environments, promoting operational efficiency while upholding data protection standards.
Challenges in Cloud Data Classification
In the realm of cybersecurity, navigating the challenges presented by cloud data classification is paramount. Understanding the nuances and complexities within this domain is crucial for safeguarding sensitive information. Data Sovereignty and Compliance Issues play a pivotal role in this landscape, delving into aspects such as data jurisdiction and regulatory compliance. Data sovereignty refers to the legal jurisdiction over data, impacting where data is stored and how it is managed, raising concerns about data control and sovereignty in cross-border data transfers. Addressing regulatory compliance involves adhering to laws and regulations governing data protection, privacy, and security, ensuring organizations operate within legal boundaries. Balancing data sovereignty with regulatory requirements poses a significant challenge, requiring meticulous attention to detail and strategic planning.
Data Sovereignty and Compliance Issues
Implications of data jurisdiction
Data jurisdiction dictates the legal framework under which data is governed, determining data ownership and access rights based on geographical location. This aspect influences data storage decisions, jurisdictional laws, and data transfer mechanisms, affecting data security and privacy considerations. The complexity of data jurisdiction entails navigating diverse legal systems, data residency requirements, and international data transfer agreements to ensure compliance and data protection. Understanding the implications of data jurisdiction aids organizations in structuring data management strategies, mitigating legal risks, and enhancing data governance practices.
Addressing regulatory compliance
Regulatory compliance entails aligning organizational practices with legal requirements to protect sensitive data effectively. Compliance frameworks such as GDPR, HIPAA, or CCPA establish guidelines for data handling, processing, and storage, safeguarding individual privacy rights and data integrity. Addressing regulatory compliance involves implementing security measures, conducting regular audits, and demonstrating adherence to compliance standards to mitigate risks of non-compliance penalties and data breaches. Upholding regulatory compliance fosters trust with stakeholders, enhances data security posture, and fortifies organizational resilience against evolving cyber threats.
Data Encryption and Privacy Concerns
Ensuring data confidentiality
Data confidentiality revolves around maintaining the secrecy and integrity of sensitive information, preventing unauthorized access or disclosure. Secure encryption algorithms and robust encryption keys are employed to shield data from unauthorized viewing or alteration, safeguarding information in transit and at rest. Ensuring data confidentiality involves implementing encryption protocols, access controls, and encryption key management practices to minimize data exposure and protect against data breaches. Prioritizing data confidentiality enhances trust with data subjects, upholds organizational reputation, and bolsters data security architecture.
Mitigating privacy risks
Mitigating privacy risks focuses on identifying and addressing vulnerabilities that may compromise data privacy and confidentiality. Organizations analyze data flows, conduct privacy impact assessments, and enhance data anonymization techniques to mitigate privacy risks effectively. By implementing robust privacy policies, user consent mechanisms, and data protection frameworks, organizations uphold privacy regulations, secure sensitive information, and cultivate a privacy-centric culture. Mitigating privacy risks instills confidence in data subjects, promotes transparency in data practices, and minimizes exposure to regulatory sanctions and reputational damage.
Best Practices for Cloud Data Classification
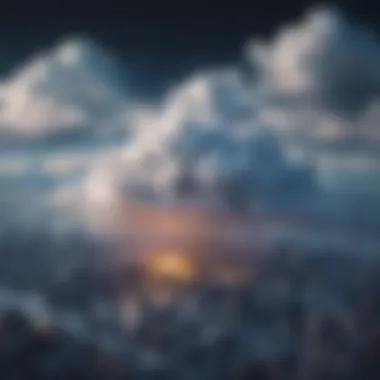
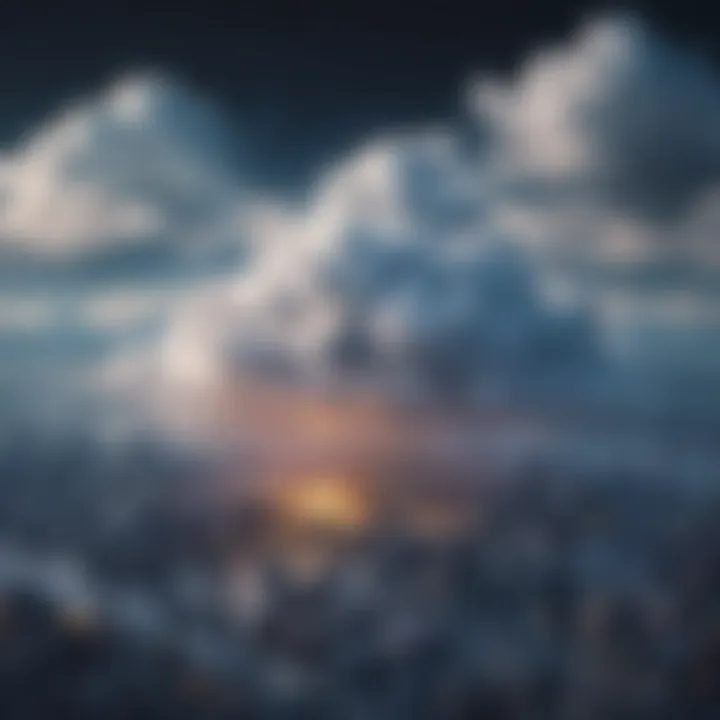
In the realm of cybersecurity, implementing best practices for cloud data classification is paramount. By adhering to robust data classification policies, organizations can effectively safeguard their sensitive information, mitigate potential risks, and enhance overall data management strategies. These best practices serve as a framework to streamline the process of categorizing and safeguarding data within cloud environments.
Implementing Robust Data Classification Policies
Defining Data Categories
When it comes to defining data categories, organizations establish a structured framework to classify data based on predefined parameters such as confidentiality, integrity, and availability. This categorization not only streamlines data storage but also enables efficient retrieval and management. By segmenting data into distinct categories, organizations can prioritize security measures tailored to the specific sensitivity level of each data set. This tailored approach enhances overall data security and ensures that appropriate controls are applied where needed.
Assigning Data Sensitivity Levels
Assigning data sensitivity levels involves evaluating the degree of sensitivity associated with each data category. By assigning clear sensitivity levels, organizations can identify critical data requiring heightened security measures and allocate resources accordingly. This granular approach enables organizations to prioritize protective measures based on the importance and impact of each data type. However, the challenge lies in maintaining a balance between robust security measures and operational efficiency. Organizations must weigh the benefits of stringent controls against the potential limitations on data accessibility to ensure a harmonious balance.
Utilizing Automated Classification Tools
Automated classification tools play a pivotal role in streamlining data classification processes within cloud environments. These tools leverage machine learning algorithms to intelligently categorize and tag data based on pre-defined parameters. By automating this process, organizations can achieve consistent and efficient data classification, reducing the margin for human error and enhancing accuracy. Furthermore, automated tools seamlessly integrate with existing cloud security platforms, enabling a holistic approach to data protection. This integration ensures that classified data is consistently monitored and enforced with appropriate security protocols.
Machine Learning Algorithms for Classification
Machine learning algorithms provide organizations with the capability to analyze vast amounts of data swiftly and accurately. By deploying machine learning algorithms for data classification, organizations can expedite the categorization process and adapt to evolving data trends. These algorithms possess the ability to identify patterns and anomalies within data sets, facilitating the efficient classification of both structured and unstructured data. The key advantage of machine learning lies in its capacity to enhance data classification accuracy over time through continuous learning and refinement.
Integration with Cloud Security Platforms
The integration of automated classification tools with cloud security platforms results in a unified approach to data protection. By combining automated classification capabilities with robust security measures, organizations can establish a comprehensive security framework that safeguards data at every level. This seamless integration enables real-time monitoring, alerting, and response to potential security threats, ensuring proactive risk mitigation. However, organizations must carefully evaluate the compatibility and scalability of integration tools to guarantee seamless operation without compromising security protocols.
In the realm of cybersecurity, the integration of Data Classification with Cloud Security Measures holds paramount importance. By intricately linking data classification processes with robust security measures in cloud environments, organizations can effectively safeguard sensitive information while streamlining access control. This fusion ensures that data is categorized accurately, enabling the enforcement of stringent security protocols based on the identified data classifications. The synergy between data classification and cloud security measures empowers businesses to not only enhance their data protection strategies but also optimize operational efficiencies through streamlined access control mechanisms.
When exploring the Role of Data Classification in Access Control, a critical component arises in Restricting data access based on classification. This facet plays a pivotal role in governing data accessibility by defining strict parameters for different data classifications. Restriction based on classification ensures that sensitive and confidential data is only accessible to authorized personnel, minimizing security risks and data breaches. The uniqueness of this approach lies in its ability to implement tailored access controls depending on the sensitivity level of data, thus offering a granular and structured approach to data security within cloud environments. While its advantages include heightened security measures, potential disadvantages may encompass the need for precise classification to avoid hindering operational workflows.
On the other hand, Enforcing granular permission settings plays a complementary role in fortifying data security and access control. By setting precise permission levels for different data classifications, organizations can regulate data interactions with a fine-tuned granularity, thereby reducing the risk of unauthorized access. The key characteristic of enforcing granular permission settings lies in providing precise control over who can view, edit, or share data within the cloud environment. This approach is popular as organizations can align permission settings with data sensitivity levels effectively. However, challenges may emerge concerning the management complexity of maintaining intricate permission structures across varied datasets. Despite this, the advantages of enforcing granular permission settings include enhanced control over data access and the implementation of a layered security approach within cloud-based infrastructures.
Future Trends in Cloud Data Classification
In this section, we delve deep into the importance of Future Trends in Cloud Data Classification within the context of cybersecurity. As the digital landscape evolves rapidly, staying abreast of emerging trends is crucial for maintaining effective data security measures. Understanding the trajectories of data classification in cloud environments enables organizations to preemptively adjust their strategies to counter potential threats. By exploring upcoming trends, readers can gain valuable insights into innovative approaches that may revolutionize how data is classified and protected.
AI-driven Data Classification Strategies
Enhancing accuracy through machine learning
Delving further into the realm of AI-driven data classification, we focus on the specific aspect of enhancing accuracy through machine learning. This approach leverages advanced algorithms and computational models to refine data classification processes, minimizing errors and increasing precision. The key characteristic of enhancing accuracy through machine learning lies in its ability to adapt and scale with growing datasets, ensuring consistent and reliable classification outcomes. This method stands as a popular choice for organizations aiming to streamline their data handling protocols effectively. By harnessing the power of machine learning, data classification becomes not just efficient but also adaptable to the evolving complexities of modern data environments.
Predictive analysis for data categorization
Exploring another facet of AI-driven strategies, predictive analysis for data categorization offers a forward-thinking solution to organizing and securing data. This approach anticipates patterns and trends within data sets, enabling proactive decision-making based on predictive insights. The key characteristic of predictive analysis is its capacity to forecast data behaviors, assisting in preemptive data classification and risk management. This method proves beneficial for organizations seeking to stay ahead of potential threats and streamline data categorization processes. While predictive analysis offers significant advantages in enhancing data organization and pre-emptive security measures, it also comes with considerations regarding data accuracy and potential biases that must be carefully navigated within the scope of data classification practices.